From the wild west of data collection to conversion modelling
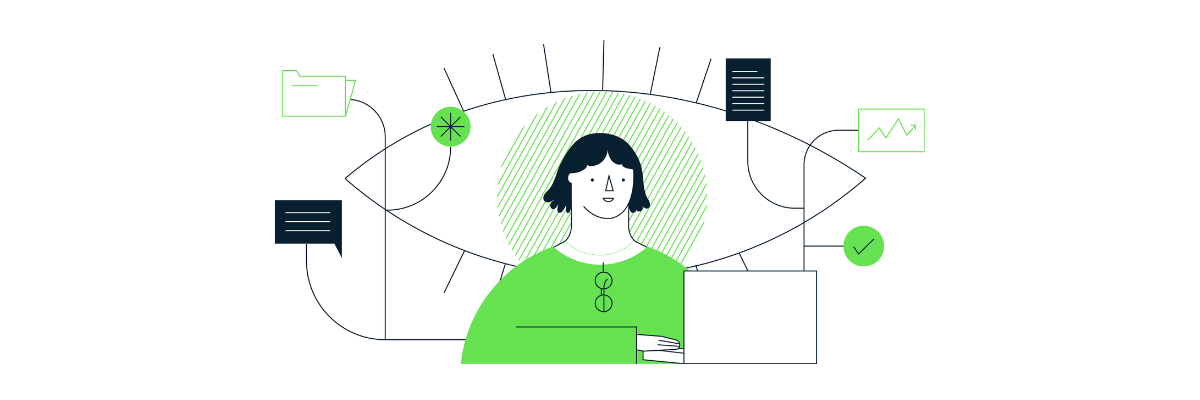
Access to ‘real’ data in Google Analytics (UA and GA4) is becoming more limited due to technology and legislation changes. So, what does this mean for people who are used to having access to all of their data, and how will this change how we use data in these platforms in the future? Is this the end of real data in analytics?
Google announced in 2021 that they are moving all of their products over to machine learning models, whether that’s data-driven attribution or filling in gaps with conversion modelling. Analytics consultants like us are used to having access to 100% of the trackable data to export to BigQuery from Google Analytics or creating audiences and segments based on the smallest segments of users when they go through a website in a very specific way, but this won’t really be possible going forward.
Increasing regulations make measurement challenging
Like it or not, this change is going ahead, partly in response to the fact that observed data is becoming less and less reliable. There are an increasing number of gaps in data collection due to improvements in data privacy legislation and technology within browsers to control the information that’s being collected about you as a user. There’s actually less data collected in tools like Google Analytics now than there was 5–10 years ago.
It’s no longer the ‘wild west’ in the digital marketing and analytics world – technology and legislation are catching up. Every browser has specific rules around how they manage cookies and trackers, and most browsers, except for Chrome, already block third-party cookies. Chrome will remove access to third-party cookies some time in 2023 and that will officially be the end of third-party cookies.
Although first-party cookies aren’t going anywhere, with the browser technology available, setting these can be quite limiting. Safari is limited to either 24 hours or seven days, depending on certain factors. In other browsers, you don’t set any analytics cookies at all – there’s no tracking whatsoever. So there are issues that didn’t exist ‘back in the day’.
Adapting to a world that prioritises privacy
Legislation like GDPR and the CCPA (soon to be the CPRA) are changing conditions all the time, requiring active opt-in consent (to be acknowledged by the user that you’re taking their data and doing something with it). The actual data you end up collecting in a platform like Google Analytics, regardless of what version you’re using, is reduced. There will also be fewer users – not everyone will opt-in to be tracked.
When they do opt-in, the data won’t necessarily be as accurate as it was in the past because the cookie won’t be there to tie it all together. If a user returns once a month, they’ll most likely be seen as a new user, regardless of what browser they’re using. The fact is, web analytics data has never been entirely accurate – there have always been reasons why data will be missed.
Even when there’s a 10% difference, it’s still useful for trend analysis and comparative change. Rather than thinking of it as the total revenue you’ve earned, it’s a change compared to a previous period. Cross-device users and cross-browser tracking has also always been an issue even when cookies were more prevalent, so some of the modelling techniques may actually improve the data’s accuracy.
We’ll never really know though because it’s a black box algorithm and we’re taking Google Analytics’ word that they’re modelling users and stitching users together, understanding behaviour from unconsented users, and applying it to the real data. We assume they have our best interests at heart and that it’s going to be 100% accurate, but it’s not transparent. The black box modelling doesn’t allow you to dig into and understand how it’s working.
The changing digital marketing landscape
With observed data less available, this is something that has to happen. Modelling is the best option available based on the constraints, both technical and in terms of the legislation. And Google Analytics is clear: if you want to use our tool, you sign up to our terms and conditions, otherwise, there are other ways (and other tools) to collect and process data.
They’ve sweetened the deal with an easy-to-install product that has a free version that anyone can use and most people are familiar with. Most people don’t have an analytics team, data engineers, or data scientists to set up their own analytics tool and send data directly to a data warehouse though, so they’re pushed into a corner and forced to adapt.
Google Ads has been modelling data for some time, specifically around conversion modelling where there’s no cookie data available. Modelling is used with the store visit data within Ads as well. Even Universal Analytics has been using modelled data for a number of years, and the sales pitch of GA4 is that it’s built on machine learning, using built-in predictive audiences and predictive modelling.
Conversion modelling
GA4 introduced conversion modelling in July 2021, which identifies viewable users that convert via everything that’s not direct traffic. It takes all of your users that converted directly, so no campaign data was available, and then it tries to model the actual campaign that drove that conversion. So, it’s not creating conversions where there were none. They try to model, based on viewable data, what the channel was that drove users to convert.
These are the use cases or scenarios where modelled conversions might be applied, according to Google:
- Browsers that don’t allow conversions to be measured with third-party cookies will have conversions modelled based on your website’s traffic.
- Browsers that limit the time window for first-party cookies will have conversions (beyond the window) modelled.
- Some countries require consent to use cookies for advertising activities. When advertisers use consent mode, conversions are modelled for unconsented users.
- Apple’s App Tracking Transparency (ATT) policy requires developers to obtain permission to use certain information from other apps and websites. Google won’t use information (such as IDFA) that falls under the ATT policy. Conversions whose ads originate on ATT impacted traffic are modelled.
- When the ad interaction and the conversion happen on different devices, conversions may be modelled.
- Conversion modelling covers both click-based events and engaged views for YouTube, to help with attribution for engaged-view conversions (EVCs).
- Any conversions imported into Google Ads from linked Google Analytics 4 properties will include modelling.
The same article also states:
“When looking at Google Analytics reports, keep in mind that attributed conversion data for each channel can still be updated for up to 7 days after the conversion is recorded.”
They’re basically saying you can’t use GA4 for the last seven days of data because it’s likely to change the attribution. Google will continue to model your data days after it happened, which is definitely going to cause some reporting headaches.
Modelled conversions and reporting
The attribution modelling methodology isn’t a completely new concept – in Google Ads, the data could continue to update because conversions are always attributed back to the click. It’s just new within Google Analytics. Google Ads has always had an interaction time attribution approach, whereas Google Analytics had a conversion time attribution approach. So, in Google Ads, you expect data to always be in flux and changing. In Google Analytics, we have to start expecting that.
The changes that GA4 is introducing is about plugging the gaps in what’s already happened – you take data that is partial and then Google models the difference, or at least what it expects the difference to be. It then does the conversion modelling, where it tries to reattribute the channel for the conversion. And then it applies a machine learning model to do data-driven attribution across that before we see a report through the UI or API.
Previously, we could go in and validate the accuracy of the data to maybe a 5–10% difference. Going forward, there won’t be any indicators to say this isn’t accurate. For the old school analytics people, the biggest fear is, how are we going to know if things are right or wrong?
This isn’t a new problem though – it’s a case of technology catching up. The increase in legislation around user privacy is, in principle, a good thing. The use of modelling is going to become more and more prevalent, both in Google Analytics and other marketing analytics and ad technology. So we have to adapt and accept that we’re going to have less and less visibility. We’re going to move away from counting things and have to start trusting what we’re told.
If you’re interested in Dan and Dara’s full breakdown of modelling technology and if this is the end of real data in analytics, check out episode 11 of The Measure Pod.
George Mendham
Subscribe to our newsletter:
Further reading
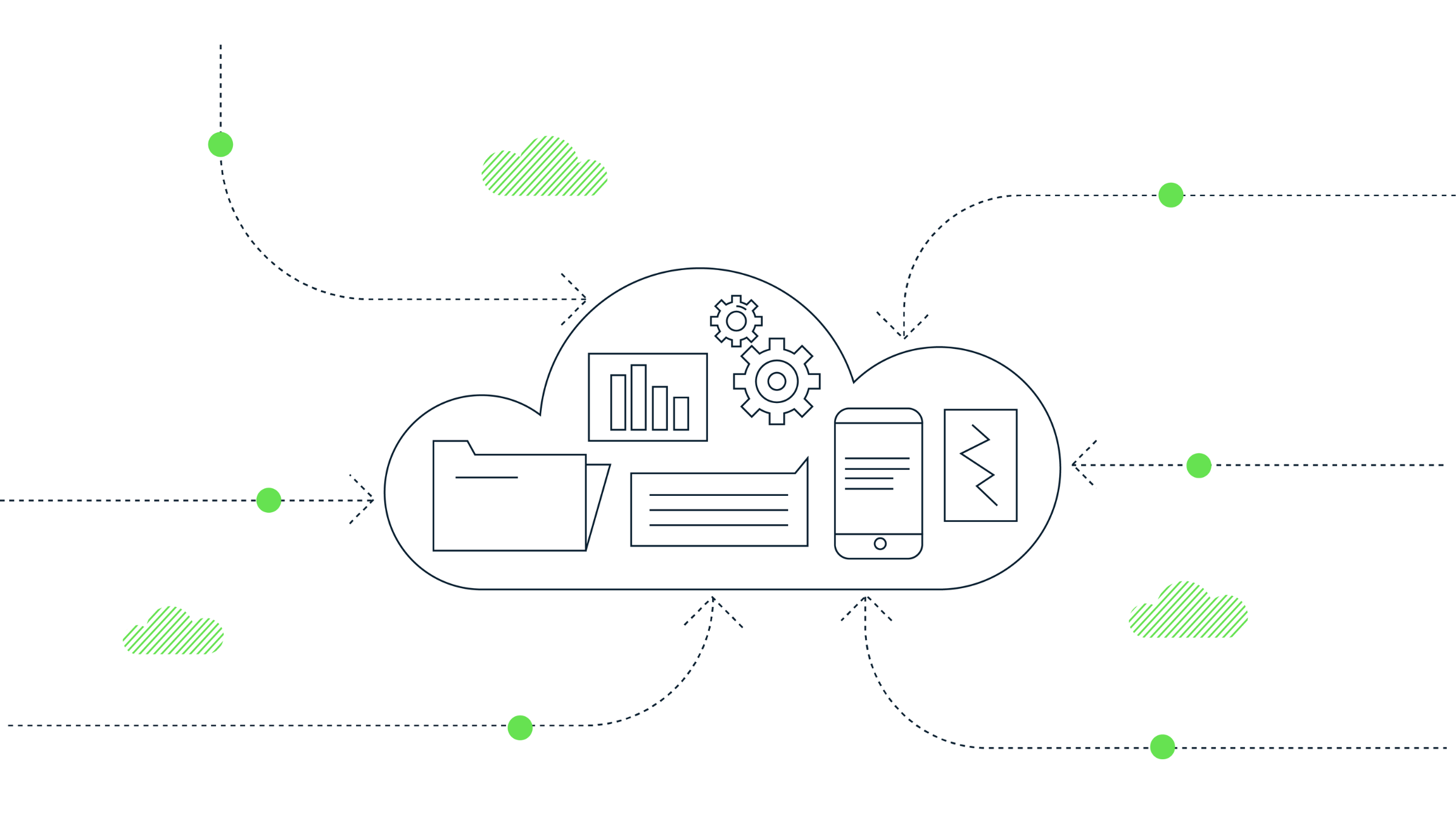
How to extract GA4’s event sequencing in BigQuery using the new batch fields
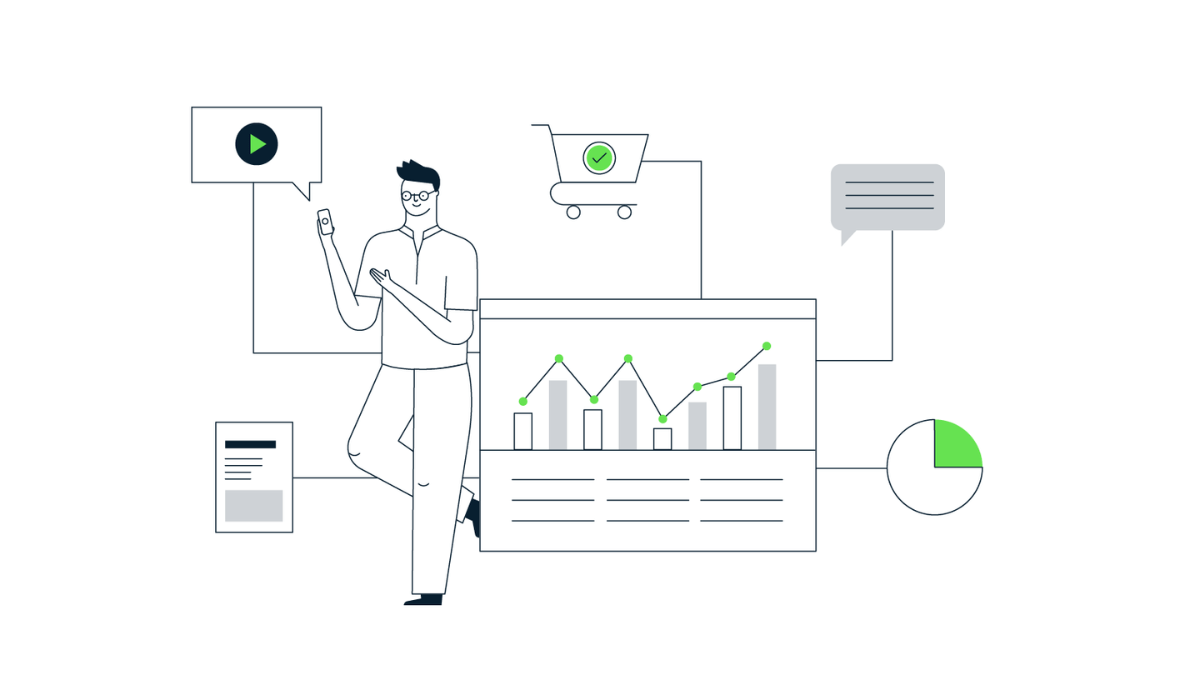
How to set up an SFTP Server on GCP (for Google Analytics data import)
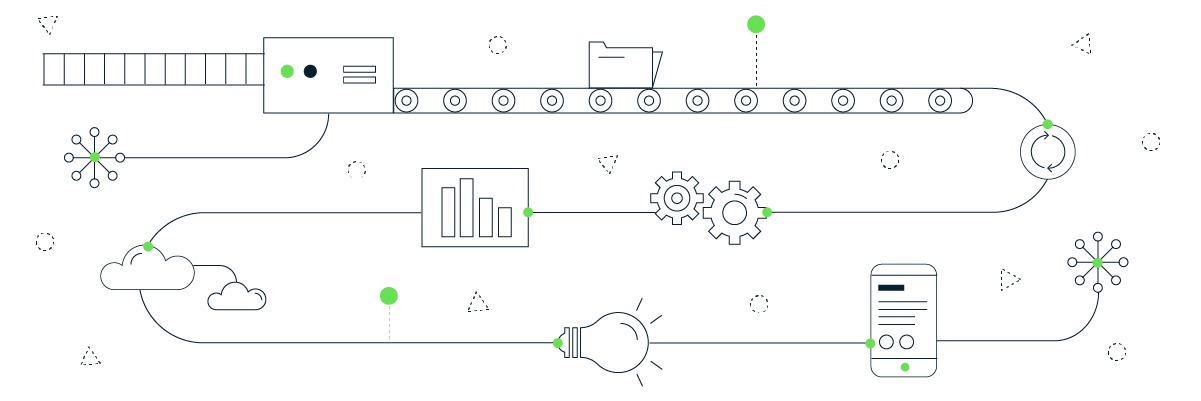