#85 Who cares about data literacy?
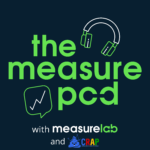
Join Dan, Dara and Bhav dive into the topic of data literacy. They share their personal definitions and perspectives on the subject, discussing the importance of data curiosity, data storytelling, and effective data communication. Bhav also explores the idea of data literacy as a dragon to be slayed, delving into the philosophical aspects of the topic. They all hope to reassure anyone listening that data literacy is something that can be developed and built upon, emphasising that everyone has some degree of data literacy, whether they know it or not!
Show note links:
- Join the CRAP Talks Slack community
- Bhav’s LinkedIn post on being data-driven and data literate
- Simo’s post on the myth of the non-technical marketer
Follow Measurelab on LinkedIn and on Twitter/X.
Find out when the next CRAP Talks event is happening on LinkedIn.
Music composed by Confidential – check out their lo-fi beats on Spotify.
Master Google Analytics 4 with Daniel Perry-Reed on the next GA4 Immersion 6-week cohort training course. Early bird, charity and group discounts availible!
Quotes of the Episode:
- “Data literacy is being able to translate data into useful information and practical actions.” – Dan
- “Data literacy is being able to use words and numbers to express thoughts and ideas, to make a point, to make an argument, to help somebody else understand what your point of view is or your experiences, and that person you’re communicating with has a kind of similar understanding of those words that you’re using.” – Dara
- “Being data literate is one who walks the path of data without fear” – Bhav
Let us know what you think and fill out the Feedback Form, or email podcast@measurelab.co.uk to drop Dan, Dara and Bhav a message directly.
Transcript
The full transcript is below, or you can view it in a Google Doc.
Intro
[00:00:15] Daniel: Hey, welcome back to The Measure Pod. I just want to start by apologising. I jumped straight into this episode, rambling off on a topic that I was really keen to talk about, data literacy. So, yeah, I don’t do a really good job or any job at all of introducing the episode, but this episode is all around us talking about data literacy, us giving our definitions of what we think data literacy is and what you’ll find towards the end as we go on and on talking about literacy, curiosity, storytelling and this idea of data communication is that I think we all agree? I don’t know, did we agree? I can’t remember. But I think we’re all on the same wavelength, but we’re coming at it from different angles and Bhav maybe you could give them a little sneak peak about slaying your dragon.
[00:00:52] Bhav: Yeah, I mean, I kind of like the mysticism view of taking data literacy and thinking of it as a dragon, you need to slay. And I give my definition on the podcast, so I won’t go into it now, but I think it was, you know, I think for me data literacy is a very deep philosophical topic, and I hope we, I hope we did adjust this on the call again you know, I’ll leave that to the listeners to decide.
[00:01:13] Dara: Just thinking about it, and we didn’t actually cover this too much, following on, on that thought, I hope people also take away from this episode, if anyone’s listening who doesn’t see themselves as being particularly data literate, I hope they come away from this realising that it’s not something to feel too fearful about. Everybody really has some degree of data literacy, and it’s something that you can build and develop, but there’s nothing worse than feeling like everybody else has something that you don’t have. So hopefully people will realise from our conversation that actually it’s a, it’s a scale and you’re going to sit somewhere on that scale, and that could vary over time, but it’s not something to be, certainly not something to be ashamed of.
[00:01:49] Daniel: Awesome, well enjoy the episode. Get in touch if you want to share your views on data literacy, storytelling, and data narratives and communication. You can find us, there’s a link in the show notes to submit a form if you want us to talk about something or head over on to slack, Bhav, there’s a CRAP Slack community, right? It’s probably worth them jumping in there and we can spin up a conversation on one of these episodes.
[00:02:10] Bhav: Yeah, if you follow the following link I think that Dan, you’ll probably put it into the show notes, sorry, but there is a Slack community. Come in, join us, ask questions and get lots of answers hopefully.
[00:02:20] Daniel: As long as it’s all nice and agreeing with us obviously. If you’re disagreeing with us then you can go somewhere else, it’s fine. Alright, hope you enjoy the episode.
Topic
[00:02:29] Daniel: So I was listening to a podcast the other day talking about the whole subject of data literacy, and it made me think about, well, it made me think about data literacy, put it that way. But it was actually more on the context of how there’s the big conversation that’s kind of forever going around. AI, machine learning, the LLMs, I can never say that acronym properly, and just the rise of those and there’s this kind of like background fear of whether or not there’s like longevity in careers, what jobs are good to invest in, and what’s going to be taken over by quote unquote the machines over time.
[00:02:58] Daniel: It was making me really think about data literacy and how I think that although the robots and Skynet is getting really advanced, it still can’t quite get to a point of translating data. It can’t understand data. It can just read facts and figures and it can present them in a certain way. But when I was thinking about data literacy, what I was thinking is actually, it became quite evident as I was thinking about this and talking to a couple of people at work, is that I think my definition is slightly different to other people, and I think everyone might have a slight different definition of what data literacy is, what it means, and I suppose how off the back of that, how relevant it is. So what I thought could be fun, assuming you guys are up for it. What I thought could be fun is us going round and us defining what we perceive the definition, or at least what our version of data literacy is. Now, I haven’t done any like official dictionary definitions here. I haven’t Googled anything else to kind of sway my answer. But if it’s good with you guys, I thought the subject of data literacy is really interesting. I think it’s something that is coming up quite a lot at the moment because it’s like the things that machines are striving for and it turns out to be quite contentious in terms of how we define it and who you are. So what do you reckon? Should we do this?
[00:04:05] Bhav: I really thought you were going to name drop GA4 in there, Dan when you said that it’s really relevant now because with the new tool comes new requirements of data literacy and learning new, like new features and functionality.
[00:04:18] Dara: You haven’t heard his definition yet, that’s probably going to be like stuffed with the word GA4.
[00:04:22] Daniel: Fair enough. I just want to remind you though, that I haven’t brought up GA4 for a little while now and it’s still being held over me as this thing, so I may as well lean into it and just own it. Let me kick things off and give you my definition and I’m going to play the game, which is the least number of words wins. So I’m gunning for victory here. So for me, what is data literacy? Data literacy is being able to translate data into useful information and practical actions.
[00:04:46] Daniel: It’s all about translation. It’s not about data presentation. It’s not about the data itself. It’s turning the ones and zeros into, and I use these words deliberately, information and actions. So useful information and actions. So can I take what something is saying, how many times something’s occurred or not occurred, or the ones and zeros that one might find, for example, in GA4? And can you turn that into actual information that someone can go make an action with? And so I suppose this kind of bleeds that line of data storytelling or that kind of narrative or building a narrative I think is probably a better way of thinking about it. Can you build a narrative around data so that you can actually do something with it? Because a lot of people don’t understand if sessions go up 5% month on month, that’s not, that’s not inherently an action or information that can be useful it’s just a number, it’s just data. So it’s translating that into a story that can be actioned upon and hopefully improved.
[00:05:36] Bhav: I like that. Dara, do you want to go next? I’m curious to hear your really long one now actually.
[00:05:40] Dara: Oh well, I don’t know, I don’t know how long it’s going to be because I’m basically going to just, it’s going to be slightly off the cuff. This isn’t part of my definition, so don’t start counting the words just yet, but I’m curious and we can come back to this. I’m curious to know why you think it’s contentious, Dan. Because in my head maybe I just have a very simple head. I think it’s simple, I think defining it is simple, achieving it maybe is much less simple or achieving consistency with it across different people in an organisation.
[00:06:06] Dara: So I’m going to start with like, I’m going to say normal, traditional literacy. It’s being able to read and write, but not just for the sake of it. It’s being able to use words and numbers to express thoughts and ideas, to make a point, to make an argument, to help somebody else understand what your point of view is or your experiences. And it’s communicating using those words or numbers in a way that there’s context and that person you’re communicating with has a kind of similar understanding of those words that you’re using. So data literacy is not much different, it’s just using all of the information that we have in the digital age. So it’s using data, understanding it with context, being able to interpret it, and being able to use it to either express an idea or a thought. Or back you up in a debate or justify a position that you have. That was a lot of words, but I stand by them.
[00:07:00] Bhav: I mean, in terms of length count, I think Dara, you’re probably going to lose, but I like the definition nonetheless.
[00:07:06] Dara: I’m happy. I’m happy with that.
[00:07:07] Bhav: So Dan, when you asked us to have a think about this before this podcast, you specifically said not to Google any definitions of data literacy. So I tried not to, and what I’ve done is I’ve gone down a much more philosophical route just to make it interesting and I’ve always been a fan of like Eastern philosophy and Confucius and all of that, kind of like that stuff. So I’ve gone down the Confucius route, and I’ve said my definition of data literacy is one who walks the path of data without fear. And by this what I mean is often data is the biggest hurdle for most people to overcome and build literacy is, it’s really overcoming that fear. And they look at data like a dragon of knowledge and fastness and of extreme power. And actually, once you slay that fear, once you slay that dragon, you can master the data in all its form. You can use it, you can interpret it, you can yield its power for good or for evil, you know, whatever you might, you know, whichever way you lean. But for me, I’ve gone down a very philosophical route.
[00:08:06] Bhav: Only because I think the topic of data literacy is a very philosophical topic in my view. I’ve always hated approaching this in a purely academic point of view. I’ve always liked approaching this in a very philosophical space. So for me, it’s one who walks the path of data without fear.
[00:08:20] Daniel: I think you might have won the word count as well, so I think double bonus points there. I like that it’s a state of mind, it’s not like an achievable kind of line to cross or an exam to pass there. I completely get what you mean as soon as you said it as well, Bhav, I really like your definition. I fully understand exactly what you meant as soon as you said it. It’s that, it’s that fear of the blank page. It’s the fear of the white page saying, okay, what do you want to see and how and why, and what does it mean? All of those things are starting with a blank page, and you have to create something from nothing.
[00:08:49] Daniel: And actually, if you can get over that fear by various different methods, you can just learn different tools and equip your tool belt, your mental tool belt sufficiently. And over time you start being more familiar with different problems and approaches and things like that. If you get over that fear of the white page, then actually, you know, the world is your oyster.
[00:09:06] Bhav: Like you said, I don’t think there’s a sort of like, you’ve completed data, right? Like there’s, it’s not like data completed it, you start off at level one, but just as like start off at level one requires knowing that they’re, you know, you’re a beginner and you have to complete level one, then you go onto level two, you complete level two, you face the boss, you move on to level three, and so on and so forth. And again, the concept of data literacy is on a spectrum, right? There’s literacy in the can you read an Excel file, all the way to the other end, which is can you take like raw data and it’s, you know, from a data warehouse and manipulate it in Python and build some machine learning model out of it, right? And actually for some people being data literate just means being able to get through the first one or two or you know, or three levels.
[00:09:48] Bhav: And for other people who are maybe more, they want to complete, you know, the hardest level of data, they want to go down this route where it’s like, actually they complete all levels plus the bonus missions, plus the big bosses, and they do it on the like extreme, hard level setting. Each one requires a mindset and ability to get over it. So that’s why I say it’s such a philosophical thing because it’s such a grey area.
[00:10:09] Dara: I think it’s just an extension of language. I actually think that the more complicated people try and make the definition or the more complicated they try and make working in data out to be, that then creates that scary dragon that people want to back away from. It’s like just walking into a room where everybody else is speaking a different language, it’s obviously going to be overwhelming. But once you start to learn the basics of that language, then you can have a basic conversation. And then the further you take that, the more advanced that conversation can get.
[00:10:35] Dara: The age we live in now, it is like that next extension of language. It used to be just words and some numbers to go into a shop and buy something and know how much change you should get back. Now this is like that next level of that and because of that, I think it’s actually not just something that’s relevant to us working in a data field, but I think it’s, it’s relevant to everybody in society. I think it would be dangerous to go through life these days without some degree of data literacy because of your information being collected and used online. So I think it goes way beyond even what we do in our daily lives and goes out to, we are getting philosophical here, but it, you know, I do think it relates to society in general. I think it should be in, you know, people should be taught it from young ages to understand and kind of develop data literacy. Just like you would learn language, both your own and maybe a second language and learn numeracy skills as well.
[00:11:30] Daniel: I think this kind of ties into a little bit to my point, or at least my thought around it being potentially contentious, and this isn’t like coming up with a complete opposite perspective of what you guys have said or the kind of the kind of norm approach to data literacy. I just think that, going back to your analogy there of it being a, a language like going into a room with other people that are speaking a completely foreign language to you. I think it’s the idea for me though, it’s not about the reading, that’s the important part, it’s the translation, it’s to be able to speak it. It’s about translating it to people that don’t have a full awareness or data literacy, or they’re not as literate as you are. So it’s not about going into a room and understanding what people are saying. It’s about then understanding that and maybe translating that back into your native tongue, to someone else that might be in the room there.
[00:12:13] Daniel: And I think for me, being data literate is actually taking the understanding or the meaning behind something, not just the things at face value. I think everyone is numerically literate to some extent. If you have a mortgage, everyone’s aware of interest rates and what effect that has on your day-to-day life, right? But I think for me, like the bit that I’ve zoomed in on and focused on is this idea that we’ve got plenty of people within our industry that work with data on a day-to-day basis. And I wouldn’t necessarily say they’re data literate or at least very data literate. I think they’re capable, they’re data capable, or they’re numerically literate. They can work with data, but they don’t have to truly understand it or care about it or, or know it truly in any meaningful way, but being able to understand what those ones and zeros mean, what the data means in whatever format, rule or otherwise or cleaned, but to then take that and say, this is what this means and this is why it’s important or not important. I think that for me is the key part of the literacy here. I think this is the thing that, I started this idea with things like AI, machine learning and you know, like the ChatGPTs of the world, the large language models. That’s the bit that I don’t think they’re there yet, or at least they’re slowly getting there.
[00:13:12] Daniel: So I don’t think there’s any danger if you are truly data literate, if you can translate ones and zeros into actions and insights you know, that term’s very overused. Didn’t really want to use the word insights, but can you take ones and zeros into something meaningful to someone that necessarily doesn’t understand the data? I think that’s the key part, and I think that’s the bit that they struggle with and that’s the bit that’s safe from the Skynets.
[00:13:32] Bhav: So I think in order to get to that point. I mean, you’re right, it’s interpreting data as well, but that requires an element of domain-level expertise, right. Which an AI model wouldn’t have. So let’s say for example, let’s say you’re looking at your sales numbers and they’re going down. An AI model, an AI model, or even someone who can read the data, should be able to tell you with some degree of certainty, is the graph going up or is it going down? Does it fluctuate?
[00:13:57] Bhav: Now the question really is, why is it going down? So that becomes the next thing. So this is where domain expertise comes in. Have you seen a shift in the market? Have we seen an economic downturn because of the state of the economy? And then the next question is like, what do we do from here? And I think all of these start to play a part in that sort of concept of data literate, and this is why I said data literacy is on the spectrum. You can read the data, you can understand it, can you interpret it? Can you infer from it? And then can you make some projections or some actions off the back of it?
[00:14:27] Bhav: But I think domain expertise plays a big part in this. If you’re not a domain expert, I can read a graph in the Financial Times paper, and I can look at it and say, okay, well this number is going up, this number is going down. Why is it going up? Why is it going down? Then there’ll be a whole bunch of stuff that’s happening in the economy in our country and other countries that will explain why GDP, for example, might be going up or down and I don’t have that context. So I think context is key.
[00:14:49] Daniel: Context is key, but I think this is integral to, you know, working in analytics, working in marketing, working in product. You can’t get away with not having domain context. You can’t get away with not understanding the client in some context. So we need to understand, okay, we saw a spike in sales. Okay, well, did they have a sale on? But having these questions, even if you don’t have the answer to hand, even if you’re not there yet, But having this awareness of what could be the contributing factors? Was there an economic change? Was there an issue with a website or the app? Was there a sale that they had on? Is this seasonal for them?
[00:15:19] Daniel: Having an awareness of these things that could impact it, I think that literacy is being aware of, okay, what questions do I ask first? Where do I start to make my investigation more efficient? And I think this is the thing that happens over time. Like I said, you’re arming yourself with a tool belt, but I don’t think you can get away with working in data without having a context of the business in any way, whether you are an agency, a consultant, in-house, you can’t just sit there and work with data. I don’t think that’s enough. Like you can’t be truly data literate if you’re just playing with ones and zeros and just kind of walk away afterwards.
[00:15:49] Dara: I don’t disagree with that, but I want to go back to the point about AI not being there yet, or these LLM models not being there yet. I’m not so sure about that, I think there’s probably pros and cons to a chatbot versus a human doing certain types of analysis and interpretation, because obviously you’re right, like it does all come down to context, and that’s the same whether you’re using words or numbers or charts or data or infographics or whatever. If you don’t have the context, then it’s meaningless. But I think it’s not always as hard to find as, you know, where do we get our context from, from information? So you feed that same information into the model, then it’s going to have similar context to what we have, but the advantage it will have over us is it’s going to have, it’s going to be able to retain and recall any piece of information that sits within Its brain, unlike me anyway.
[00:16:38] Dara: So there’s probably going to be something it’ll miss. Maybe it won’t quite get the messaging right the first time around, but I don’t know, I would say let’s just totally throw this out there, but I’d say more often than not, if it’s got the right data fed into the model, you’re going to be able to get to a point where it’s presenting the information in a way that’s going to make sense more than you would with the majority of people. Because each of those people is going to have different varying degrees of that context. They’re not going to have the whole picture. So, you know, if you plug away at it, you ask the right questions, and you say, sorry, that doesn’t quite make sense, and you refine your prompt over and over again, I think you’re going to get to a point. You’re also not going to piss the AI off, whereas if you keep asking a person to dumb it down, dumb it down, dumb it down, they’re eventually going to say, I’ve tried so many times, I’m not going to, I’m not going to keep explaining this to you.
[00:17:26] Dara: So I think, you know, there’s pros and cons, but I’m not convinced that it can’t demonstrate data literacy, that it can’t interpret data and present it in a way that would make sense to somebody who doesn’t understand all of the kind of nitty gritty in terms of like how that data’s been collected and processed and manipulated and whatever else.
[00:17:46] Bhav: On the topics of AI and LLMs I try to hold back because I know it’s going to, if it’s not there, let’s say Dan to your point, it’s going to catch up and let’s say Dara, you’re right, it’s if it’s already there, if you feed it enough data, I agree. Like all of this stuff will be easily accessible for anyone right? For me, the concept of is this mainstream yet? The answer is no. Like is it something that you could walk into any organisation and use these fancy tools? No you couldn’t, which is why for me the concept of data literacy is still very much a human problem. And even I think the people who are very data literate will be able to take advantages of technology to get even better at it but for the everyday person, you still need so much underlying basic knowledge that, you know, like using LLMs to solve, you know, answer intricate data problems probably still some way away from there.
[00:18:42] Bhav: I don’t want to extrapolate and make an assessment of like years, is it years? Six years, you know, whatever it might be. But we’re definitely not there right now. If I walked into any organisation and I said to a product manager, do you consider yourself data literate? I’m probably going to get yes, no, somewhat right? And the yes is going to be, they can go into their data warehouse, they can go into their analytics platform, extract some information, they’ll be able to chart that information in a way that helps them and their stakeholders visualise that data. They’re probably going to be able to annotate what they’ve seen, and they may be able to add some context around it and that, for them, that’s going to be more than enough.
[00:19:17] Bhav: For the maybes it’s going to be, I can’t get the data, but if someone was able to give it to me, I could manipulate it myself, like in Excel or Tableau or whatever data visualisation tool they’re using and then do the rest and then the no’s are going to be, I don’t know how to get it, even if I got it, I don’t know what to do with it, I don’t know how to visualise it, I can’t even do a VLOOKUP in Excel. So for me right now is, those are the key sort of like internal problems that I think about when I think about data literacy. You know, I’m not even going down the root of like should we be teaching people how to write SQL code because you know, that’s a million miles away. Like first can I put data in someone’s hand? Can they manipulate? And if you think about it, like I don’t know when the last time you guys did a, an interview case study was, I assume it’s probably not been for a while, given that you’ve been at Measurelab for so long.
[00:20:04] Bhav: But usually what happens is you’ll get just like a raw data dump, like in an Excel file or something like that. They may even give you access to a dummy database. And then you write some basic scripts and they’ll tell you, these are the tables, these are the keys, and these are the definitions of all of the columns inside it. And then you are expected to go away and find some magical insight. Now here, I mentioned data literacy around context and domain knowledge. In this instance, you don’t even have any context. They’re just saying, can you go and find some insights? And what they mean by here is, and I think this maybe comes down to the most basic definition of data, is can you go in, look at this data, chart it, draw some insights, make some recommendations. It’s a very basic thing, but it’s so fundamental to so many roles that are not data roles like marketing, product you know, so and so on and so forth.
[00:20:46] Dara: To try and kind of follow on from that, I guess, and to go back to my definition a little bit like I do think it is back to saying it’s a bit of an extension of language because if you, if that role that you were interviewing for was a creative role, you’d be asked to do a similar thing, but instead of using some code or a database and some SQL, you’d be using either the words or you’d be using images. So it’s like, here’s some raw material, we want you to interpret this and present it back to us in a way that we’re going to find interesting or show that you know what it means and that you can work with this kind of raw material, whether that’s words, numbers, or whatever. So it’s kind of similar, isn’t it?
[00:21:25] Dara: What you want to see in that interview is you want to see that obviously the insights are going to be completely made up or they are going to be based on that data, but it’s not a real example. You want to see that somebody does something that you might’ve done or something that you’ve seen before, something that you know relates to a real life example where you think, oh, they’ve come back and they’ve said, actually these two columns are supposed to be the same, but the numbers don’t match or, you know, I’ve noticed that there’s a huge spike on this date and then I looked up that date and I realised that that was you know, the day the Olympics started, and that might be why this happened, or whatever. You’re looking for somebody to be able to kind of demonstrate to you that they’re able to ask the right questions, they’re able to kind of interrogate that and they’re able to kind of create something with it. So again, I kind of think you could interchange that with words, numbers, or other kinds of data, information.
[00:22:10] Daniel: Yeah I get that. I think it’s creating something from nothing right. The idea there is you’ve got something. You are creating something from that, whether that’s, you know, going back to your example, Dara, whether that’s like a design and you know, an image of the back of a text prompt, you know words, whether that’s you’re given data and you’re creating a narrative in words, you know, you can kind of take these things. I think from a data literacy perspective, and I think this is where I was leaning quite heavily into it, is that it’s not about the techniques or the tools. It’s not about the can you transform this data? Can you clean this data or validate that it’s the same or should be the same as this. It’s more like, can you tell me why this column or this number’s important?
[00:22:47] Daniel: You can strengthen that muscle, you can practise this. But it comes back to kind of like using the kind of, I suppose it’s like logical thinking in a sense of like, okay, what could be the causes there, rather than run through every possibility, can we be like, okay, well let’s be pragmatic and let’s see, okay, what does this mean? What does this number mean? What can we do to that? Is that useful in a, in a kind of practical aspect? Is there anything I can take from this that’s meaningful? And I think this kind of goes into this idea of like, that depends on the audience. So the being data literate to the CMO is different to the MD, different to the paid search manager, for example. As an analyst, if I’m a data literate analyst and I’m kind of working in the paid media team versus the product team, it’s going to actually mean different things and depending on who asks me.
[00:23:28] Daniel: And I think that kind of what you were saying, I think Bhav earlier around the people having different levels of literacy as a spectrum of course, but I think this is something that it isn’t about your ability to be data literate because you are, if you are performing the analysts role, I suppose, let’s say you are the analyst in an organisation for whatever team, the most important impact is the person who’s using this information. You can come up with all of the insights, all of the actions, all of the thing possibilities. But if you are not the one putting them into action, actually, is that kind of a wasted effort? And I think this, this goes into that idea of like, is data literacy a company-wide thing or is it an individual thing? And is there a need, for example, to everyone have the same level of data literacy? Or actually, is it something that is scaling depending on the role, the seniority, horizontally, vertically, that kind of stuff.
[00:24:14] Bhav: You’re right. It got me thinking that data literacy it is ring-fenced in your domain and it does kind of touch on what I’m, what I was talking about around domain-level expertise. For example, if I’m the CFO, I don’t need to be able to be literate in the social media data. I just need to be able to understand financial data, interpret it, extrapolate it, forecast it. Now, if I’m on the other hand working in engineering, I don’t need to be able to understand what the financials look like, I need to be able to understand things like site latency and time to load all of those backend type metrics that help them understand how the website is performing. So I guess I still think there is an element of domain knowledge that goes into it otherwise, you could put data in front of anyone and whether they’re able to understand it, you know, yes, the short answer is yes okay. This number goes up, this number goes down, this number is flat. What does that mean? That will come down to your domain.
[00:25:04] Bhav: If you put the right data in front of the right people, they should be able to understand it. Maybe that’s what data literacy means, is if you have the right data that’s within your domain. Can you understand it? If I put, you know, in front of you guys, if I put, I don’t know, fashion trends, so I assume you guys aren’t very into the fashion world, but if I put fashion trends in front of, if I put fashion trends in front of you, you can see what the numbers are doing and they’re going up or down, but you probably wouldn’t be able to understand why certain dresses or shirts are in season or why they’re going up. And, you know, it’s not your space to care.
[00:25:37] Dara: Yeah, I kind of think of it as like data literacy is, maybe it’s unfair to say it’s a means to an end, but why are any of us literate with words or with numbers? It’s so that we can communicate. And going back to your point, Dan, about like the different levels and it being a scale, I think that’s really relevant because I think you need to have a different level of data literacy, depending on what you’re trying to achieve. And then you need to be able to pitch that literacy or point it at different levels depending on who you’re interacting with. So if you work with data, if you’re a data scientist, your level of data literacy is going to be very high. But the people you’re going to be communicating with, they’re not all going to have that same level of literacy as you.
[00:26:15] Dara: So it’s almost like you need to be data literate in order to then work on being a good data communicator because you could be the most literate person in the world, but if you can’t communicate a message to the people around you because you just have all of these advanced concepts that nobody else can grasp, then you’re not going to get buy-in for whatever you’re trying to get buy-in for. You’re not going to be able to present a convincing argument because you’re pitching it at too high a level. So it’s like being data literate is the precursor to them being a good data communicator. And if you are in a role where you’re using data, day in, day out, your literacy needs to be high, but you also need to be able to communicate with people whose literacy isn’t quite so high.
[00:26:54] Dara: And then if you’re on the other side of that, you’re somebody who’s working. And this nowadays probably includes every single business. If you’re in a business and you’re working in a position that isn’t directly working with data day in, day out, you still need to be able to communicate with people who have a very high level of data literacy. So your own data literacy needs to be, you know, there’s a baseline and it needs to be at least that baseline. And then depending on who you are and what your role is. You might want to take it above that baseline to push yourself even further and be able to have even better conversations with those people who have a higher degree of data literacy, which again, is a bit like good language. If you need to be able to pitch, then you need to be able to pitch to different levels. And that’s a skill that’s in demand you know, somebody who has the information, has the knowledge, but can tailor that message depending on who it is that they’re, that they’re dealing with.
[00:27:43] Bhav: So I have a question on that because I think we’ve been talking about the concept of data literacy and definitions of what it means. And I wrote a post a while ago around, how on the outcome, why would we as an organisation, want to be data literate? And for me, data literacy drives data drivenness or the ability to make data-driven decisions as an organisation. I guess my question to you guys would be, if you keep that in mind, how do you measure data literacy and what is good enough? Because I think we can talk about the definitions and concepts and I think, you know, we’re all probably saying the same thing, we’re adding different angles and flavours, all of which probably seem correct but how do you measure it? Like how do you go about saying, yes, we are a data literate company. How do you cross that line? How do you complete that level?
[00:28:26] Daniel: I think I’m going to go full circle on the whole premise of me bringing this up to you guys actually, I don’t think data literacy is important. I think the data communication is the key part. And I think if you’re going to be a data-driven organisation, you have to have the communication nailed, and the better that you are at communicating what the data’s telling you, the more data-driven you’ll be. You could be, let’s say you’ve got an organisation that’s not data-driven in any meaningful way, they’re not very data literate, and they bring someone in who’s highly data literate, a data scientist, very clever person, and they do a bunch of models, they provide that. But if they’re not presenting that and explaining that and communicating that very well, if they’re not providing actions or tests or hypotheses off the back of that, then the business is going to go so what, I’ve got another dashboard I won’t look at.
[00:29:06] Daniel: Even when we talk about that, like when I talk about data visualisation, data visualisation is one of my, one of my passions and actually I always, I semi-joke about this, but it is 100% true. If you have a good looking dashboard with crap data, people will use it. And if you’ve got a bad looking dashboard or a bad interactivity-based dashboard with good data, no one’s going to use it. So actually it’s the same thing with data communication. You could have the most data literate person, but if they can’t communicate that, there’s no point, it doesn’t matter, no one’s going to listen. And so I think in terms of being a data-driven organisation, it’s actually very little down to literacy. And I think it’s a precursor, it’s a building block, but you can’t just be data literate and be data-driven.
[00:29:44] Daniel: I think you have to have that, I don’t want use the term, but the data storyteller right? This is the a job title that I think popped up for about six months, a couple of years ago. But this idea of can I communicate data? Can I communicate what this actually means, inwards to people that may not be there with actions, is there something that changes it from counting to let’s do this thing or experiment or test or kind of build a hypothesis off the back of it. And I think if you’re data-driven, the last part of being data-driven is actually saying, okay let’s do it. Let’s use the data to drive a hypothesis, to drive an action, to drive an experiment, to drive a test. But it can’t just be from literacy, you can have the most literate person, but if they can’t communicate that very well, the rest of the business is going to be like, well, so what the hell do I do with that?
[00:30:24] Bhav: So I’m going to just challenge one thing you said. You said data literacy it’s not as important as data communication. I would argue that, let’s say you did bring someone in who was data literature and they were a great communicator of that data. That still creates a bottleneck and you need data literacy in an organisation to ensure that everyone is able to access the data and then the communication becomes the next piece. If people can’t access the data or interpret it, or build some, you know, some visualisation at the back of it, they’re not going to be able to communicate it, okay, you can have the best person who does everything, but they’re going to be a bottleneck. And you want to create an environment which has data democratisation, baked into it so that anyone can go in, look at data and build it.
[00:31:07] Bhav: That’s really where you want to get to because in that way that allows your super data literate smart person to focus on the big problems, on the meatier problems that no one else is going to be able to tackle. You ideally, you want to be able to have people that go in and can do their own data analysis, you know, even that surface-level and communicate that because, you know, not everything’s going to require a data scientist.
[00:31:24] Daniel: I think this is a separate subject because I’ve got some views on data democratisation. There’s this idea of do you gate it or do you let everyone self-serve right? And you’ve got this idea of like open it up to the masses or, or control it, but kind of keep things ring-fenced, correct, and maybe streamlined, but again, I think if you are really good at communication, data communication, then you adjust your communication methodology to someone that may be not used to data. Like the thing is you don’t actually have to present numbers. If you can translate numbers into words, if you are building a dashboard, you don’t need charts like bar charts, pie charts, numbers everywhere. You can’t just write up a narrative. You could start by having a weekly email that goes out to the leadership team, for example, that’s just telling a story of what happened. You don’t have to share numbers, you don’t have to share exacts. But I think this is all in the narrative, the communication, and I think as the communication can kind of like educate and get people interested and get the sense of kind of excitement around, oh, what else can we learn?
[00:32:17] Daniel: And I think there’s like an education or almost like a passive education program going around that. And then maybe data literacy comes out the back of that through democratisation. But I don’t think the answer there to become a data-driven organisation is sharing access to raw data or to clean data in some way like that. And it’s not even jumping in with dashboards, it is just can the value of data be communicated effectively before we start showing that we have data? Because I think that’s often what I see, maybe the wrong way round in a lot of organisations where they get almost overwhelmed with so much data that they actually don’t know where to start and they don’t know why it’s important, so they just don’t look at it at all.
[00:32:53] Daniel: Whereas if you can tackle it from the other way round and say, look, we’ve got some great stuff we’re learning and then don’t even kind of reference the data or show the data, but over time it’s kind of like drip feeding this idea that all of this is coming from this data set. And I think that will start building up this kind of like, oh, I see why I would learn this. Why would I even log into this platform that gives me access to all this data? What’s the point? And you can actually start to build it the other way around.
[00:33:16] Dara: See, I’m going to throw out another term here. I’m not helping by just moving it from data literacy to data communication. But there’s another one that’s got me thinking about, so Bhav what you said about if you’ve got a really great data storyteller, if you’ve got someone who’s really literate, but can also communicate at the right level to the right people. I don’t think the organisation needs to be data literate, but they do need to have an appetite to be, so I think there needs to be some data curiosity in the company because if you are willing to learn, then you’re going to listen to that person. You know that classic thing of like the, what do they call it, the HiPPO, where it’s like there’s a bunch of clever people in a room and they work out what you should do, and then the boss comes in and just says, no I’ve got a gut feeling I’m going to do it the other way. So in that kind of organisation, you’re probably never, it doesn’t matter how good a storyteller you are, you’re probably just going to get shot down anyway. But if there’s an appetite within the business to listen to people who are data literate. Again, as long as they are also good communicators, then you might say, well, I don’t really understand this chart you’re showing me, but can you help me?
[00:34:16] Dara: If there’s no appetite for that, then you’re never going to become data literate. But maybe you can be lacking the literacy to begin with, hiring those clever people who can then help you to learn what questions you should be asking, and it becomes a collaboration. So, you know, if you’re the person who’s less data literate in that two-way communication, they’re helping you ask the right questions, and then you are listening to them and you’re using the information they’re giving you to make the decisions that you’re ultimately going to make.
[00:34:43] Bhav: It’s so hard for me to get on board with this idea because I’ve managed a number of analytics teams. And analytics resources are one of the most finite amount of resources any organisation has right? Let’s forget the data engineering resources needed to model that data and build that data so that the analysts can do their job quickly. Let’s just focus purely on the analysts. No company has enough analysts to be able to support every single stakeholder and every single request. And actually, you know, I go back to my definition of one who walks the path of data without fear. If people are able to comfortably get in and out of, you know, and it might be that they just get their data from dashboards. If they can take, say for example, you use Tableau and you can download the underlying data, then you can manipulate and show it however you want, even if you can’t do it in Tableau.
[00:35:25] Bhav: That for me is a big step. It’s someone who is comfortable taking data, manipulating it, reworking it, and then telling the story they need to tell without having to rely on someone in my team to be able to do it for them, because it’s not like a small task. I don’t want to belittle anyone’s work. Everyone has their own challenges and everyone has their own problems they need to solve. There are certain things that stakeholders should just be able to get and do themselves and they should feel confident in being able to do it and save the meatier questions for the really skilled and really data literate people. So okay, you guys have both sidestepped my question of how do you measure data literacy. So I’m going to ask you my next question, which is, who should be responsible for ensuring a data literate environment? I know like if you throw communication at me again, I’m going to cry.
[00:36:18] Dara: To prove I’m not just a question dodger, I’ll go back to your previous one. I think the reason why I didn’t answer is because I think it’s really difficult, if not impossible to, well, no, no, that’s not true. Of course, you can measure it, but you’re going to be measuring it in your own, I guess we’re back to definitions again, aren’t you? Or maybe it’s not even the definition, but it’s like, where on that scale do you want different people to be? I could imagine it becoming quite complex. You know, if you’re in this role, you need your data literacy to be an 8 out of 10, and if you’re in that role, you need it to be a 2 out of 10. It could get quite complicated, I think.
[00:36:49] Daniel: But I don’t think it’s a scale within like a 0 to 10. I think this is going to be a kind of what you were saying earlier, Bhav, it’s like it’s going to be relative to the role, and I think there’s going to be a level of data literacy. I think it is pretty black and white actually in your specific role of whether are you are or you aren’t. Because it’s basically saying, are you data literate enough for this role, for this position, for this team you’re in. And I think this is going to be, let’s say you’re on the experimentation team. You’re going to be like, well, how many hypotheses have you generated off the back of data? Not through, you know, like gut feelings and things like this. It’s like what’s the source of your hypothesis? And if it’s data related, okay, well I can measure that. If it’s a marketing team for example, and whether it’s a channel or the kind of the CMO, it’s like, okay, well how many times have we used data to make an informed decision or experiment within our campaigns?
[00:37:32] Daniel: Even if it’s just running a test to experiment there, I think you can quantitatively measure whether they’re successful or not. That’s another side of the story, but I think you can quantify the success of whether you’re data literate because eventually, and anyone working in experimentation or within that side of it are going to realise that the kind of the low hanging fruit and the gut feelings and the kind of community driven suggestions are going to dry up pretty quick, especially if you go really well and fast into the world of, I’m going to stick with my example of experimentation. So you’re going to have to become data literate sooner or later.
[00:38:03] Daniel: So I think you can measure it, but I think it is going to be a, it is a line of saying you are or you aren’t data literate enough for this role, and that’s going to be defined by your peers, I think, or your team. Now, if you’re the first person in that role that’s driving the train of thought of data literacy now I have no idea. I don’t know where you start there and how you do it, I think that’s just a moving needle that will just shift as you become more data advanced, data mature.
[00:38:23] Dara: But do you break it down then into it’s different, because there’s more than one component to it, isn’t there? So you could be thinking back to what you said a few minutes ago Bhav, and I actually had that experience in the past, by the way, where I hired somebody into the team, this is a long time ago. They were really smart, had a like, really good stats background. They could do a lot of really clever stuff and they could really do like, not just kind of for the sake of it, but really clever, useful stuff. Unfortunately, it was too early to have that person and it just, we weren’t able to use it. So what if you have somebody who okay, maybe that’s not the best example, but the point I was going to make is you could break data literacy down. You could have somebody who’s very good at understanding data and is able to do a lot of really important work that then needs somebody else to put that layer on top to then communicate it to another group of stakeholders.
[00:39:11] Dara: If you then had just this kind of like catchall, I know we’re not suggesting that, but if you had this single score for data literacy and that person was pulled down because they were lacking one side of it, but they could still be really useful. Does it become, am I just overcomplicating it?
[00:39:28] Bhav: I like the concept of breaking it down because I was going to say the way that I’ve measured, I’ve tried to measure in the past when, again, when I’ve been sort of leading data teams and analytics teams has really been around actually, who are our key stakeholders? Who are the people that my team work with day in day out? Can we get them to go through some type of basic training program? So we’ll have a module based on experimentation. One that’s based on say, Amplitude, like here are the 101s of Amplitude. Then the next one would be, you know, asking good questions. And actually if you have enough of these, then you can, you know, just by proxy, measure how data literate an organisation is.
[00:40:05] Bhav: Then it goes back to that saying, you can lead a horse to water, but you can’t make it drink it right? But as a data leader, you kind of have to kind of first at the very start, lead them to water and then hope that they drink it. So that’s how I’ve kind of, I mean, I’m not saying it’s been successful and I think other ways that people have done is by doing like drop-in sessions. So I’ve worked with teams where they’ve had like an analytics hour where over lunch you know, you come in, I’ve seen lunch-n-learns where you kind of demonstrate and you, you know, you try and teach people in the organisation different concepts. The thing with these things is that over time they naturally die. Initially there is this excitement, there is this like, oh yeah, you know, your first session will be like 25, 30 people maybe, but then the next one will be like five, and then pretty soon just be you and it gets very sad.
[00:40:47] Daniel: You could have been the most efficient communicator and just taught everyone how to be data literate on your first go, it could have been the case.
[00:40:53] Bhav: I mean it could be.
[00:40:57] Dara: Well that kind of tees back up to your, what was then your second question, which is who should be responsible for it? And I think like hearing you say that is just further convinced me of what I already thought, which is it has to be, it has to come from the top. I think if you are trying to convince other people in other departments or other business units to have an interest in this, and they don’t have an interest in it, then it’s going to fall on deaf ears. And it doesn’t mean it’s magically going to work if it comes from the top, but what it does mean is at least the whole company will have that in its DNA and it will be, it’ll be so people who show that curiosity will be rewarded and people who don’t, just won’t progress in the same way. But if it’s down to an individual within an analytics team or a data team, they’re just going to come up against brick walls if the rest of the business doesn’t buy into it, I think.
[00:41:45] Bhav: I think that’s a, you know, it’s a really good point actually, and I saw it first hand work at Hopin. So where I was last, which was Hopin, a virtual conferencing platform. I was the first analytics hire, so we had a data engineering team, but I was the first analytics hire. Up until this point, the product team that I was working with were largely doing their monthly product reviews based on outcome. Sorry, not on outcome, on output. So what have we delivered this month? You know, how far are we behind schedule? Are we going to hit target? Things like that.
[00:42:13] Bhav: I kind of joined and I did put all of the foundations in place. We’ve got the, you know, the team in place, we embedded analysts. We started working with them, we started identifying metrics. It wasn’t until our COO joined and she was ex-Amazon and she changed the product, the monthly product review meetings to incorporate an element which was all about outcome. And suddenly there was this shift in culture where we suddenly had metrics fully, properly defined in all teams. We had the dashboards built, we had event tracking being rolled out very quickly. We had PMs who were taking more of an interest in the numbers, how they were doing, what was going up, what was going down. I introduced a fortnightly metrics review meeting for our leadership team to be able to see on a very regular basis how all of our key performance metrics were doing.
[00:43:01] Bhav: So you know this was started off with our North Star metric and then it worked it’s way down. Obviously, we didn’t go right into depth with each other product-level metrics, but the product teams themselves had monthly product reviews where they were then like, okay, let’s go these are your metrics, let’s go through them. And suddenly you know, we didn’t call it data literacy. You could argue that there was this sudden shift in mindset, we had moved the needle significantly in creating a data-driven organisation, which was built on the foundations of data literacy. I’m very proud of that story, by the way.
[00:43:29] Daniel: So you should be.
[00:43:30] Dara: Yeah, you can tell and it is an example of a case where it did need to come from you know, it does, or saying come from the top. It’s almost a bit cringe saying that, but it has to be baked into the company, doesn’t it? It has to be like integral. It can’t just be, hey, you seem to like data, why don’t you be the data evangelist and convince everybody else who doesn’t care that they should care? Like, that’s just not going to work, is it? So there’s got to be either, it’s either got to be a collection of people that are senior enough to be able to, you know, actually make change or it’s got to come from Yeah, it’s got to be already baked into the culture or the DNA of the company.
[00:44:06] Bhav: In that instance, it really helped that our CEO was data literate. You know, she didn’t need to understand the breakdown of each metric or where it came from, or how it was defined. It just helped that she could look at a number, understand its place in the wider ecosystem, and hone in on it. And it was, it was an incredible skill like I’ve rarely seen it in leadership.
[00:44:25] Dara: That Kind of proves the point, isn’t it? That it’s not about necessarily knowing everything about one aspect. You don’t have to have the deepest knowledge of the ins and outs of how all this data is collected. You just need to know how it pieces together. You need to have that understanding of why it matters and what you can actually do about it. If it goes up or down, you don’t have to be a technology expert, or you don’t have to be a data scientist. You just need to know what place that takes in the conversation and how you can actually use that information.
[00:44:51] Daniel: So I have a question for you both then just to, as we’re kind of nearing, nearing the end of our time here and definitely not even scratching the surface on this, and I’m sure everyone else has got a thing to add, or a perspective on this too, but let’s say I am in an organisation and I’m wondering whether or not my organisation is data curious (TM) or data literate or there’s an element of like a data culture there or whether or not they can try and change that. Maybe they’re in a leadership position, maybe they’re not.
[00:45:16] Daniel: Where do you start? Like how would you assess whether or not you are even, if you think you are or you’re not, and even, and how would you get better at it? Let’s say you’ve done the assessment, how, how do you get better at being data literate?
[00:45:26] Bhav: So I think it starts with understanding how data literate you need to be as an organisation. If you’re a very early stage startup and you barely have your, you know, your data engineering it’s higher standards and you’re just collecting data and you’re shoving it into a data warehouse or something like that, you probably don’t need to be a data scientist level of data literate. You just need to be able to take that information, provide a sort of like a heartbeat of the company, right? So you understand what your core metrics are, visualise them and be able to demonstrate to your organisation that all your heartbeat metrics are performing optimally, and you’re heading in the right direction, your health is good.
[00:46:04] Bhav: You’re essentially, you’re keeping your finger on the pulse. Then as an organisation, as you start to grow and as you start to mature, you want to start answering some of the more under-the-hood type questions, you want to start peeling back that the, you know, the layers of the onion to start asking, okay, why is this happening? And this starts to become a point where you might yourself not have all of the answers, but you recognize you need to bring in experts to be able to answer some of those questions. So I think as an organisation, whether you’re a startup, mid-size organisation, or an enterprise organisation, you should be able to answer the finger on the pulse type questions about your organisation. What does the health of my company look like? If you can’t do that for me, that’s a fundamental problem, right? Like, that should be the very least, that should be the lowest level of data literacy that I would expect from any organisation. Can you tell me how healthy my organisation is? Then how much further you go depends on what Dara said, which is around data curiosity.
[00:46:56] Bhav: Do you have people in the organisation who are asking you, so me, you know, for example, the right question about the data, and as a data leader, if I can’t answer them, I’d want to go and find the answers. Am I equipped to go and find the answers? Do I have the budget to hire the people to find the answers? Can I bring in an agency? Can I do some type of self-serve? Can I get from tooling? And that’s for me, is how you’d probably go about assessing. It’s like start off with the very basics, if you can’t even do your basics right, then forget about, you know, moving on to the more advanced stages.
[00:47:23] Dara: On the second part, I’d love to answer the second part of the question, which was how do you then kind of upskill? But to be honest, I actually don’t know could working in a specialised analytics company that is a small company, I’m not best placed to answer that one. I’d love to have an answer to that, Bhav, I don’t know if you have experience from working in larger companies, but how would you go about it? Because again, if you are in the data team, your skill development’s going to be very deep. Whereas if you are, you’re C-suite or if you’re a marketing manager or marketing director, how would you go about upskilling and becoming more data literate when your needs are a bit broader and less deep.
[00:47:59] Bhav: I guess it comes down to urgency. If it’s urgent to have those needs, you have to bring in specialists. I’m probably the worst analyst in the world, you know, of all the analysts in the world, I’m probably the worst. But I don’t consider, you know, I don’t consider it my job to, you know, go in and answer every single question. If they hired me as their first person, I assume it’s because they want to build a data organisation. If they hired me as their first analyst to be able to do all the work, I’d probably question their judgement because it sounds like they could probably be better off getting a more, an individual contributor type person to come in and answer those questions.
[00:48:31] Bhav: But I have been in situations where I’ve been the only analyst. So I used to work for a travel company and in those instances, thankfully I overcome the fear. So if I go back to my definitions, when it comes to data, I walk without fear. And I was able to go in and do an analysis. So what we were trying to do was answer the question are our TV campaigns making a difference? I did not answer that question myself directly for a very long time. And when I did answer it, it was very basic.
[00:48:57] Bhav: So I wanted to use the tool, so I started learning R and understanding if I could use a seasonal decomposition analysis to break down my year over year trend. So like going back like the last five years. And then what I would do once I’ve taken out seasonality, once I’ve stripped away the trend, what I’m left with is the noise. And then what I would try to do is allocate that noise to when we were running TV campaigns. I showed this to a data scientist who, they were a partner of ours who used to do attribution modelling with. They were like, this is actually a data science method. This is how we would tackle it ourselves, or at least one of the ways. And I just did it, but it’s because I didn’t really have any fear.
[00:49:31] Bhav: So I guess it comes down to, I’m sorry I’m taking a long time to answer this question. In that instance, I was a data person, the only data person. I couldn’t answer the question, I wasn’t scared to answer the question. If I was a marketing person, would I go through the lengths I went to? Probably not. I probably wouldn’t consider it my role, nor would anyone expect me to be able to answer that question. However, they would probably go out and say look Bhav, can you go and find an answer to this question? If you need to work with external agencies, go work with them. If you need to work with consultants, go work with them. And in that instance, you’re not upskilling yourself, but you’re trying to find the solution. So, it doesn’t really answer your question.
[00:50:04] Dara: No, no, no. But I mean, I’m interested in something else as well there, because when I said earlier and I hadn’t considered what you then responded with, when you said basically the, you know, the analytics team within a bigger company is going to be a resource that’s very much in demand. So my natural thinking, and this obviously wouldn’t work in a big company, is if I wanted to be more data literate. And if I’m the stakeholder, I would just go to the people in the data team and say, hey, let me sit down with you and help me ask you better questions. But from what you’ve said, chances are they might say, go away, we’re really busy, you don’t get to sit here.
[00:50:37] Bhav: They wouldn’t, but at the same time, if someone wants to learn how to answer, like a really complicated question, like far here for me to turn them away, like for me, that’s great. Someone’s showing an interest in becoming a more data-rounded person. So I don’t, you know, I don’t want to discourage that. But at the same time, if I’ve got a million things to do and they are critical things, I’m probably just going say look, I’ll answer the question for you because I have to do all these other things. But let’s maybe put some time in the future where I can walk you through how I did it.
[00:51:05] Daniel: I’ll just close up by saying for me the idea of someone acknowledging or saying out loud that they’re not data literate or they don’t have an interest, is like the myth of the non-technical marketer. I think there’s the idea that we’re all technical nowadays. We all understand technology in a certain way and saying out loud, oh, I’m not a technical person, that’s inherently not true. I think everyone is technical, they might not admit it, they might not accept it, but I think we are, and I think everyone is to an extent, data literate. And I think there’s that next layer, it’s a precursor to a lot of other stuff.
[00:51:33] Daniel: You might not be venturing into that area of storytelling or the data communication, but I think everyone is data literate to some extent or another. And I don’t think anyone is going to start from scratch here. Truly unless I think they’re, you know, a kid at school or something like that. So I think, you know, everyone is data literate. You are data literate if you’re listening to this. And actually I think, you know, as Bhav was saying right at the beginning, it’s about slaying that dragon and not being scared of it, rather than actually having a skill set differential or something like that. So, there is no such thing as data illiterate people. I just think that’s the precursor to the more interesting stuff like curiosity and narrative and the communication side, which is a skill you can learn, develop, and depending on your organisation, might be part of it or might be something that’s driven from the top.
Outro
[00:52:16] Dara: That’s it for this week. To hear more from me and Dan on GA4 and other analytics related topics, all our previous episodes are available in our archive at measurelab.co.uk/podcast, or you can simply use whatever app you’re using right now to listen to this, to go back and listen to previous episodes.
[00:52:35] Daniel: And if you want to suggest a topic for something me and Dara should be talking about, or if you want to suggest a guest who we should be talking to there’s a Google form in the show notes that you can fill out and leave us a note. Or alternatively, you can just email us at podcast@measurelab.co.uk to get in touch with us both directly.
[00:52:51] Dara: Our theme is from Confidential. You can find a link to their music in the show notes. So on behalf of Dan and I, thanks for listening, see you next time.
Daniel Perry-Reed
Subscribe to our newsletter:
Further reading
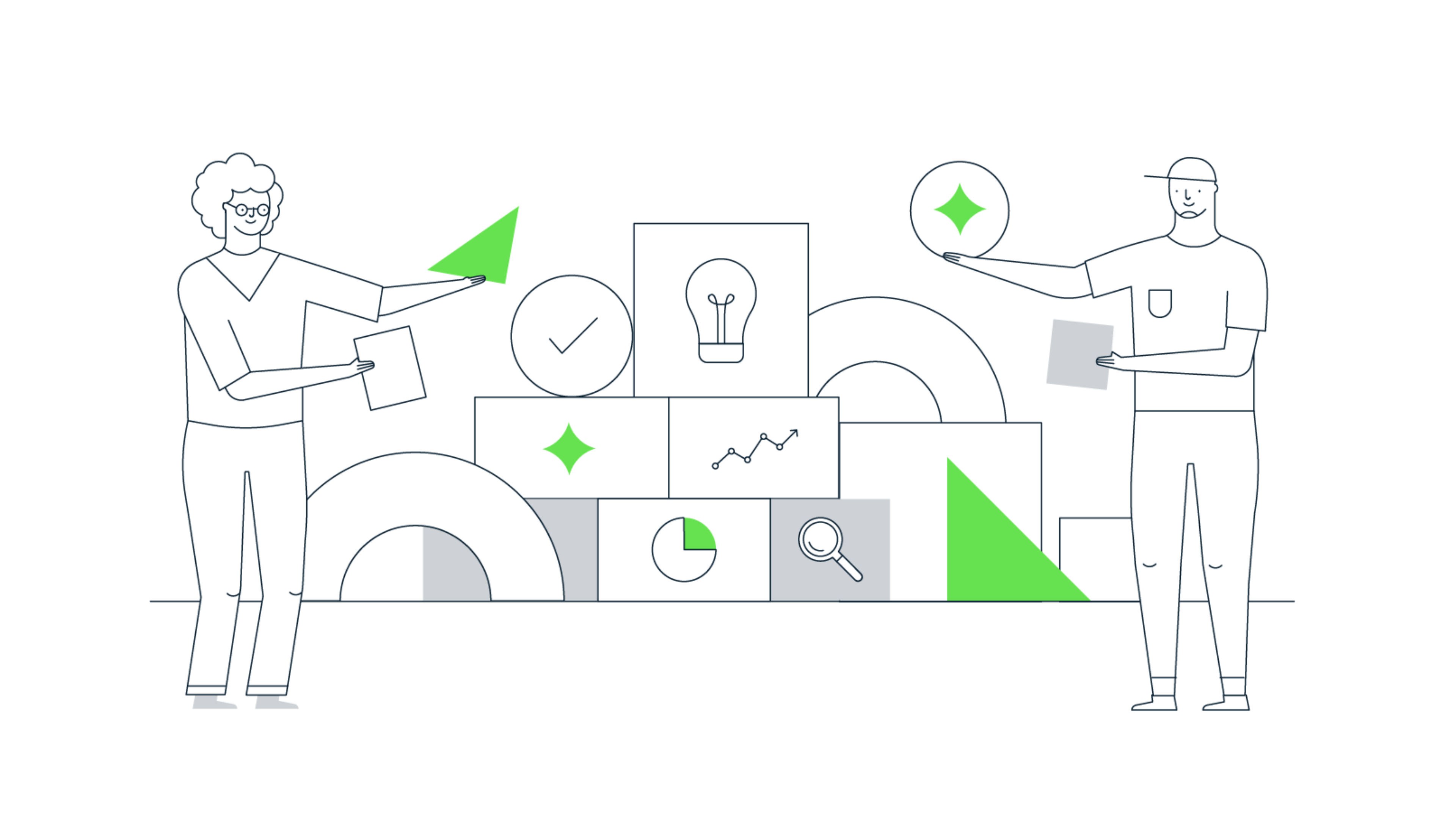
Easy ways to prepare your BigQuery warehouse for AI
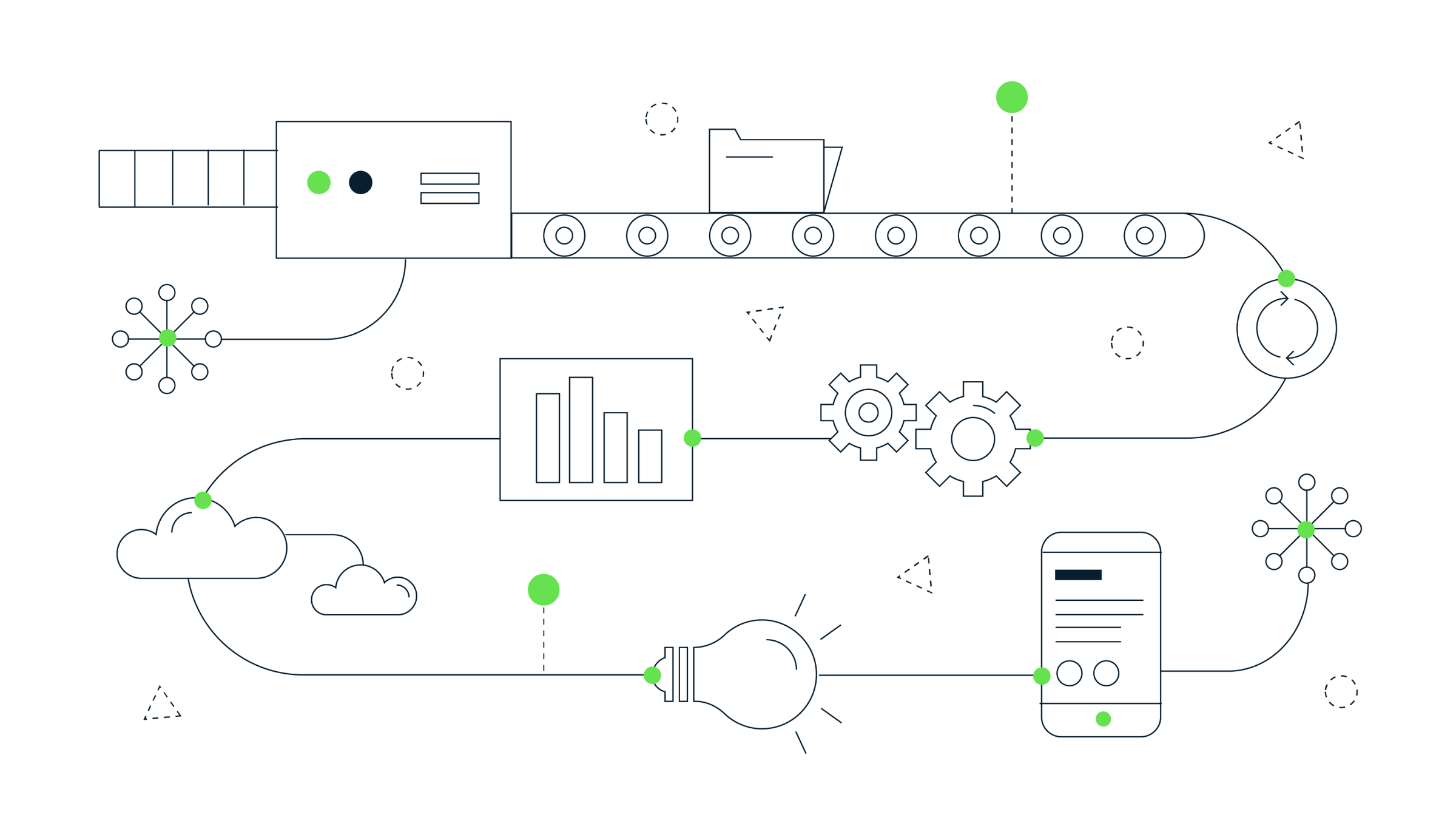
Data pipeline optimisation with Google Cloud and Dataform
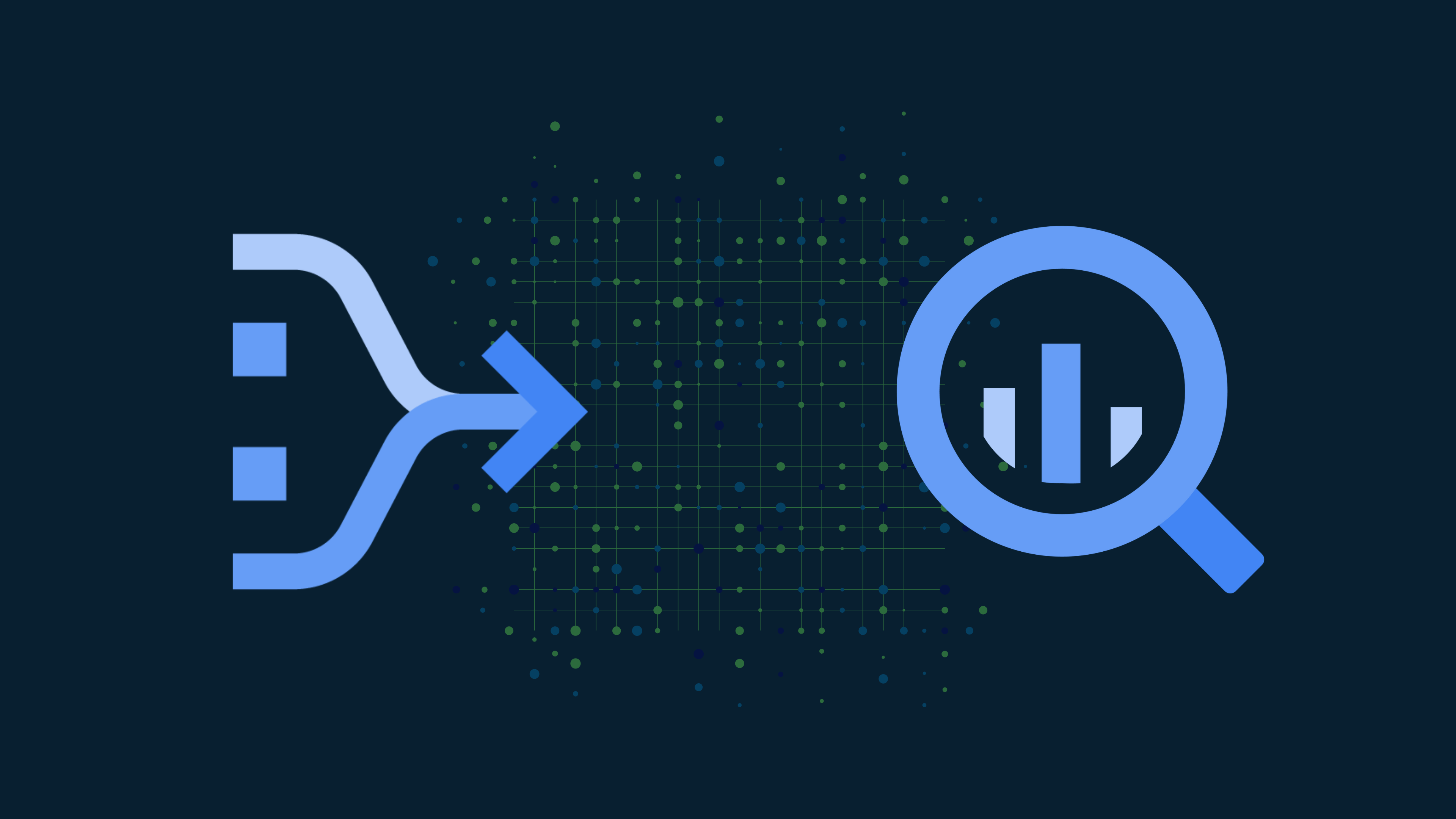