#88 Analytics as a data product (with Weiting Xu @ Cazoo)
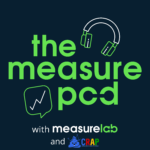
In this week’s episode, we dive into the fascinating world of analytics as a data product. Our guest, Weiting Xu, shares their insights and experiences as the head of data at Cazoo, a company that sells used cars online. We explore the challenges of treating analytics as a product, especially when it comes to managing internal users and their ever-changing needs. We discuss the importance of observability, the role of a product manager in the analytics space, and the need for agile ways of working.
Show note links:
- Connect with Weiting on LinkedIn.
- Check out Weiting’s CRAP Talk.
- Read the Forbes artilce on What Is A Data Product And What Are The Key Characteristics?
Follow Measurelab on LinkedIn and on Twitter/X, and join the CRAP Talks Slack community.
Find out when the next CRAP Talks event is happening on LinkedIn.
Music composed by Confidential – check out their lo-fi beats on Spotify.
Master Google Analytics 4 with Daniel Perry-Reed on the next GA4 Immersion 6-week cohort training course. Early bird, charity and group discounts availible!
Quotes of the Episode:
- “I think that there’s been a bit of a mindset shift in the analytics space to start to think about analytics [as a data product]. So like, what are the analytics products within the space and who are the consumers of those products?” – Weiting
- “This is where product thinking does start to show its strengths because you then can do things like stand ups and retros to understand what worked, what didn’t work, which typically you wouldn’t do if you weren’t operating in that sort of like product mindset.” – Bhav
- “There is a genuine risk of any change. Specifically when we’re coming to look at processes or frameworks, it’s just perceived as additional admin or a blocker for me doing my job or me doing the thing I want to do or have been doing, whether it’s efficient or not.” – Dan
Let us know what you think and fill out the Feedback Form, or email podcast@measurelab.co.uk to drop Dan, Dara and Bhav a message directly.
Transcript
The full transcript is below, or you can view it in a Google Doc.
Intro | Topic | Rapid fire
Intro
[00:00:15] Dara: Hey guys, really good show today. I’m finding these ones, I think I said this before, but I’m finding these ones really interesting. I’m loving learning more about product and analytics in the product space. So this is a former speaker at CRAP, Bhav, isn’t that right? I say, isn’t that right? I actually saw her speak and it was brilliant. I was there at the CRAP Talks. I might get our title wrong, but she’s head, head of data at Cazoo. And this is a really good chat with her about her experience.
[00:00:41] Bhav: Yeah, and you’re absolutely right. So Weiting was another former first time speaker at CRAP Talks. Super proud of that metric that I’m racking up first time speakers. If you are listening and you want to speak at an event, do reach out to me or Dan and we’ll try and get you on the lineup. But yeah, today’s chat was great. I really enjoyed talking about this subject. It’s a fairly new topic, and I don’t think it’s been very well covered in the analytics sphere. So it was kind of nice to have a different perspective on this, how they’re doing at Cazoo, hearing about some of the challenges that they’re facing.
[00:01:12] Bhav: And you know what? I think what made this conversation really human was that sometimes there just aren’t perfect answers to questions, right? It’s trial and error, like all the good things in life, right? You know, no one’s going to nail it the first time round. Hopefully all of, you know, everyone that’s doing it, writing about it, talking about it is leaving a trail for someone to pick up and, and follow and hopefully get us to that sort of utopian view where we are able to see data and analytics as a product. Or, you know, maybe we end up in a world where actually it’s not the right way to go, who knows? But, you know, it’s trail blazers like Weiting who are doing it and giving the rest of us something to think about.
[00:01:46] Daniel: For sure and I really liked this episode in particular because it feels like we’ve done every permutation of product and analytics with our time with you, Bhav. And I think this one we’re talking about analytics as a product rather than product analytics or marketing analytics specifically. I don’t even think we even clarify any type of analytics. It’s just data, data as a product. And I found a lot of real interesting value come out of the back of this conversation. So I’ve read about it and everything I’ve ever read about this subject, I’ll link into the show notes. If you’re listening and you want to go into the detail and everything will be listed over there, but especially from an idea of understanding the value that this can bring and understanding the changing the focus on technical delivery to a kind of business value, and it’s such a almost obvious when you say out loud, simple change of mindset, but it makes such an impactful difference in the way that data is perceived and the way the process behind delivery. And as you’ll find out, as we talk to Weiting she mentions that you know, fundamentally data as a product is just a framework.
[00:02:39] Daniel: Like it’s just a framework and it’s just something about experimenting with, seeing what works for your team, works for your organisation. And ultimately it’s just a better way of increasing the ROI or at least the value output of a data team or analytics team since there’s no secret sauce or magical ingredient here; it is purely a framework to adhere to. And I think anything like this, you know, like you said Bhav, it’s like the ground is quite, it’s not as trodden in this industry around analytics, and I’m really excited about, I’m already thinking about ways I can adopt it and integrate it into the work that I’m doing as a, as an analytics consultant. So It was really, truly valuable and I think I’m going to be thinking about this for some time, enjoy the show.
[00:03:14] Bhav: Hi all, today I’m going to welcome Weiting to the show. So I’m excited to introduce Weiting. Weiting is not just you know, another cool podcast guest that we’ve got joining us today, but she’s also a CRAP Talk, an ex CRAP Talk speaker. So very special relationship there for me. Weiting gave a talk on product analytics I think it was earlier this year. I seem to have lost track of time, it’s nice to have you, maybe you could kick off by just introducing yourself.
[00:03:37] Weiting: Thanks Bhav. Yeah so the talk that I gave, the CRAP talk that I gave was my very first one ever and that was really exciting for me. And since then I’ve just been on a roll of giving my opinion everywhere. So it’s really exciting to join you today on this podcast and, you know, give my opinion some more. So I am currently the head of data at a company called Cazoo, we sell used cars online. And I look after the data analytics teams and data science teams. Yeah, I’ve been working in analytics for about 14 years. I started at a little company called Zoopla, which some people might have heard of, and then proceeded to work at other companies like Shazam, Bumble and one called Patsnap was a, which was a B2B intellectual property company that didn’t have as much data as the B2C space, so that’s sort of back where I am now.
[00:04:32] Bhav: Exciting well, I mean, the background there of companies you’ve worked for, I’m sure most people have heard of them, with the exception of maybe that last one, but Zoopla, Cazoo, Bumble, these aren’t small companies by any stretch of the imagination. So welcome to the show, today I think we’re going to be discussing analytics as a data product, right? So I guess my first question just to kick us off would be, what do you mean by like, what do we mean by analytics as a data product? For anyone that’s not familiar with the concept, how would you go about defining it?
Topic
[00:05:02] Weiting: Data products is something that I’ve been thinking about a lot, and I think that it has different meanings to different people. So traditionally, or I think even in the last like several years, most people might consider that data products are things like machine learning algorithms, or data feeds like APIs, things where a team of engineers is producing data as an output for like other engineers or to monetize in particular.
[00:05:37] Weiting: So the consumers of those are usually like external to the company rather than to the team itself. And I think that there’s a bit of, been a bit of a mindset shift in the analytics space to start to think about analytics as a similar thing. So like, what are the analytics products within the space and who are the consumers of those products? And generally in my experience, when you work in a data team, and especially in my experience, working in companies that are startups or scale ups where the data team is serving the entire business, you get to work with all kinds of people and have all kinds of consumers and that expands I guess the portfolio of products that the analytics team is delivering.
[00:06:26] Weiting: So I would include things like the data model itself, for example, like if you have cubes or if you’re using dbt, however, the data is pieced together from all of its different sources. Things like the reports and dashboards that you’re creating and sending on to different members of the business, and also of course, the actual analysis that you’re doing. And that I guess also feeds into any kind of advanced analytics work, which ties in then very nicely to data science and that path towards algorithms, which were the original data products.
[00:06:59] Bhav: Okay so for the context of this conversation, we are ring fencing analytics as a data product within this internal user space. Because one of my questions was going to be, in the past, when I’ve come across any form of data product, there are usually two avenues. There is the external users and there are the internal users. The internal users being like, so stakeholders and external users are going to be, I used to work for a B2B product company and we had data products that we would, it would be part of our customers package where they could access their data and they could see how their customers were doing.
[00:07:31] Bhav: So it was very much something that we were able to monetize to some extent, but you know, in its most infant mode or something that we allowed customers could see. They could get, you know, we would give them a view that would allow them to see with their data, their customers data within a nice interface and that was what we were referring to. But here we’re now talking about using analytics as an internal, as a data product for internal users, right? So what are the main challenges of internal users and external users? Because it’s, you know, for external users it’s done, you know, we know how to do product for external users, but how do you create a product for internal users, specifically something as complex as data?
[00:08:07] Weiting: Yeah, that’s definitely something that I’ve been struggling with. And for me, one of the big challenges is that the landscape of internal users is changing all the time. So when you do have external users, you make a product and then you basically can never change it because people come to expect that. And then they rely on you, whereas internally, the set of people can be different from week to week or the circumstances around what you’re doing can immediately change. And I think that trying to keep up with that change management is probably one of the biggest challenges. And so with that, I guess there’s a broader question about change management and how you deal with that. And I think that there are some pretty standard answers there around like the relationships that you build with people and how you do prioritization.
[00:08:55] Weiting: But one of the main things is actually you can use data to solve your problems about data. And so starting from a place of observability. So I think one thing that I found recently was an article that you’d written Bhav, like some time ago about using some sort of Jira plugin to get observability about the kinds of work that you were doing. So that is currently something that I’m thinking about is just sort of to understand with our teams, what kinds of work that we’re doing for who, and does that actually match up to like the greater needs of the business.
[00:09:25] Bhav: I think you’re referring to the actionable agile, right? For anyone that isn’t aware, actionable agile is a feature within Jira that allows you to see things like how long tickets are in certain states, how fast you’re closing them, which of the analysts are closing them fastest. And it really allows you to bring a data-driven approach to like your agile process.
[00:09:45] Weiting: Yeah, exactly. So for us one of the things that we spend a lot of time doing as the messengers of the things that are happening around the business is that we end up having to fix bugs and bad data. And a lot of the time, bad data or I guess bad data quality is the outcome of having like poorly designed processes or yeah, just like lack of communication and bad process. And we are the ones who accumulate and aggregate all of the data together and then surface the reports where other people are spotting these things happening. So we spend a lot of time drilling into it, trying to understand what the source is, and then bringing it back to the different people to bring them together in order to solve those problems. And so one of the questions that I have is like, how much time do we actually spend doing this?
[00:10:35] Weiting: What is the percentage of our work that is this? And how can we then say, hey, is this really where we’re delivering the most value if we’re spending like, you know, 30 or 40 percent of our team’s time working on this, can we perhaps work together a bit more closely or in a different way so that we can possibly shift that problem or like understand the problem a little bit earlier on and solve it a bit earlier so that we can then spend our time actually like collecting good data and then doing analysis to, you know, identify new opportunities for the business or something like that.
[00:11:10] Daniel: So I have a, I have a question Weiting, actually I’ve got two, so I’ll throw both of them at you and you can decide where to start. So the first one is when you’re working at a company, maybe the size of Cazoo or some of the other ones you mentioned, and there’s a data team and there’s a lot of people working on that. And I suppose the end users, there’s numerous and they change. How do you organize data products? How do you keep an eye on what you have, where they are, who’s using them? So that’s the one thing in terms of like just management of the data products, once you have the data products. And the other question I had, and maybe all the way back to the beginning of the episode is really just like, what’s really the difference between maybe building a model versus building a model as a data product.
[00:11:44] Daniel: Like what are the kind of structural fundamental differences in terms of just doing the work versus the kind of the productization of this. I’m really keen to get from you like where that difference is and why we’re using this label products now when maybe we think that we’re kind of doing it already. I don’t know in the way I’m thinking about it, it’s like I do data products. I’ve never called it that, but maybe I haven’t, maybe it’s something different.
[00:12:05] Weiting: Yeah, I think those are both good questions. Kind of an answer to your first one, which is about how do you organise, I guess, categorise your products. I guess the learning for me, having worked at Cazoo and it’s like incredibly high growth journey is that you don’t until you realise that you have so much that nobody knows what’s going on. And then you actually have to think a little bit more critically about the problem. So we expanded really quickly as a company, and I think consequently the way that we worked had to change a lot because when you have 20 people and when you have 50 people, and then suddenly when you have 500 people, the needs that you’re filling are very different, right?
[00:12:52] Weiting: And Immediately in any job that I’ve worked in, I’ve done stuff and seen how legacy is created because you don’t know what the future is going to look like. So I think the project that we are going through now is trying to think about how to make things a little bit more organised or accessible. I think there’s a whole other conversation about self service, which we will very happily ignore it within the scope of this, but it feels like the thing that makes something a product and in particular, like a model or a data product is how you approach the change management with respect to it. So understanding when changes are requested, like against a certain data model, let’s say that you have like your core revenue model and your stakeholders need to like have additional revenue metrics, understanding what the impact of that is going to be and having a process where when you do it, it’s tested, it’s changed. So really I think leveraging some of the cycles of like the software development process, I think that is for me the difference rather than just like building things ad hoc and this like stack of, or house of cards.
[00:14:01] Bhav: Weiting, you mentioned and I guess this kind of ties into the, when the company goes from like 50 to a hundred, 500 people your stakeholders change, things like that. But I guess people’s expectations change as well, right? So as part of this change management, people’s expectations of what they want from you suddenly starts to grow as you deliver more. How do you manage people’s expectations growing? Because when you’ve got data products for external users, It’s fairly straightforward. You kind of like, this is what you get to some extent, you may have a backlog and it’s fairly easy to manage because you don’t have a direct line of contact with external users, but when you’ve got internal users who are potentially breathing down your neck, you’re trying to, you know, adopt software engineering methodologies in creating an environment where you can look at data as a product.
[00:14:45] Bhav: You know, in theory it’s great. In my experience people always want more and they don’t always want to follow the processes and they don’t always want to use the systems that you’ve built and the processes you’ve built and the frameworks you’ve built to do that. How do you manage people’s expectations?
[00:15:00] Weiting: I would say that early on, I was quite fortunate because when you work in product analytics and you work with other people who also do product. They are all happy to, you know, go through the same change that you are, or to follow all of those ways of working because essentially that’s their ways of working, right? We’ve just stolen all of it from software engineering and product development. And so I think initially being able to work with that kind of group of people helps me to be able to set up good patterns or ways of working or communication.
[00:15:33] Weiting: And I think as you work with different types of people so like within Cazoo, for example, there are two parts of the business There’s like the ecommerce online sales part of the business and then there’s like all the stuff that we’re actually still doing with the cars and like, you know, we’re moving them through the production cycle or whatever. And that side seems like almost like a different kind of digital transformation. And I guess it’s a balance between understanding, like here are these people who are doing these things that, you know, they have a lot of industry expertise and they have a lot of knowledge and just trying to learn from them rather than push too many changes on them at once versus being like oh, here are like finding ways that you can say, well, like if we work together on this, there are small ways that we can help you and just getting their buy in really.
[00:16:26] Bhav: Okay do you get like people who are just like really averse to this kind of software engineering agile way of working, you know, whatever you want to call it, because they’re so used to I want this, therefore I must have it now.
[00:16:37] Weiting: Yeah, and to some degree, I think that the team, as a team, we are like still doing that. Maybe not now, but like, oh, quite soon we will do this, but we are also doing this and this. And it just goes back for me to that observability, because if you can show what you’re working on, then you can give transparency to everybody and then force them to prioritise things against each other, right? So it’s like, do you want this report or do you want this piece of analysis, which is actually possibly going to deliver more value and some of it is just down to the maturity as well, and I don’t know if you found this, but when you are still in like a digital transformation space, working on actually having visibility of all of your data or being able to tie pieces together, then the needs for analysis are like a little bit different because what’s the point of doing analysis if you can’t even trust like the reports that you have or the data that you have right, so it’s just stages.
[00:17:33] Daniel: The trust is a huge part, right? Because as soon as that’s burned, that bridge is burned. It’s really hard to rebuild that even if it’s all fixed. There’s this kind of legacy kind of like, oh, that was that one time that it was broken or there’s this bug or something like that. You mentioned about the maturity and I think that’s a really, it’s a really interesting part whenever it comes to anything to do with analytics or data or products even, and it’s this idea of like, is it a company size or vertical or industry, or is it a maturity thing? So like, for example, let’s talk specifically about data products. Let’s say you’re a solo analyst, let’s say you’re, you know, you’re even a part time. For a company of that size, is it worth productizing the data products? Or is this something that you kind of grow into when you have the capacity for a team of people to manage that process?
[00:18:12] Daniel: Or is it more of a maturity thing that you mentioned? Because I think it’s always hard to detach those two ideas, because often we think bigger organisations are more mature and naturally they’ll go into that, but I know that there might be a difference. I’d love to get what you think about that.
[00:18:24] Weiting: So I guess just to take your example and play it out, say that you have a, you know, a large company out there and everything that they have is like still in Excel, possibly at that point, the idea of productizing data looks a little bit more like it would with an external set of consumers, which is like we have this set of reports, and these are the changes that we’re able to make to them. And there’s not really as much opportunity in that sort of situation to be agile or to do risky things or like make big changes I would imagine. So I guess I wonder too, if it’s got something to do with like how centralised data is as a function or like what your operational model is.
[00:19:12] Dara: I’m wondering, so aside from the data maturity of the company, what if it’s a company that’s just not used to product thinking? What if that’s just not something that they have experience of, but maybe their data could be, you know, they could be a bit more mature in terms of using data, would it still work to try and introduce this kind of concept of treating data as a product? Or would there be challenges if the business itself is less familiar with kind of product management principles?
[00:19:39] Weiting: I think that it would probably still work because ultimately, these are just frameworks, right, for how to make decisions and take actions. And you don’t have to be like follow anything exactly. I think different situations call for different variations of it, but if you wanted to use different language to mask it or like make it more convenient for the people who were not necessarily used to it, that would be another alternative. They could make up their whole own vocabulary and feel like they had ownership over it, for example right. At the end of the day, it’s just a set of guidelines for how you might try to like deliver a different kind of value, I guess. Have you ever seen it just like not work at all?
[00:20:25] Bhav: I think on this, it’s a difficult one, because I think in order for a company to really adopt data as a product, let’s say analytics as a data product, the company really needs to understand and embrace agile ways of working, right? I genuinely don’t think that you can shoehorn one, you know, certainly something like data, which isn’t typically thought of as product into a company, which hasn’t fully immersed itself into product thinking. And I think that’s, I think that’s a challenging part, but any company that has immersed itself or is on the path to immersing itself into a product way of thinking and adopting software engineering best practices or product best practices.
[00:21:06] Bhav: I think they’re more likely to succeed regardless of the size, because it just means that you have another team that you have to work with in a way that, you know, is going to get the best outcome or the best output in the leanest way possible, right? So I think where my, some of my challenges come up with when thinking about data products is if you think about data engineering, data engineering, and typically work in a product way anyway, right? Like, I don’t think data engineering, unless you go back like 15 years when data engineers were more like data managers and they didn’t have those things. They were just some team who would be in the background somewhere. But I think most modern day data teams really have adopted like product thinking.
[00:21:48] Bhav: And their consumers are usually the analysts because the analyst will be like, oh, this is what we need. These are the models we need, and the analyst becomes then the gateway between the data and the business. When you start thinking about analytics as a data product or as a product, where some of the challenges for me might come up are you’re really, you know, and Weiting I’d love to hear from you, you’re putting in barriers in place by introducing, say, for example, a product manager, an analytics data product manager, who has to be the person who then collects the feedback, collects requirements, manages the roadmap, manages priorities before, you know, before prioritising something. And I guess my question on this would be, can that succeed in an organisation that’s used to being a bit more sort of like, well, I’m going to just go speak to the analysts.
[00:22:34] Weiting: Yeah, I’m super interested in this as well, because I think it all sort of goes back to like, what is your operational model? And especially because we use dbt, so now there’s this whole other layer of analytics engineering. And is that like a capability? Is that like within a regular analytics team? Is it its own team? How does that interface between all of the different areas? Who then owns for example, like the data model? If that’s a data product, who owns that? And the answer is like, I don’t know. It just really depends I think again, there’s never like a good answer, but I can imagine that in many companies, there will always be people who will chafe against working in a product way. That exists within like product and engineering teams as well right.
[00:23:24] Weiting: And for example, that’s why those areas often have like support functions. So there is a whole team that’s dedicated to support. So if you just play that through to its natural conclusion, imagine if you had a whole team of like people who just did data support, and that was a way to get into data as like a intermediary job. Would that work? Possibly, it might be like a really interesting experiment, I think. So I think that there are, if you could do experiments with teams more easily, and it wasn’t subject to the confines of like budgetary constraints and hiring, and you know, like actual people’s lives, it would be really fun to play around with different configurations, but there’s not necessarily a great way of getting all of that different experience without having jobs a lot, and that prevents you from really being able to like get embedded into a company and, you know, seeing things through either so, yeah.
[00:24:17] Bhav: This is where product thinking does start to show its strengths because you then can do things like stand ups and retros to understand what worked, what didn’t work, which typically you wouldn’t do if you weren’t operating in that sort of like product mindset.
[00:24:29] Daniel: I think the bit that comes before this is about kind of explaining and selling the value of the product way of thinking, because there is a genuine risk of any change. But specifically when we’re coming to look at processes or frameworks, it’s just perceived as additional admin or a blocker for me doing my job or me doing the thing I want to do or have been doing, whether it’s efficient or not.
[00:24:51] Daniel: So I think this is the key thing is like if we’re looking at kind of productizing analytics, it’s like, it still feels pretty new within the analytics space. And I think actually it’s trying to kind of convince, explain, and adopt that outside of the team as well. Making sure that this is a, this is a good thing and it’s going to be more helpful before it’s just perceived or maybe irreparably perceived as an admin exercise.
[00:25:12] Weiting: I think you have to be adaptable within the team as well and just do things and see what lands and what doesn’t, because it’s not like you have to be rigid about it, right? Because data is very different at the end of the day. And I think it is quite different from like other products and that people don’t feel that they have opinions or expertise in data. I guess that’s different, it’s like when you work in data, you are always the expert in what you’re doing. Whereas oftentimes if you work within like a product environment and are trying to encourage a product way of thinking with commercial people, they are still feeling like they’re the experts, right? So there’s like the whole conversation and products about you need to be an expert in the domain or not in data. You always have to be the expert in the domain and possibly that gives you like an added layer of, it just adds an extra layer of complexity, I think.
[00:26:05] Daniel: Okay sorry, Bhav I know you was going to go take us off onto a different subject, you know, especially people listening and myself working with different organisations, I think you walk in somewhere and they often, or sometimes might not have that kind of like always experiment culture to begin with, to be able to try this kind of stuff new and actually maybe the groundwork to kind of, to think about this in a certain way is actually an important, maybe overlooked step in, in this whole process before it falls flat on its face. I’ve seen new systems and processes introduced and without that kind of work, legwork up front, actually, it can be really difficult, especially if they don’t have that kind of mindset to begin with, anyway sorry, go on Bhav over to you.
[00:26:39] Bhav: I think it’s an important point. I think, you know, when you are introducing new things, it does take a while and sometimes it does feel like pushing a big boulder uphill, but hopefully you reach that point when everyone can see the benefits of it and it’s reached the top of that mountain it’s all suddenly downhill from there, which is downhill in the good sense from there, not downhill in the bad sense. So what I was going to slightly pivot to is, is the role of the product manager within this concept of analytics as a data product. Who plays that? Do you feel like you need to hire a product manager, a data product manager, or do your analysts take on the role of a product manager? Like, how do you do that?
[00:27:16] Weiting: Yeah, it’s interesting because when you are a data manager, so somebody who is like, you know, managing other people within a team or having direct reports or working directly, you know, with stakeholders, I think sometimes you have to fill all of the roles and it depends on, especially within like a smaller organisation, you have to wear all of the hats. You have to play the role of that product manager and then you have to do all of the HR stuff as well. Or like, you know, sometimes all of the individual contributor work still. And so it just really, yeah, nobody really equips you for that as you progress throughout your career, I would say and it’s very eyeopening.
[00:27:56] Weiting: We briefly had a product manager who was supporting the data team who helped us with Dan what you called some of the admin stuff because actually once you get on top of the admin stuff, it kind of just runs itself. So like we do a backlog review once a month and that’s fine now but it was so painful to get set up and having that person who is specifically there just to do that for us and tell us here’s how you need to do things makes a huge difference. And so, you know, before we had that, we just had this like list of like hundreds of old tickets from requests that people have made. And now when you look at it, it’s broken down by quarter, it’s really nice, you can prioritise things easily, see where people’s requests have gotten to.
[00:28:41] Weiting: And it takes maybe 20 minutes a month to maintain it’s fantastic. What I do think is interesting is within the analytics as a data product space, I don’t know what the role for a product manager there really looks like, because there’s not, I guess this idea of like developing a product, so like this discovery piece and like making something which is going out there to end consumers isn’t necessarily the same as if you worked within like a software or any other product space, and it might not attract the same kinds of people or attract people for a different reason. So I don’t know who would fill that space I guess, that’s the question. It’s like, yeah, in that world, who is an analytics product manager and what are they doing?
[00:29:28] Bhav: And this is something I’ve wrestled with personally myself when I was at Gousto, when that article you referenced earlier, I wrote that at Gousto, when I was working at Gousto. One of the the things about thinking about analytics as a product is that when, you know, when you think about, say, for example, data engineering, which is to some extent, just another extension of software engineering, there is a tangible product output, there’s something you are building, that’s going to require feedback. And, you know, it’s probably going to take you a month or two months, whatever, and you’re going to build it and you know, that’s all you’re going to work on. When you’re dealing with analytics, the feedback loops, and the cycles, the work cycles are so short, that it’s kind of like there isn’t time for discoverability in that sense. And actually what I found was that I had to go and adopt the, sorry, I had to go and request the time of an agile coach to coach me in how best to shape my team and how best to get, you know, the most out of us.
[00:30:18] Bhav: And it sounds like you did something similar, except you, you know, you had a product manager who coached you to do this. We had an agile coach, they were the ones who walked me through Jira best practices, how to set up the, you know, the perfect Jura board and how to use actionable agile and all those things. So I think it makes sense it’s difficult to hire for that role as a product manager. Ideally, what you’re trying to do is make sure everyone’s working in that sort of like that product way where they are regularly grooming their backlog, they’re regularly running retros, they’re regularly getting feedback in a, you know, in a timely manner, as opposed to having a sit in awaiting feedback in the Jira column for like four or five weeks, right?
[00:30:54] Bhav: We’ve all been there, we all know what it looks like. So do you then, if in the absence of a product manager, do you adopt sprint cycles? So do you work within you know one week, two weeks sprint cycles to ensure that you are still kind of like using this software engineering mindset to rapidly race through as much work as you possibly can and close off things that you, you know, that are constantly being opened.
[00:31:17] Weiting: I would say that we work in more of a kanban way more than anything, they’re not strict sprint cycles, but what we do have is just like a sync up every week to make sure that the things that we are doing are the right things for a company that is changing direction very quickly. Our team is small enough that we are able to do that. I don’t think that that would necessarily scale. And if we were in a larger data team, you might have to think about a different way of doing it. But we’re like 12 people at the end of the day right now.
[00:31:51] Bhav: Some people argue 12 is a good size analytics team. My last team was about six analysts. So i’m listening to 12 like, oh, that’s you know, that’s a lot of analysts. I think on your point yeah, I mean I still think you can adopt you know, like working with sprint cycles in a kanban way, right? Like for example, you do your best to size up each ticket every week and anything that is quite big, you then turn into a story or what do they call it in Jira? I’ve forgotten what they call them, epics thank you, yeah epics. Yeah and then you break it down so that you’re still operating in a sprint way. I don’t know maybe this is just something for you to think about and ensure that you are as a team moving in a operationally more efficient manner.
[00:32:31] Weiting: Yeah, I think so. I guess the interesting part of it for me is that I possibly, like yourself, never actually have like gotten any sort of formal training in product. All I know about it is through working in product analytics with product teams. So you kind of just absorb it through osmosis and then say, hey, this little bit is interesting. I want to like try to adopt this, or actually that sounded really cool. Or like let’s avoid that, I think it’s really silly or whatever. So there are aspects of the product teams where they are doing like all of the simulations and like really trying to plan ahead, which seems so pointless because as an analytics team, anything could change at any minute. And so like, there is no point in looking too far forward into the future, I guess. I think the expectations are a little bit different for how you are going to deliver things.
[00:33:23] Dara: If you’re an analytics team lead and you’re listening to this podcast, now you’ve both kind of mentioned coaching, or at least Bhav, you mentioned coaching and Weiting you said that you had somebody in filling that product manager role briefly, who kind of helped get things on track. If you’re listening to this and you’re leading an analytics team, you’re not currently following kind of product thinking, what skills do you need or where would you start? What needs to change within your team and what would kind of that sequence be like, what’s the first, most important thing you’d need to do to kind of upscale and then what else might you need to do after that to kind of make sure the team is is up to speed.
[00:34:01] Weiting: I actually really rate Bhav’s. I don’t know if it was a suggestion, but it should be of actually working with an agile coach. So if you work in a sort of product forward business, then probably there will be an agile coach within the business and they will be happy to help you because they are so excited to help teams work better and more effectively. So like a hundred percent reach out to them and to talk them through your problem and get them to help you identify like which areas you could improve in or just figure out where to start like It’s such a great resource and I think that, that was something although we did have a product manager that was something that I also did a lot of was to like relentlessly hound the agile coaches with questions about how to do this or like I’ve never run a retro before what do I do? And yeah, they’re just really, they’re always happy to help.
[00:34:50] Bhav: Yeah 100 percent and certainly at Gousto before I adopted I didn’t really call it data products or analysis of data product. But although in hindsight, I guess it was like, now that I think about what I was trying to move towards, I kind of went through sort of like a four to five week extensive training every week with an agile coach, where we discussed everything from setting up Jura boards, to reporting, to you know, creating psychological safety nets for your teams. I’ve fully immersed myself into that kind of agile way of thinking before I made this change, because I think it’s easy to kind of like shoot from the hip and just say, hey, we’re going to be agile now. But what does that mean? And I think if you’re an analytics leader and you’re thinking about this, it’s worth doing the due diligence needed up front to really understand what you’re about to get yourself into.
[00:35:35] Bhav: Even like, you know, Weiting you mentioned right at the front observability is important. If you are about to embark on a journey where you’re going to go down, you know, analytics or data as a product, you really need to be able to have some type of measurability in place to understand where you were to where you’re, you know, where you want to get to, I think without it’s not going to succeed. I think going to an agile coach and throwing yourself into this product thinking way is going to be a good starting point.
[00:35:59] Bhav: Weising, I think, you know, obviously we’re talking about product here in the product sense, sorry in a data sense that when you are dealing with products, there’s always going to be that concept of user experience. What does a good user experience look like for your stakeholders who are working with you as a product team? That’s my challenging question.
[00:36:18] Weiting: I love that, what it really makes me think of is when I worked at Zoopla, my first boss had something that he called the found at the printer test, which is imagine that you were to print out your output of whatever, if it was a presentation or like a report or, I don’t know if it necessarily works for a data model in quite the same way, but let’s just extend this analogy, like, would the person who picked it up with absolutely no context be able to have some idea of what was happening. And he used to drill this into me, just over and over again. But it basically boils down to a couple things, which is documentation, so have you labelled all of your axes correctly? Or you know, in the case of a data model, do you have documentation for your metrics or whatever? Is it easy to find? Simplicity as well like, is it straightforward and easy to read if there’s a trend? Is it clear that that’s what you’re showing using like the appropriate visualisation? And I feel like those are the sorts of things that contribute towards a positive user experience.
[00:37:27] Bhav: Do you have a way to measure it? Like, do you have success criteria? Like, as a team, how do you measure the success of your output, or do you measure it via the outcomes and the decisions that are being made? Yeah, I think that outcomes is a good one. So I think that we’ve built a lot of dashboards, which three people have looked at about five times and then have never been revisited. And, you know, you can get reporting on that sort of thing now, so there is a lot of misses as well. But then, you know, you only need to have like an occasional success to keep the morale going. And we’ve had, you know, like several we’ve consistently had great examples where we’ve, you know, helped to inform a really important decision or helped people to, you know, implement cost savings initiatives over time. And I think that although within this like data as a product space, ultimately we are partners of these other teams. And so our success is their success and vice versa. Like we’re working together and like that collaboration aspect is always going to be so important.
[00:38:28] Dara: You mentioned other teams and I meant to ask you this earlier as well, because when you, when you were talking about your experience, you were talking about being in a kind of centralised data team. And I feel like maybe you’ve answered this through the conversation, but I’m just wondering if it would change anything, if it was a very large organisation that had multiple different data teams. So, you know, let’s say they had like a customer insights team and a marketing analytics team at a product analytics team. I’m guessing there would be extra hurdles to get over, but would it be best to try and get all of those data teams treating, you know, following a kind of product thinking approach, or could it still work if just one of them was?
[00:39:05] Weiting: Yeah, not necessarily, I guess and maybe Bhav has alluded to this as well. There are certain streams of data work, which are more amenable to a product being productized than others. Like at the end of the day, you will always have like separate streams where you have to like fix those bugs or like make some small changes to the dashboards or that’s probably not a great example but like I think that you can have both at the same time or like try to adopt different kinds of product solutions or best practices or ways of working to the different kinds of work that you’re doing.
[00:39:45] Weiting: So I guess one example for us was we did like a big customer segmentation piece where we actually had the product manager who’s working with us at the time, like to go around and do a discovery. And he talked to all of the stakeholders and tried to understand what their needs were and what they expected the output to be and that was like a huge success for example.
[00:40:04] Daniel: So who cracks that whip now? Like who who’s doing that now that you, the kind of the borrowed time of this product manager has now gone you’ve got this kind of relatively well oiled machine kind of self kind of governing and running. So what I understand in terms of data products, the big difference there is that it’s centred around business problems and solving directly business problems or addressing direct kind of connecting the solution to revenue or something like that.
[00:40:27] Daniel: So who’s doing that now when these are all skills that you had within that product manager, is that still happening? Is this something that another one of those upscaling things that we all collectively as analysts and data scientists have to do ourselves, or is there like a, a head of team, or is that your responsibility waiting in that case to make sure that it’s hitting the nail on the head for the rest of the business that kind of need to use these products.
[00:40:47] Weiting: Yeah, I would say that there was definitely an upscaling piece whereby all of the lead analysts in the team, like really learned a lot from that and are able to drive it forwards themselves. Not everybody wants to work in that way either but it does feel like in a modern data team, especially as you progress in seniority, there is a lot of those skills become increasingly valuable, I think, and I think can be directly tied to progression as well, right? So, as you know, analysts who are listening to this right now, I would say just throw yourself in the deep end and, like, definitely upskill yourself in those areas as much as possible because it absolutely pays off.
[00:41:29] Bhav: I think a large part of this concept of moving analytics, you know, from just traditional ways of working to a more product to productizing it, does require a development of more professional skills or soft skills, whatever you want to call it, I don’t like the term soft skills, so I say professional skills. And it kind of brings me to the battle of the hearts, product managers love saying no. Analysts don’t know how to say no, right? So analysts love saying yes. And this is a bit more of a fun question, but how do you kind of merge this world of product managers who are just like, no, we’re not going to build that. No, put it on the backlog. No, we’re going to car park it, whatever ridiculous product terms, you know, come out in that week versus the analysts who are so friendly and super helpful and really want to do everything and answer everyone’s questions and make the world a better place and sing kumbaya with everyone. How do you merge those two mindsets?
[00:42:20] Daniel: You only temporarily have a product manager, then you get rid of them. And so everyone says yes all the time.
[00:42:26] Bhav: Oh my God, what a horrible solution.
[00:42:28] Weiting: I try to encourage the analytics team to fight into the delight and saying no also, so that they can help people and say no with a smile and then feel really happy about it.
[00:42:39] Bhav: It’s a tough one. I mean, by the way, that was supposed to be a difficult question on purpose. Every now and then like throwing those ones. And I guess this is where you would have, you know, product manager you know, playing that role where if someone does go to an analyst and say, hey, could you just do this for me? You could just bat that person and say, oh, can you go speak to my PM? And they’ll prioritise, but in the absence of a PM, it’s around this, as you said, we do need to develop some skills in being able to like, you know, push back and find delight in saying no. So, okay I’ve got two last questions, my first question is why should businesses consider productizing analytics?
[00:43:14] Weiting: So that they’re getting the most ROI for their analytics teams. I think that it’s tough with analytics because so many analyses that you do can actually just be little rabbit holes and dead ends. But the opportunity is just huge, isn’t it? Like, when you do actually find a really good insight or unearth something, then you can make the business a lot of money basically. And it’s the same I guess that is the same with product is that you have to take risks and take a lot of them in order to find the payoff. And so really it’s just down to like that risk reward balance.
[00:43:53] Bhav: So it’s kind of like thinking about monetization of your analytics as a product. Because you would do that as a product manager anyway, you do that as a marketing person. Why not as an analyst, like what is the ROI on this? What value is this going to drive? So having that mindset of what value is the work I’m about to do going to add to the business, so that is why. Okay, brilliant. And my, I guess my second just follow up question is, if you are about to embark on that journey of productizing analytics and data, what are some common mistakes to watch out for?
[00:44:21] Weiting: Trying to do everything at once, probably. Yeah, I’m not sure I’ll put that one back on you. What were some mistakes that you made that possibly I am still making now?
[00:44:31] Bhav: I don’t make mistakes. It’s a tough one because I think when I adopted agile analytics and tried to productize it, I used my team as a testing ground and then I shared the results with my other sort of like peers who are leading analytics teams. And by doing so, they were able to see what progress we made, how much more efficient we became. And that was a great way to get them to start thinking about this. Because I think Dan asked earlier, when you have got multiple analytics teams, how do you get them to start working this product way? So I think my watch out would be if you’re going to go down this route and there are multiple analytics teams in your organisation. Try to solve for everyone, not just for yourself. And that’s something I’ve always lived and breathed by, if you’re only solving your own problems, you’re probably missing out on solving problems across the board.
[00:45:22] Bhav: So that would be mine. Like, you know, if you are going to go down this, think about your organisation or think about the analytics organisation, what the structure looks like, and can you deploy this sort of like mindset, this methodology, this framework, whatever you want to call it across the board. Don’t just solve it in isolation of just your own team, think about how this might solve problems across the board. So that’s, that would be my common mistake to potentially watch out for.
[00:45:44] Weiting: I’ve thought of one as well, which is take meeting notes so that you actually action the things that you say that you’re going to do. Otherwise, what’s the point of any of it? That was a mistake that we made for a while before we thought, you know, we could just write this down and make our lives a lot easier.
Rapid fire
[00:46:00] Dara: Okay, just five more rapid fire questions, and then you’re off the hook Weiting and this has been a really, really great conversation, really enjoyed it. So, question number one, what is the biggest challenge today that you think will be gone in five years time?
[00:46:15] Weiting: Ah, I’m so excited about this, is GA4 migrations, because everybody will either be off of it or have already done it and then we won’t have to think about it.
[00:46:28] Daniel: We’ll be on to GA5 by then. Oh, maybe I’m giving you the answer to the next question. Jumping the gun. So what will be the biggest challenge in five years time?
[00:46:36] Weiting: This is like a really hype answer I think, but I have so many misgivings about everything that’s happening in the world of AI in particular with respect to like diversity and inclusion, because ultimately it’s only as good as the data that we put into it, which is not very good in the first place. And what kinds of problems is that going to amplify in the world? And that really scares me.
[00:46:59] Dara: Okay, of all the money myths out there, what’s one myth that you’d really like to bust?
[00:47:05] Weiting: Maybe that everybody should be doing AI? Get the basics right first, possibly?
[00:47:11] Dara: Kind of similar question, if you could wave a magic wand and make everybody know one thing, what would that one thing be?
[00:47:17] Weiting: Okay, this is really not related to anything, but you know like hair grips? That women use to put your hair in. Apparently, you’re supposed to do the wavy side down so that it actually sticks. And I’ve been doing that wrong and like upside down my entire life and only realised this like in the last six months. So that’s amazing, basically.
[00:47:40] Bhav: I mean, it’s not a problem I’ve ever had to deal with.
[00:47:45] Weiting: Well, you know, if you have daughters in your lives one day, or like granddaughters or something, and you have to plait their hair, now you’ll know.
[00:47:54] Bhav: I mean, I’ve got two boys. So thankfully, it’s not something I have to worry about anytime soon either.
[00:47:58] Dara: Okay, the last one, some people think it’s the easiest, some people think it’s the hardest. What’s your favourite way to wind down outside of work?
[00:48:05] Weiting: I do a lot of yoga, so for me it’s probably quite a straightforward one. I’m going to not go to yoga today I’m actually going to go swimming today in the Brockwell Lido. I’ve never been there before and it’ll be cold, so looking forward to that.
[00:48:20] Daniel: Thank you so much Weiting. It’s been a real pleasure chatting to you and thanks for giving us your time really to volley questions at you like this. I feel like I’ve learned an awful lot, so thank you for humouring us and for telling us about your experiences. I think it’s been really valuable.
[00:48:33] Bhav: Yeah, thanks for joining us. I think it’s been fun to catch up again. Hopefully we’ll see you at the next CRAP Talks, but until then, like, you know, this has been fun to talk about product and thinking about data as a product, I think it’s a, it’s a fairly new concept so hopefully our listeners found it useful and we’ll start to think about potentially adopting certain aspects of it into their own working lives.
Daniel Perry-Reed
Subscribe to our newsletter:
Further reading
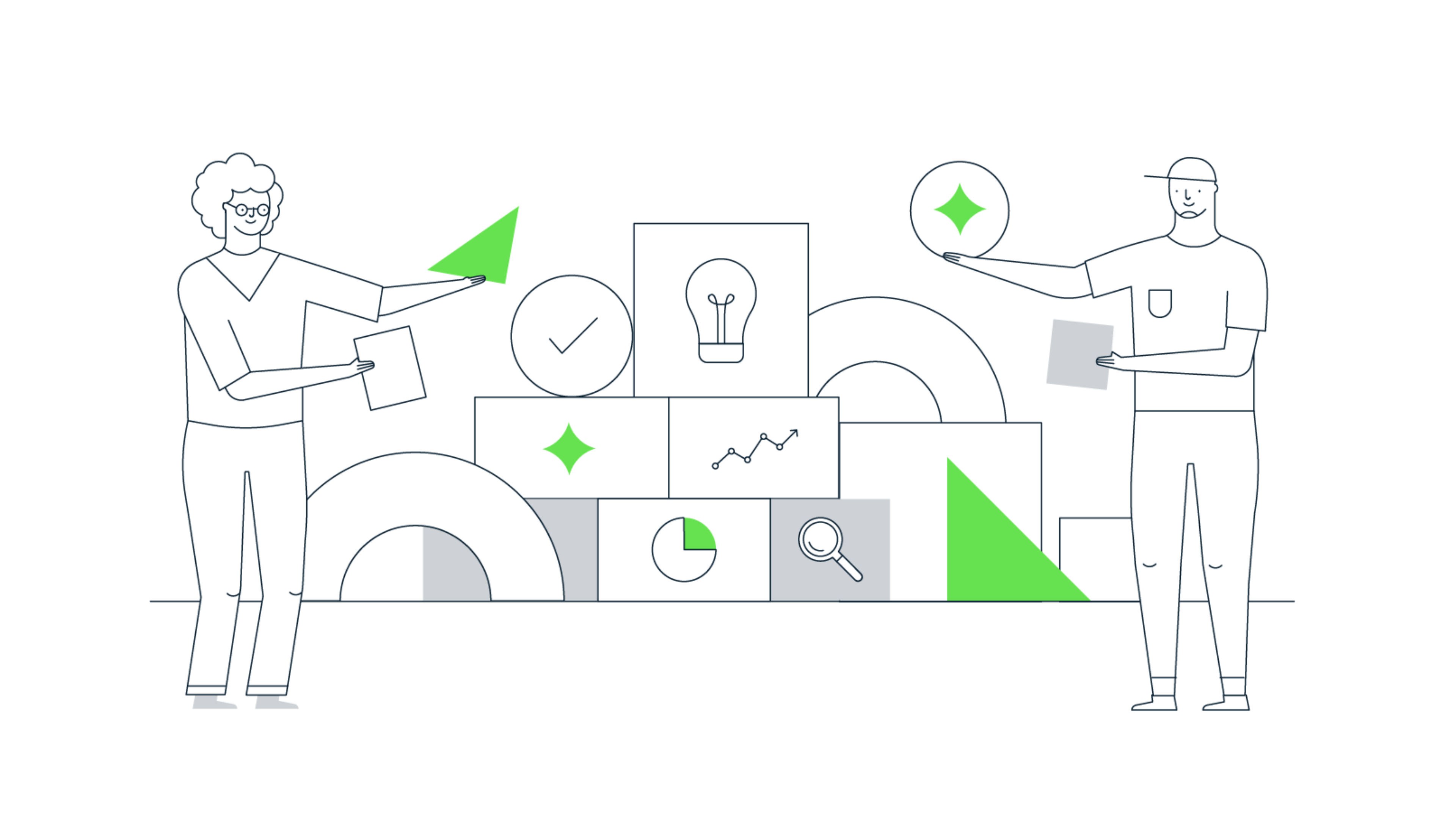
Easy ways to prepare your BigQuery warehouse for AI
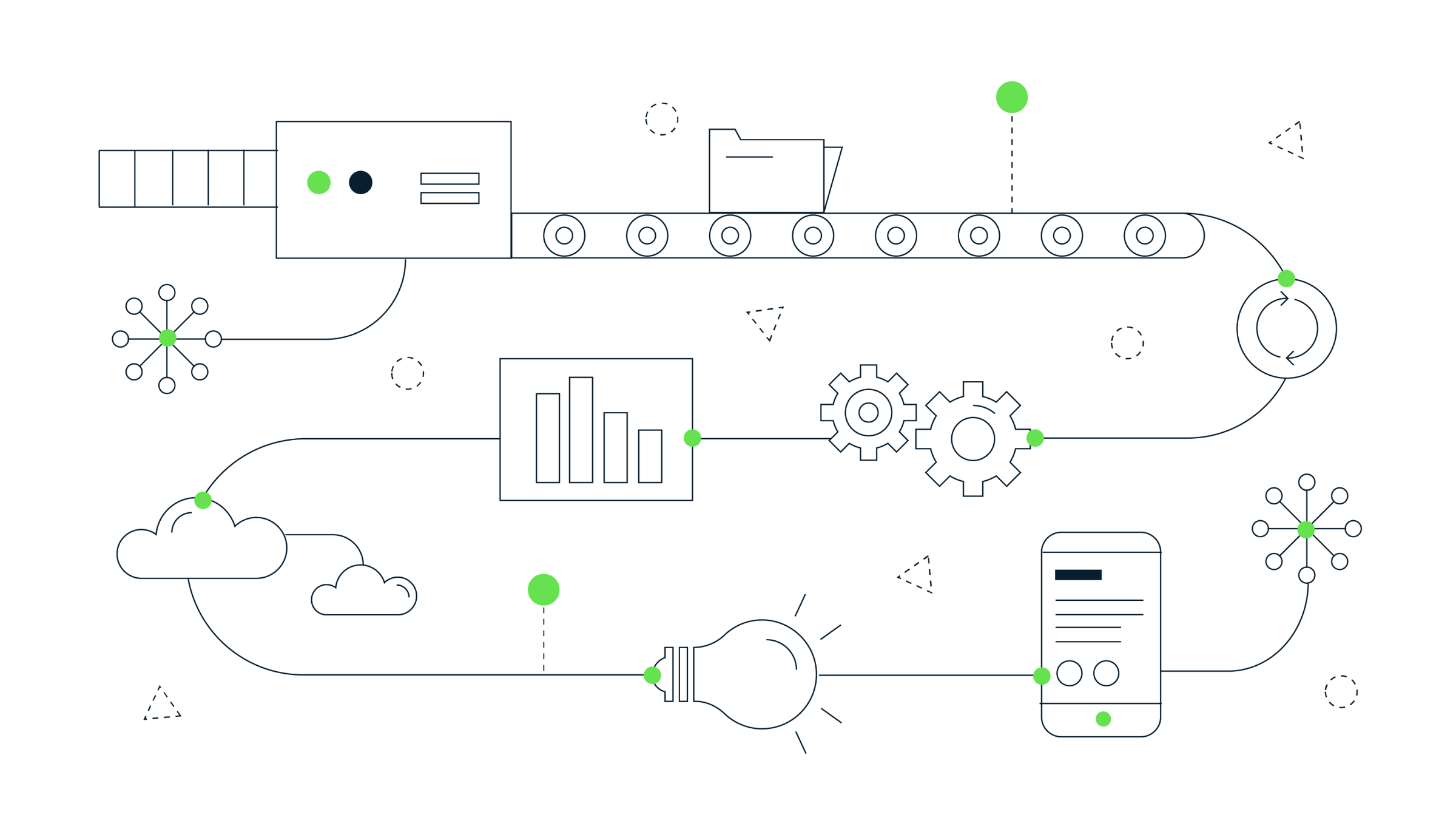
Data pipeline optimisation with Google Cloud and Dataform
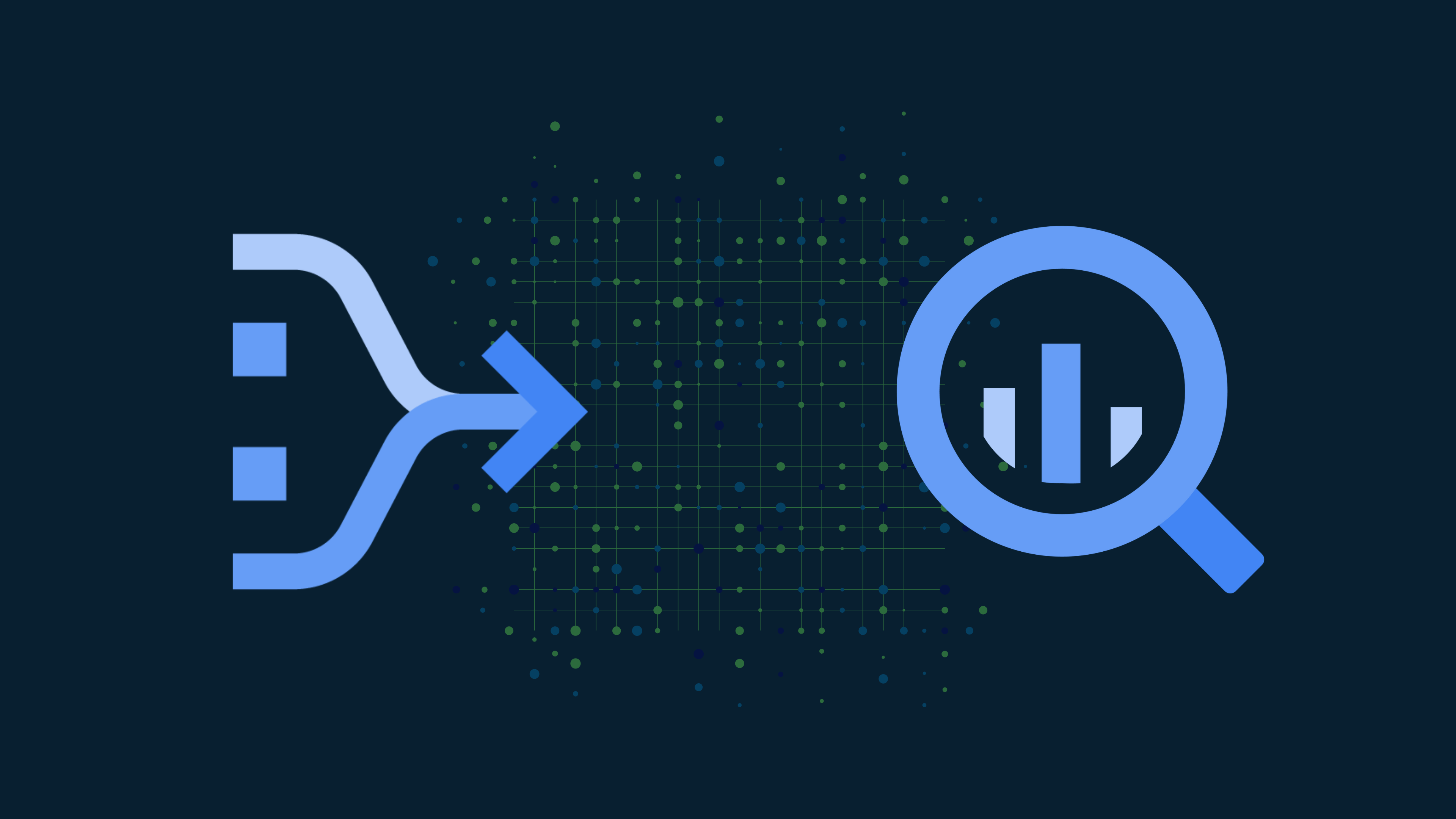