#121 Using Dataform and DBT in modern analytics (with Verónica Delgado-Benito at Springer Nature)
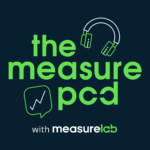
In this episode of The Measure Pod, Dara and Matt are joined by Verónica Delgado-Benito to chat through her journey from molecular biology PhD to data analyst at Springer Nature. They cover everything from her time in venture capital at Project A, to the quirks of working in scientific publishing—and what it’s like to swap lab coats for dashboards. They also dig into a recent project we worked on together using Dataform to streamline reporting across Springer’s complex analytics setup.
Show notes
- More from The Measure Pod
- Sanity over vanity – focusing on metrics that matter (with Ole Bossdorf @ Project A)
- Veronica’s LinkedIn
- Streamlining analytics for Springer Nature with GA4 and Dataform
Share your thoughts and ideas on our Feedback Form.
Follow Measurelab on LinkedIn
Transcript
Build the foundations with something like Dataform or DBT and your tomorrow self will probably thank you for doing so.
Matt
It’s a double-edged sword, because on the other hand, it took me a long time to understand that you need to balance the amount of time you spend doing a task and what you get from it.
Verónica
[00:00:00] Dara: Okay, so a very warm welcome to this week’s guest on The Measure Pod. So Veronica, firstly, welcome to The Measure Pod. Thanks for agreeing to come on and talk to Matthew and I.
[00:00:28] Veronica: Thanks for having me. I’m very excited to be here today with you. I’m a podcast fan, so yeah.
[00:00:33] Dara: Oh, wow. No. So we’re, we’re under pressure in that case, rather than me doing a really bad job of introducing you. I’ll hand it over to you and you can do a much better job of introducing yourself. Just tell our listeners a bit about you. You can go into as much or as little detail as you want, but just a little bit of background and, you know, so you’re, you know, what you do now.
[00:00:51] Dara: But also it would be, it’s always interesting to hear about people’s journeys, to, you know, how they got to where they are today.
[00:00:59] Veronica: Yeah, definitely. So I work now as a prob data analyst, for Springer Nature, which is a scientific publisher. And, before that I’ve been here for almost two years already.
[00:01:15] Veronica: Before that I spent another two years in a venture capital project here in Berlin. I’m based in Berlin, so, I was there for almost two years and also for the podcast funds and for my previous boss who was also in, in the master report. So it’s Olive. He did an episode with you recently and yeah, he’s a great person, great professional.
[00:01:42] Veronica: key person in my career, and I was working with him for two years. and before that I did a bootcamp in data science because I decided to change, career. So my background is. Academically, I did a PhD in molecular biology. That’s how I ended up in Berlin. So I was basically in a lab doing experiments, fully academic in a research institute. And then from there I did the transition into data work.
[00:02:14] Dara: So, okay. So you have to expand on that a little bit, what you just said, you just went from there into the data world. I mean, there’s got to be, there’s got to be a bit of detail there. Highlights ’cause a very, very different line of work. Very different. Yeah. entirely different, I would imagine. So what, what, how did that change come about?
[00:02:35] Veronica: I think of different reasons. So, one of them, I wasn’t really sure after the PhD what I wanted to do. but I knew that whatever I was going to do had to be, I needed to learn more about data because in the lab and in research, I was getting to a point where all my projects were dependent on bioinformatics at the end of the day.
[00:02:58] Veronica: So, and I wanted to control a bit more what I was doing. So I said, okay, whatever I do next, I need some background. and then. It happens that in Germany there’s a great thing, that when you, when you finish working or you are under unemployment, you get an educational voucher, kind of a limit of money.
[00:03:22] Veronica: So I said, okay, after finishing my PhD, I need to use this because. It’s a great opportunity and they financed my bootcamp. So I said, okay, let’s do that. And then I got completely hooked into data and what are the possibilities that were in front of me. So yeah, that’s how the transition basically happened.
[00:03:43] Dara: Wow, amazing. That’s a great scheme they have in Berlin. That’s, I mean, what a great thing to be able to actually retrain yourself after finishing in a, in a position. I’m so there. Yeah. I think we might all move there. Also a great city. So and, and then was it from there into finance or did I miss, did I miss a step?
[00:04:01] Veronica: for the, from there I went to the vc, yes. But, within the VC I worked in, data, in the data team. So I was not working in finance. More in, in data analytics. Yeah.
[00:04:16] Dara: Yeah. so, Have you got any regrets about leaving academia or, I guess I probably know the answer to this, but it’s worth
[00:04:24] Veronica: No, no. I mean, I miss the intellectual stimuli a lot. I think that’s difficult to get somewhere else in, in the same amount and the. I also liked a lot being in the lab up and down and doing manual things. So that’s it. But I, yeah, no, I, I am really happy with my decision and I have so many opportunities in front of me in general, career wise. That makes a lot of sense. So no, I, I think, the only thing I would’ve done differently is maybe do a bootcamping data analysis instead of data science. But at that time I had no idea. So I, and the data analysis bootcamps were not really a thing. It was mostly data science. So, yeah.
[00:05:14] Dara: And do you, do you say that just because of the specifics of the job that you do now, or do you, or do you just mean in general that you didn’t particularly enjoy the data science or, well, not that you didn’t enjoy it, but that you might have preferred a data analysis one.
[00:05:27] Veronica: Yeah, I think what I missed with my academic background, what I missed the most and what has been the most difficult thing to develop over time is the business side of things. So trying to really connect with the business goals, the business strategy, and I think the Data science bootcamp is really techy and really into the, yeah, the like really technical, technically more challenging into the, the math, the algorithms and so on.
[00:06:00] Veronica: Whereas the data analysis part was more into trying to connect it to things like, okay, we do this because it has this reason and this is what I have done most of my career after, post academia. So I think it would have made more sense for me to, yeah, to focus a bit more on that. Definitely.
[00:06:21] Dara: Yeah, it makes sense. I think it’s, you know, being able to tie what you’re doing back to real business value is obviously essential. So yeah, I understand that.
[00:06:30] Veronica: Plus for a person who doesn’t, that doesn’t have a PhD in math or any kind of like hardcore math background, I think I’m years away from a person like that, or that is to the point of studying computer science.
[00:06:45] Veronica: Right. So if you really want to go down the hall of data science from scratch, you need to Yeah. Dedicate your life to it for a while.
[00:06:55] Dara: Yeah. So can, can you tell us a little bit more about what you’re doing in your, in, in your current role? I mean, what are the kind of skills that you need and what’s what, what are the kind of projects that you’re, you’re working on?
[00:07:09] Veronica: Yeah, sure. So, I think in terms of skills, like my job title is product data analyst. So of course I work closer, I work very close to, product managers and, basically, This comes, with the, with the, with the fact that we try to understand how users or, in this case, how our researchers interact with, with us, with Springer Nature, with our websites, with our products, and how can we improve them in a way.
[00:07:45] Veronica: So there’s, I think, clear. Skill needed is definitely stakeholder management. And then what we were talking about, the co the communication and the, the continuous focusing to the business goals and business strategy. That’s key because in terms of analysis, you can do whatever and you start thinking about ideas or talking about ideas, and you can, yeah, do it for hours, but.
[00:08:13] Veronica: Really trying to keep the focus on what is important for the product side and for the higher up, the higher upper management strategy, then that, that’s what keeps going for sure.
[00:08:26] Dara: Do you think, is that something that comes naturally or is that a, is that because you can have the technical skills, and this is something that’s pretty common in the industry, isn’t it?
[00:08:36] Dara: Where there is that disconnect between the people maybe with that very deep technical knowledge and then the. Maybe higher, the, the people with a higher level understanding, sort of like a, a, a shallower understanding of that detail, but that they are the ones maybe ultimately making the final decisions.
[00:08:54] Dara: Do you think that the ability to communicate between those, you know, to bridge those two worlds, is that something that you feel is something that can be developed over time or is it something that’s more natural? in, in, in certain people.
[00:09:09] Veronica: A bit of both is like, LAN or, or you know, I think of bad, like important, like more famous players I think. you need to have or is better, let’s say, if you have kind of a natural talent for it, but at the same time you need to work for, to develop it. Otherwise, it’ll, it’ll not happen. So naturally, you, you may have a personality fit or, or a way of communicating or some background that may help you to, to do this, but you definitely need to work.
[00:09:44] Veronica: And even if you have it and you start a new role. There’s going to be new stakeholders, new strategies. So you always need to kind of develop it and me kind of keep on informing yourself about what are the new things, who you need to talk to and, and so on.
[00:10:02] Dara: Yeah, I agree. And, and that was a slightly tangential, tangential question, but I just find that very interesting and given the role that you’re in, I was very keen to hear your thoughts on that, so, yeah, very interesting.
[00:10:13] Matt: I was just going to. I was going to slightly change direction, slightly into, as I’m prone to do the tech side of things, what has been your experience say since leaving academia? You obviously worked within data science, which is, like you said, very hands-on, very in the, in the weed, in the code, in the, in the mathematics, in the algorithms of it all.
[00:10:33] Matt: When you got into, into the analysis side of things at the, at the, the finance, company. What was your, like, tech stack? Were you straight into for, for, for the listeners who don’t know, obviously we, we’ve worked with Springer Nature quite a lot and, and we’ve recently been working with them on a Dataform project within big queries.
[00:10:54] Matt: I know you know BigQuery from your last couple of years at Springer Nature, but did you? Is that what you were using for your entire analyst career or have you used different tools and things more or less?
[00:11:05] Veronica: So I was familiar with BigQuery before, but when I worked in print a I had the luck and the chance to collaborate with.
[00:11:16] Veronica: Many companies, many companies that had different tools. So I also got to know other reporting tools, other data warehouses, other transformation tools. So I was very lucky on that end too, to get to see a bit of everything. But yeah, I think also in terms of technical skills. Like the way I work with BigQuery and, and in Springer Nature, definitely.
[00:11:41] Veronica: There are also a lot of talented people. The, the, the amount of people doing analytics is very big. So there are a lot of things set up already and I’ve learned a lot over the, over these years for sure. Even though, and doing SQR is not my. Preference. You know, I, I, it is not the thing that I like to do the most, to be honest.
[00:12:05] Matt: What is the thing you like to do the most?
[00:12:07] Veronica: Yeah, more, I would say more talking to problem managers and if, for example, one, to do an experiment, decide if the experiment makes sense. What relatives would we have, how long would the experiment take to run? What are the conclusions we’ll take from it? So all of these more strategic decisions.
[00:12:27] Veronica: And also to have time to interpret the data and say, okay, because of the data, we should do A, B, C, then we can do whatever. But, you know, that does have some guidance based on that. So this data culture part of the role is really interesting for me.
[00:12:45] Matt: And it links back quite nicely to academia in terms of hypothesizing, experimenting. Yeah. Understanding results, I suppose.
[00:12:55] Veronica: Yeah, definitely.
[00:12:57] Dara: I mean that, that, that must give a, I was thinking this earlier. That must just give a great grounding. I, you know, when I asked you did you regret anything about leaving academia and you were pretty clear that you don’t, but you, it must be a big advantage taking that background into industry because.
[00:13:10] Dara: I don’t want to be too disparaging, but sometimes in the head, in the wild, you know, things aren’t done in such a rigorous way. So having that kind of rigorous scientific background must be, maybe it’s a double edged sword. Maybe it’s helpful, but then maybe it’s sometimes as well.
[00:13:26] Veronica: I was thinking exactly that, that, that word like it’s, it’s, or yeah, that’s, it is a double-edged sword because on the other hand, you really like, it took me a long time to.
[00:13:41] Veronica: understand that you need to put into the balance the amount of time you spend doing a task and what you get from it before you actually spend hours into it. In academia, no matter what you need to like, go as deep as you can until you get what you need. And it doesn’t matter if it takes one month, one year, right?
[00:14:01] Veronica: The importance is to go to the end. And, it took me some time to say, okay, you know, this task actually is going to take three months, which we don’t have. And also, I may spend my time on something else that is going to bring more value. So all of these, yeah, it’s a double world. Definitely.
[00:14:23] Dara: So one of the, one of the things we did want to talk to you, in, in a bit of detail about, Matthew mentioned this briefly earlier, is a recent project that we worked together on, and obviously appreciate.
[00:14:36] Dara: We can only go into it to a certain degree. level. but it’d be nice to get a little bit of an understanding if we can, on, the kind of background of that project and, and, and maybe some of the e even if we focused it on some of your personal outcomes. You know, what, what, what did you learn through that work?
[00:14:53] Dara: so, so maybe Veronica you could just start with giving us just a little bit of a background or, or an overview even of what the. The project was this Dataform project that we’ve worked with you on?
[00:15:02] Veronica: Yeah, so, basically we wanted to leverage our data infrastructure, tools. I had worked in the past. I had worked in the past with DBT, which is also great. And, I know that having a tool allows for it. Data transformation with some specific characteristics like TBT or like Dataformula is really, really, a plus. And, we wanted to do that in, in our team and with our data. So yeah, that’s why we, we work with, with you and, to do this at the same time that you have your own task, you.
[00:15:45] Veronica: Yeah, we are. You need to, you need some help. So, yeah, that’s why we also collaborated with you and, we, yeah, it also makes sense to do it with people that have done it multiple times for different companies. So to have a clear idea of the setup.
[00:16:02] Dara: And what went into the, so sorry, maybe I’m jumping ahead. What went into the decision as to whether you went with DBT or with Dataform or, or with some, something else entirely.
[00:16:15] Veronica: So our internal, I would say internal discussions and also I remember we had this discussion as well with, with Matthew on the team. Depending on the, like, the rest of our setup as well, what makes more sense in terms of cost and, and in terms of, yeah, what we had already. So both.
[00:16:38] Matt: Yeah, it, I think primarily it, it, it was, it came down to the type of data we, we, we were working with as well. Like, it was all data that was already located in BigQuery and it was primarily like Google analytics type data. So with something like DBT and I suppose it might just be worth just to take a quick aside here just to explain DBT and Dataform for any listeners that haven’t come across it, but they’re, They’re essentially really pulling apart and modularizing sql, code and, and creating sort of models and transformations for data that’s very repeatable, pulled apart, testable version controlled, backed up in, backed up in services like GitLab and GitHub. It just really brings software engineering best practices that have been honed over decades to data engineering, which has been lagging behind a little bit on, on that front.
[00:17:34] Matt: So that’s what those tools are. DBT being the, the original and then Dataform came out of a spinoff. Somebody who left Google years ago started, started data from Google, came in, bought the company back off, merged it into, into. Google and then eventually BigQuery. And then Dataform is just getting more and more closely melded into BigQuery as a lot of things Ed in a theme of the past, sort of three weeks of this podcast.
[00:18:05] Matt: So it made sense. Given the data, given the, the, the models that we wanted to be built and all of the stuff and, that, that we discussed, that deform worked because it was free. It’s part of the existing services and we didn’t have to host it or do all the other things we would have to have done with DBT at the time.
[00:18:24] Veronica: Something that I really liked about DVT, sorry, Dataforms from the beginning. I mean, DVT will do the same, but we have now, we had before as well, but now, I really like that Dataform provides a clear, ERD so, ERD, so this is the entity relationship diagram where you can see the relationship in between all your tables and all your queries and, when you have Dataform or DBT, this is much more controlled.
[00:18:58] Veronica: And, I feel that the ownership of the queries tables is also more clear and you can reuse, you can define certain things and reuse them. whereas, if you don’t have a transformation set up like that, you just need to create queries all the time or like to reuse the query that somebody else wrote and so on, which is not the best.
[00:19:27] Matt: Yeah, I, I suppose one, one way, ’cause one of the nice things you say, like with that, with that graphical interface where you can sort of see the, what do we call it? Compilation graph, I think, I believe is the term in, in Dataform. But it’s automatically handling the dependencies between each of the nodes of that, of that graph as well.
[00:19:44] Matt: Whereas perhaps with a more traditional way of doing it within a warehouse where you’re using stacks of SQL queries and dealing with the dependency between the different. SQL queries can become troublesome and it can be about timing and about this, that and the other, which can get a bit tricky to handle, especially if it gets very large and you’ve got lots of different tables and lots of different reporting outputs that you’ve got to deal with.
[00:20:07] Matt: So it is a really nice way of just viewing things and, and ensuring that everything is waiting on what it needs to wait on and delivering what it needs to deliver.
[00:20:17] Veronica: Yeah, exactly. And one of the key points I think of the success of this project is that we. So from the very beginning together, how do we want to structure it?
[00:20:27] Veronica: Because at the beginning it’s a blank canvas, and you can do everything. But, having an idea of what type of, let’s say levels or, different tables you want to have and why they are like this, I think that makes a lot of sense. and it helps, the difficulty of all of this is making it scalable because of course you can have a picture of the tables you want today, but you need to think of how your setup is going to look tomorrow, look like tomorrow, so you can scale it. And that, that’s, I think, the difficult analytic, the key part of this.
[00:21:05] Dara: And, and on that note, what’s the, what does maintain ’cause I, I, and you know, tr treat me and maybe some of our listeners, like, they know absolutely nothing or virtually nothing about this, but what, what would be the, what’s involved in kind of maintaining and scaling it, like you said, so let’s say the, the, the, you know, the needs, the number of tables grows or the, the, the, the size of the data, whatever.
[00:21:26] Dara: You need to scale this, you need to change it. Is is it quite an involved process maintaining this once it’s all set up, or is that just in the hands of a small number of people who can make a small number of changes to make sure it’s, you know, what kind of like what’s involved in that, in that ongoing maintenance and, and scaling. The solution.
[00:21:44] Veronica: I would say, depending on how you set it up, that’s why this is an important decision from the beginning. But ideally it would rely on a small number of people or the people that work with it, ideally. But it really all depends on how you set it up. And. One of the key aspects here, which I think it really relates well to, the beginning of our conversation, is the knowledge of the product.
[00:22:10] Veronica: In this case, because we are products, we are talking about the product side, right? If we would be talking about the marketing side, then the marketing knowledge, right? But that you have a clear understanding of the problem and some ideas of how it may evolve. And this requires conversations with the pro managers, with, with a strategy to see, okay, this is how we may grow in this direction or in this other direction.
[00:22:35] Veronica: So hence our data will probably look like this. And then it makes sense to have this structure. You may be wrong, but it makes sense to have it like this.
[00:22:49] Matt: And I, I think I. And Todd, you agree with this, Veronica, but there’s almost a bit of a, there’s a bit of a paradox in terms of, of the complexity of things because when you, when you first come across something like Dataform or DBT, especially as an established analyst, it’s very foreign and it’s like, it’s a new way of working, pulling apart SQL queries into these modular forms and having, like having repeatable pieces of it tucked away over here to be used in multiple places.
[00:23:15] Matt: It is a bit odd at first, it’s a bit disorientating. It can feel like the simpler of the two routes is to go down the scheduled query path because it’s familiar and you know how to do it and you can just write it all in one thing. So it feels less complex. But I think in reality it’s actually more complicated to have stacks of scheduled queries because for one, most of that knowledge is locked inside the individual’s head.
[00:23:42] Matt: And it’s very difficult to sort of explain that to somebody else. and for two, like you, you can have all of these, this documentation and, and unit testing and assertions, assertions being sort of automatic testing that occurs and version controls and all of these different layers. That, and the modularized nature of it that I think makes it easier to maintain.
[00:24:05] Matt: Even if, when you first get into it as an analyst, you might think. This feels a bit more complicated than I’m, than I’m used to. I don’t know. I don’t know if that reflects your experience.
[00:24:15] Veronica: Yeah, I mean I think that everything that is new takes time. It takes time and, and thanks to you actually and everything that, because we did as well, quite extensive Hanover process and educational process. And this is also important to say we did this and it makes a lot of sense. It’s not that we collaborated and then we were alone with the, with the tables or with the, with the infrastructure. No, it’s a process of why is being set up, understanding why things are being done, and then hung, hungover.
[00:24:51] Veronica: So these. This makes sense. And of course, as everything new as I, as I was saying, as I was saying, and when everything new comes in, it takes time, but it makes a lot of sense already from the. From the perspective and maybe from the people that didn’t use it. With Dataform, you can see the query that is populating the table.
[00:25:15] Veronica: But you can also see a line that goes back to the previous table that is connected to it. And you can also see the query of that. So you have a real clear visibility of how things are put together, whereas in. If you have scheduled queries first, you need to have permission to see these scheduled query queries.
[00:25:35] Veronica: You need to know how to set them up. you need to understand how the tables are connected. So yeah, it also does not create or remove the dependency on other people to keep on working.
[00:25:48] Dara: So once you get, once you get your head around initially, just the, the, the fact that it’s something new, it sounds like from what you’re saying, that actually you end up in a place where it’s a lot clearer.
[00:25:57] Dara: You can see what’s connected to what you can, you know, as opposed to maybe the old wave. Maybe I shouldn’t say the old way. ’cause maybe this is still a suitable way for certain people depending on their needs. But you know, the way you were, it depends on the complex.
[00:26:09] Veronica: Yes, it depends on the complexity.
[00:26:12] Matt: If you’re going to, if you’re going to, you’re not going to, you’re not going to create a Dataform repo to potentially make, have a reporting table that.
[00:26:21] Matt: It’s probably overkill. But when you’ve got a more complicated sort of state of, of raw data then and a lot of different reporting. Tables and potentially a lot of different stakeholders, it definitely makes sense. I think
[00:26:34] Dara: I was going to ask you about that actually. I mean, you’ve kind of answered it there, but like, I guess like with a lot of technology or a lot of solutions, whatever, there’s, there’s a, there’s a tipping point and it’s maybe sometimes not that clear where that is.
[00:26:46] Dara: I, I’m trying to get my head, my own head around at what point somebody would need to use something like. Dataform or, or DBT, because there’ll be a point where it’s too small or too simple. And there’s a point then where it’s definitely big enough and complicated enough. But do either of you have any guidance for maybe anyone out there who’s listening, thinking, Ooh, I’m not sure I’m somewhere in the middle. Do I need this? Do I not need this tough question?
[00:27:10] Veronica: Massive spread. I can say what I think of you most to each other. I can say something, but, that is the, I think that, apart from the complexity. It’s about as well, the amount of data transformations you want to make. Because if you just want your data to do small calculations and to do small transformations, then you probably don’t need the Dataform.
[00:27:38] Veronica: So at, at the, at the core of it is used to transform data. Right. And to, and to, yeah. Create new tables and so on. So that, that also makes sense and depends as well on how. Many data sets you’re working with together at the same time.
[00:27:58] Matt: I think that’s an important, I think that the, how many, if you’re trying to join multiple data sets together, say, say if we, if we switch to like a marketing analytics mindset for a moment, you’ve got Google ads coming in, Facebook ads, GA four, couple of other different sources.
[00:28:14] Matt: You want to pull that data together and try to sort of join it and mull, model it. Just do Dataform, start there, start thinking there. because it’s just going to make your life a lot easier in the long run. Even in the short term, it’s going to be a bit more complex to get your head around because again, this is part and parcel of the BigQuery.
[00:28:32] Matt: It’s not going to cost you any more money. It’s just going to give you a more robust foundation to sort of build on top of. and I think a little bit of, of what you said earlier, Veronica, where you’re saying, you know, plan out. What you’re wanting to do and, and, and how this is going to look for scale scaling down the line.
[00:28:50] Matt: it could be that you are, you, you know, you, you’ve got a real concrete plan and know what you’re going to do, like, right? We’re going to get this data in, then we’re going to get this data source in and this data source in. If you know and have a good plan in mind, then why not begin to do it with Dataform?
[00:29:03] Matt: Because you can, you can scale it and pull in different resources and build on top of the modeling you’re building rather than going off for six months. A lot of scheduled queries and they go, right, okay, we need to tear all that down now and build out this, ’cause we’ve got this data source in. So I, I think, yeah, simple stuff where you’re just sort of accessing raw or near raw data, you’re probably fine to use just big que and they’ve, they’ve now added in repositories in big queries, so you can even back up your little standalone SQL queries.
[00:29:34] Matt: When you start to join a couple of datasets together or start to have a bit of a longer term plan, build the foundations with something like Dataform or DBT and your Tomorrow Self will probably thank you for doing so.
[00:29:46] Veronica: Yeah. And of course it’s also connected to GitHub, which is, or you can connect it to it, GitHub, which is great because you can have version control, you can have others reviewing your code and all of these always, yeah, makes it much better.
[00:30:05] Matt: I, I was just going to say, how have you found them? So say your day-to-day, and I know you’ve used this before and, and, and things like that, but let’s not say since the project we work together, but comparing a day-to-day of say, troubleshooting or creating a new request or dealing with a new request in an existing world where it’s just schedule queries and, and sort of stacked queries in that way, versus doing that with something like Dataform or DBT.
[00:30:36] Matt: What are. Can you feel and see a difference there of like, it’s simpler because it’s, it’s of the way it’s structured and things or, yeah.
[00:30:45] Veronica: I think it’s also a lot more transparent. Like, let’s say, my colleague is off today or seek, then I can really see the stuff or I can review the query, I can see what has changed, how I, how was before, whereas is.
[00:31:03] Veronica: You have just scheduled queries. Either you have a very good documentation in place, with everything, or you need to continue. You depend on others. You depend on the person that did it. And I think here, like with DBT or with Dataform, that dependency is smaller. It is still there, but much smaller. Definitely.
[00:31:23] Matt: Yeah. And, and I suppose as well, I mean. You know, hopefully good colleagues don’t leave, but they do. And people move on and go to different businesses and, and the dependency you have on an individual as they leave the company when they’ve got this complex data infrastructure they’ve constructed, and a lot of it exists inside their head.
[00:31:43] Matt: And with the best one in the world, manual documentation is prone to gaps, and dying on the vine a little bit. the automated nature of some of this stuff just makes it. A no brainer and easier to onboard new people should you have to.
[00:32:00] Veronica: Yeah. Maybe something as well that we should say is that. Or that I think it’s a real advantage of these tools is that you can connect to GitHub and you can have different environments. So you can have, you can test things without necessarily making changes in, in the lives, version. So this is super helpful, because you have an idea or you want to test different ways of putting something together.
[00:32:27] Veronica: And you don’t necessarily need to tell all, all your colleagues, okay, I’m going to change this. You can do it just yourself on your local machine and then see how it looks. So that’s also great.
[00:32:39] Matt: Yeah, that’s, that’s like one of the biggest things that I think comes from that software engineering best practice rule book of, you can almost think of say all the dashboards you’re building for, for product lead, product owners as.
[00:32:53] Matt: Production data products, and you can be experimenting and building and doing what you want with the underlying SQL in your branch, in your, in your Dataform repo without ever worrying that you’re going to accidentally break something that they are reliant on, on us from a business perspective. That, that you can just tinker to your heart’s content until you’re happy and then push that up to the production environment, which is, which again is something that’s not really been a mainstay of, of. Data engineering and, and analysis for a, for a very long time.
[00:33:23] Dara: So, Veronica, you mentioned, I think I’m right in saying you mentioned at the, as part of your intro that you had worked with DBT before. Am I right about that?
[00:33:34] Veronica: Yes, but not as much as with the Dataform.
[00:33:38] Dara: Okay. I was just going to ask you, I mean you can probably, you can probably still answer this, but what, what, what’s your. What’s your kind of view on, like what if you were to compare them or, or even like, what are some of the differences? I guess they do similar things, but you know, are there any notable differences that you’ve identified having worked with both of them?
[00:33:56] Veronica: I think they are structured in a slightly different way and they, yeah, the methodology of how you set up.
[00:34:09] Veronica: Like definitions or, or stance and so on. It’s a bit different, but it’s, they, they, they’re very similar. Like I, I think it’s more a preference than, than, I don’t know, Matthew, correct me if I’m wrong, but, but for what I have seen, it’s more a matter of preference and really. Advantages of one versus the other.
[00:34:32] Veronica: But I’ve not used DBT to understand that I have used Dataform, so I’m also not objective in my comparison. I think that visually it’s also different. So it’s just a bit ma mainly on how you, how you like it. I like DBTA a lot, but Yeah. You know like recently is more like, yeah, they did a great job.
[00:34:55] Matt: Yeah. I think so too. So d the, the, the sort of headline differences are, DBT uses HY for its sort of repeatable code pieces and, and sort of so forth within, within date FORMM and DBT. You can write actual code functions to sort of loop through things and create little distinct functions of transformations of stuff in, say, JavaScript and Python rather than sql.
[00:35:22] Matt: and DB T uses Python Dataform using JavaScript. DB as Dataforms got absorbed by Google, they no longer offer a cloud version that you can deploy anywhere. I don’t believe so. DBT obviously used to be a standalone thing that you could host. sorry. Dataforms used to be a standalone thing.
[00:35:38] Matt: You could host wherever you wanted, but now it’s very much integrated into BigQuery, where DBT, you can still host a version of it wherever you want on your own servers. You could host a version of DBT in Google Cloud, if you so wished, but obviously there’s associated costs with that hosting and things.
[00:35:56] Matt: I think DBT is also a prob, well, it is a more mature product because it’s been around for a lot longer, but I think Google has done a very good job of really integrating a Dataform into BigQuery. So if, if most of your data is sitting inside a BigQuery and that’s going to be your warehouse, it does feel a little bit like a no brainer to me, but I’m sure that people will tell me otherwise, but.
[00:36:21] Veronica: Yeah, exactly. I think it really depends also what, where your data is stored and located. That’s, definitely, a key point for deciding between one and the other.
[00:36:33] Dara: We’ve gone this far and nobody’s mentioned ai, so I’m going to be the, I’m going to be the, I’m going to, I’m going to be the one to, I’m going to be the one to throw it out there.
[00:36:42] Dara: but like initially, at least to do with Dataform. This again, could be to either of you. Everything in BigQuery now, everything in Google Cloud has got AI assistance. Is, is, does Dataform, is this going to, is it the need? What’s my, I’m going to try not to ask too warmly a question, but like, is the need to use something like Dataform, is that inevitably going to disappear?
[00:37:04] Dara: Will you just have something, you know, a conversational interface where you’ll say what you want to do with your, with your data and it’ll, it’ll go away and it’ll. Do the, do the Dataform bit for you, and you don’t, you won’t even need to have the skills to do that. Is that the way it’s going to go, do you think?
[00:37:22] Matt: I think Dataform is, I think this is a good example of what Dataform is and why it’s different to other things on, say, BigQuery, and the AI stuff, they’re, they’re putting out there because a lot of the a ai features they’re adding in seem very standalone, so like. A data preparation where you, you get a data setting, you go through a set of transformations and output a table or a, like the canvas assistant where you’re exploring a, a piece of data and trying to get to a conclusion or the data engineering, assistant that will take you through trying to get pipeline of data out and it transformed in some way.
[00:38:00] Matt: Dataform to me is big. A much larger picture. You’re, you’re taking your, a large number of different data sets and combining and modeling and putting them all together in very repeatable fashion to service, or, service activations in whatever form they take, be it dashboard, be it ml, be it whatever.
[00:38:22] Matt: and so far there’s not been any rumblings yet of Google. Having an assistant sitting inside a Dataform or anything like that. Perhaps because of that, because you need to have that larger picture view like Veronica talked about a little bit earlier, where you, you need to think about a really wide estate and how you’re going to transform and build and scale that.
[00:38:42] Matt: I’m sure there will be assistance to start. I have no doubt about it, but currently there isn’t anything there. Even if it’s useless, they’ll just put it in there anyway. Yeah.
[00:38:51] Matt: But it’s, as I said, the salt tape. The salt tape Gemini onto anything, Lauren. But, but maybe my, so sorry, Veronica, just before you give your take on that, I just going to ’cause to, to, to me, to my uninformed brain.
[00:39:03] Matt: Everything you just said there, Matthew sounded like a, it actually made it a prime candidate for AI fy it’s like, it’s repeatable stuff. It’s, it’s pulling in, you know, across multiple, multiple systems or data sources. So isn’t that exactly the kind of thing that AI can help with? What, what am I misunderstanding?
[00:39:22] Matt: Maybe everything I.
[00:39:23] Matt: I think it can, yeah, I, I, I, I think it can, can, and could I suppose that, that what it lacks currently is that big picture view of things and the, the stuff that Google, gestured towards, shall we say next, that it is going to start to have a larger picture of your entire warehouse and it’s going to have access to this data over there and this data over there, and it’s going to be able to sort of understand your specific enterprise world a little bit better.
[00:39:51] Matt: At that point, it can maybe start to help you model wider data sets and, and, and create transformations. But I think the problem at the minute is any of those transformations, it does, will be very much in a use by use case, by use case basis rather than linking all those use cases to be together into a larger model if any of that makes sense, that might have just been a brambly bit of nonsense.
[00:40:13] Veronica: But yeah, no, I have no doubts that it will happen and it’ll be there. But you just said something that for me is key in this, which is about giving access to the data sets and giving access to your stuff. So. I think that, of course, everything about AI is really hot right now and, and everyone wants to do it and develop tools that are AI based.
[00:40:43] Veronica: But the situation is Jumanji at the moment, meaning that, I think there will be a point where the, the privacy, aspect of it is going to come into the or, or it already is, but I think it’s going to come into the, into the picture more and more. And, I’m not sure how many companies would be willing to give AI, Google, or whatever enterprise access to everything in order to do the job.
[00:41:14] Matt: yeah, you mentioned, I mean, that’s one of the things that came up in next where we’ve got this, can we get the term right this, ’cause we, we, we buggered up a term last time, but the, the idea of the. The idea of, of sort of taking Google Cloud off the cloud and having your own localized version of it for really large enterprises that are very sensitive to, to, to regulation or to whatever set of the banking sector or whatever it may be, and it being. We said airlocks last time, which definitely wasn’t right.
[00:41:49] Dara: Yeah, I’m trying to Google it. I know, I know what you mean. That all I can think of is the wrong way to say it. Air gap. Air gap. Air gap. Air gap.
[00:41:55] Matt: That’s the one. What’s mine, yeah. Have an air gap to, to give the, to give the company the, the feeling and trust that they own the data and that it’s, it’s still with them and they’re just utilizing tools developed by Google rather than it going off and living in Google’s ecosystem and on Google’s cloud.
[00:42:13] Matt: I think people are still going to be sensitive to that. So that’s it. Definitely a problem. Google has to figure out how to solve or to at least convince people they’ve solved it.
[00:42:22] Dara: So, so what about in your, so you, you are right in saying, you said a few minutes ago, Veronica, that you said that, you know, like AI is obviously the hot topic at the moment.
[00:42:29] Dara: what, in, in your, kind of, in your current role, are you, are you feeling the pressure to, to do more with ai? Or are you finding, ’cause I, and I guess a little background to my question is. From our side of the fence. As a consultancy, we’re obviously having to keep up with all of this stuff. And what we sometimes forget or don’t appreciate is that on the other side of the fence in-house it might be very different and in some cases businesses might just be like, look, we’ve got priorities.
[00:42:57] Dara: And at the moment this just isn’t one of them. We’ll get to it. So what, how are you finding it? You know, an in-house role, how are you finding all the buzz around it? Are you feeling that’s filtering down to pressure on you or is it actually just noise that you can, you can just filter out?
[00:43:13] Veronica: I think that, AI internally, or, or from my point of view, it’s, it’s a topic and it would, everybody’s looking at it and every company looks at it.
[00:43:25] Veronica: So it is definitely a hot topic and it’s something that everybody does. I mean, like with everything that is new, you have two options. Either you close your eyes or you, you, you accept the situation. So now, I mean, we need to accept this is what it is and every, I think everybody is into this phase and see what can we do with it.
[00:43:47] Veronica: But from my point of view, at least, I think the smart thing to do is, Or, or something like, technically AI will do everything. I, I have no real, I, I have no intention of being a pro now in any language, programming language, or doing anything because AI can already do it for me and will do it for me.
[00:44:11] Veronica: What I think it’ll still take time is having this thorough understanding of the business and, and deep understanding of the product. Or so on. So for example, in my case, because I did the PhD and I worked in academia, I know how research publishing works and, and how everything is, is, set up from the beginning to the end.
[00:44:36] Veronica: And what I have in mind, what our users are like, because I have been one of these users and these. AI will never be able to give it to me. Yeah. Can give me a summary or can give me some ideas, but this is the advantage I would say, or like this should be the focus, let AI do what AI can do, which is a lot of things, but yeah. But yeah, definitely companies are all into that.
[00:45:02] Dara: So it sounds like you’re not worried about it. ’cause I know some people are thinking, oh, it’s going to come along and take my, take my job. it sounds like you are in the other camp of people thinking, no, let it do the, let it do the repetitive work. Let it, let it take that off my hands and let me use the stuff that only, only I can do.
[00:45:17] Veronica: Yeah, exactly. And also, I mean, and there’s no way we can, even if you don’t like ai, it’s, it’s here already, right? P it life with everything in life. You need to accept that. The reality is it is what it is. So once you take this, spend less time. Complaining or lying, trying not to accept something that is already happening.
[00:45:41] Veronica: Then use the time for something else and I, of course it’s going to replace some, probably some jobs, but more than replace, I think it will replace certain tasks and certain skills, and the jobs will evolve. Definitely like, I mean, if you compare now what our parents did, did, or like to do jobs now and in the sixties or the fifties, of course they are different, even if they have the same name. So I think it will just evolve.
[00:46:11] Dara: I can even go narrower than that. If I think about what we did at Measure Lab at the start versus what we’re doing now, it’s, it’s, it’s very different. And that’s only 12, 13 years. So, you’re right. You know, these things keep changing and, you can’t, you can’t ignore them.
[00:46:25] Dara: You know, AI is here. Technology is changing. We just have to adapt to it, only, and I think, what you were saying about it not replacing jobs, something Matthew says a lot around it. Augmenting, you know, it’ll augment you and you can, you can use it as an age to make your job easier, make you more efficient.
[00:46:44] Veronica: Exactly like we have Google Maps that at the time we didn’t have for, I don’t know if you, like, I was recently planning a trip and I asked at DPT, why not? I mean either me spending time there and then I give me some answers and I might it, it may help. And so I just think that closing the eyes is not that good strategy because it’s already here and we are still here working. Right. We are not. Anyways. Exactly, exactly.
[00:47:14] Dara: I just had one more question for you. I don’t know, then obviously maybe Matthew’s got more or maybe there’s other stuff you wanted to bring up yourself. but just one other question I wanted to ask you. So you, you mentioned, and I agree with you, that you know, you don’t really need to go and I.
[00:47:28] Dara: Be an expert in any particular coding language now. So what are you doing, what are you spending that extra time on? Are you learning anything else now instead of, and I, and I don’t just necessarily mean with your, like specifically to your role now, but what do you, you know, what are you spending learning time on now that you might’ve previously spent on, something like coding languages that you can now use AI to help you with?
[00:47:52] Veronica: Yeah, I like, I personally like a lot storytelling, so I try to get informed or like get educated about that because at the end of the day, a lot of things are how you tell them or it’s about how you tell them and not exactly what you’re saying. So I like to get educated into that. Also, I like the part or the fact of making.
[00:48:22] Veronica: decisions, more data driven. This is like, also like a super word now, this data driven everywhere. Okay, so apart, but apart from the super word or the, or that it, like AI that is hot. I think it makes sense to, to understand what does it really mean to be data driven and what are the limitations on the possibilities of it within a specific context.
[00:48:47] Veronica: So I like those topics to be honest. But yeah, I also spend time coding, but yeah.
[00:48:56] Dara: Okay. I think that draws us to quite a natural conclusion. I’ve really enjoyed this chat, so thank you again for agreeing to come on, and talk to us and it’s really, really great to hear that you’re a fan of the show as well.
[00:49:08] Dara: It’s nice to have somebody who has listened to episodes then come on actually. See what it’s like sitting in the hot seat and being a guest. So, it’s been a really, really interesting conversation for me. I’ve really enjoyed having you on the podcast and, and, and talking to you about your, your role and your interests and, and your, obviously your views on AI as well.
[00:49:27] Veronica: Thank you so much. And also Matthew and the team for working with us. It has been a great experience. And yeah, also to chat to you today. And for anybody out there that wants to do the transition or any transition, don’t be scared. If I can do it, everybody can do it. And definitely yes, yes. And it’s fun.
[00:49:47] Dara: So yeah, I’m always happy to chat. That’s it for this week’s episode of the Measure Pods. We hope you enjoyed it and picked up something useful along the way. If you haven’t already, make sure to subscribe on whatever platform you’re listening on so you don’t miss future episodes.
[00:50:01] Matt: And if you’re enjoying the show, we’d really appreciate it if you left us a quick review. It really helps more people discover the pod and keeps us motivated to bring back more. So thanks for listening, and we’ll catch you next time.
Subscribe to our newsletter:
Further reading
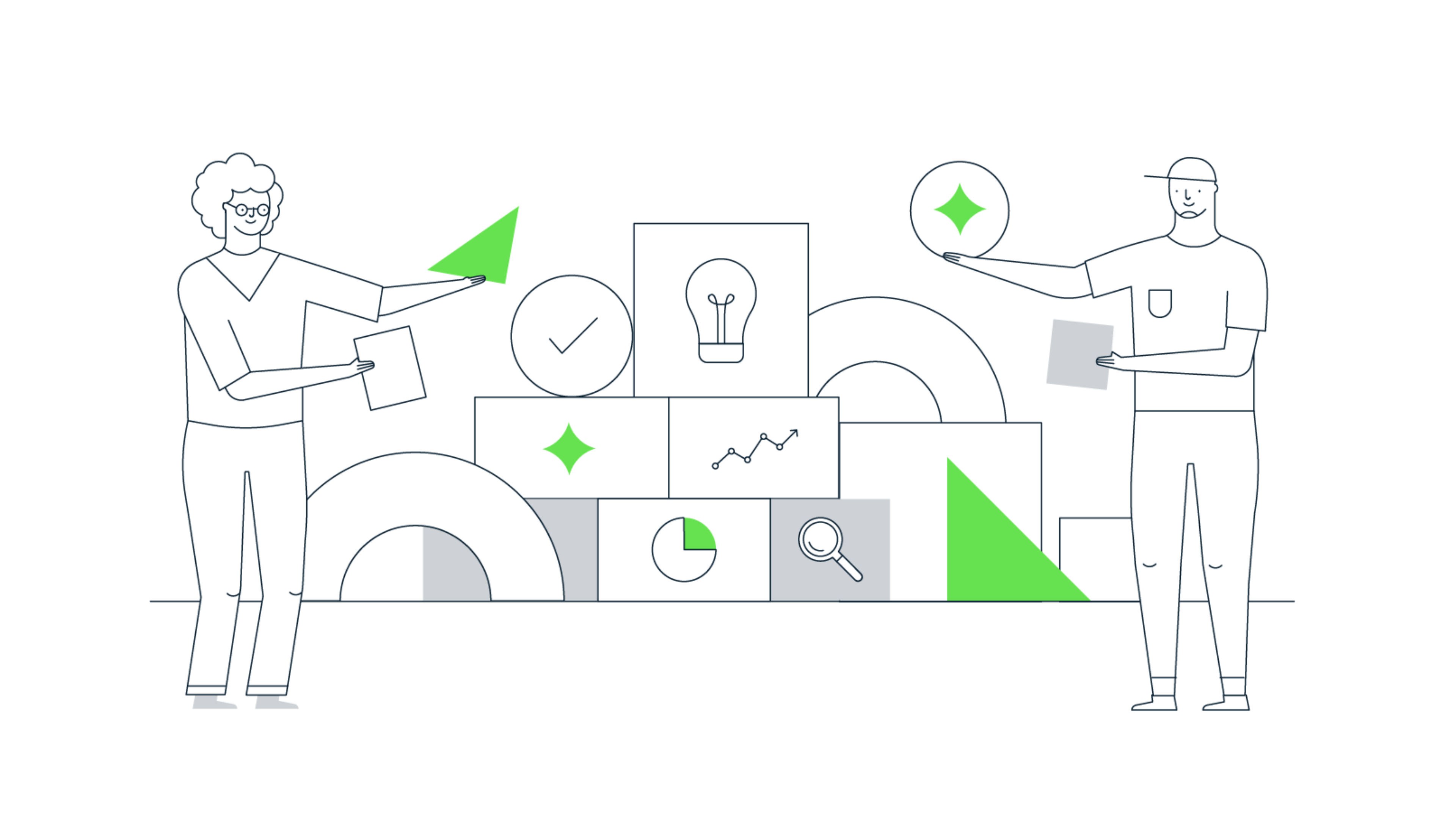
Easy ways to prepare your BigQuery warehouse for AI
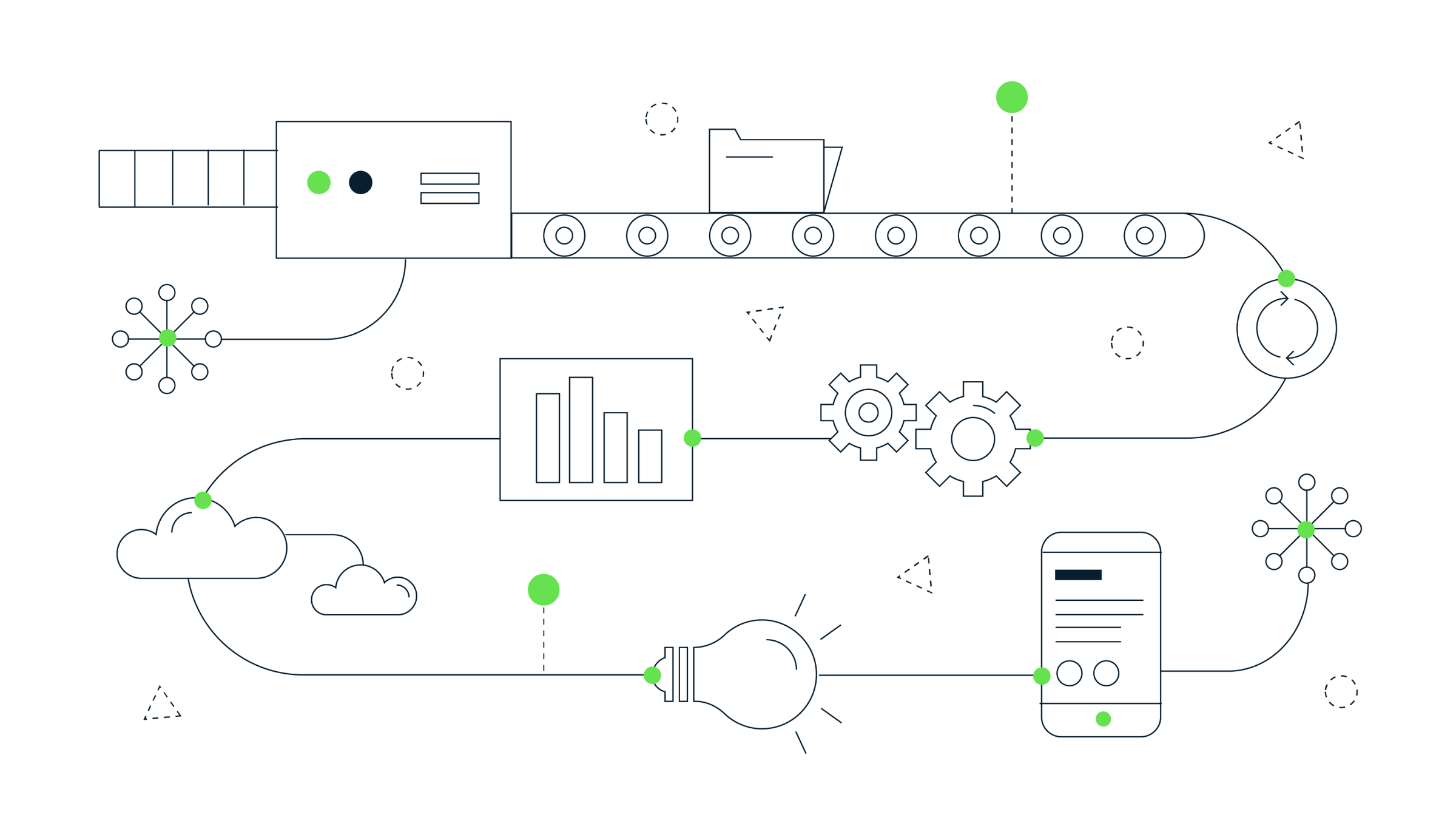
Data pipeline optimisation with Google Cloud and Dataform
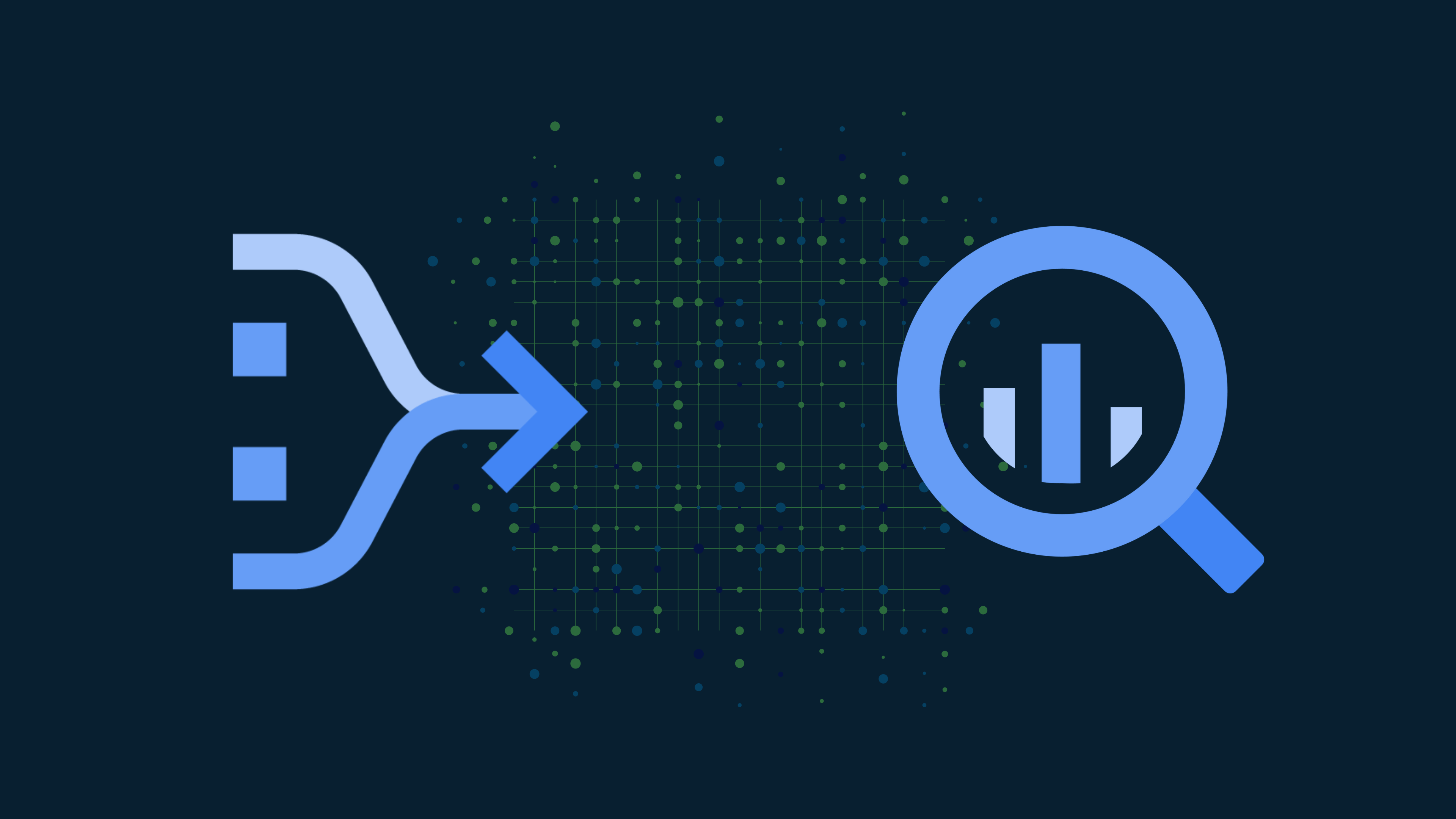