#20 Why you should link GA4 to BigQuery today (with Lace-Chantelle Rogers)
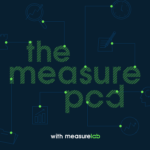
This week Dan and Dara are joined by Lace-Chantelle Rogers to discuss why it’s important to link GA4 to BigQuery as soon as possible. They cover the various ways that BigQuery is an amazing tool to take your analytics to the next level, and all of the things you’d want to do in BigQuery depend on having a set of historic data to query.
The full blog from Lace on this subject is here.
Get started and link GA4 to BigQuery by following this guide https://bit.ly/31Iqu2B.
The new beta Amazon Redshift Google Data Studio connecter can be used directly in GDS, but the details are at https://bit.ly/3GMnNfR.
The Google Cloud Pricing Calculator Lace mentions is at https://bit.ly/3yb0qcs.
In other news, Dan plays more games, Dara is allowed out and Lace gets festive!
Please leave a rating and review in the places one leaves ratings and reviews. If you want to join Dan and Dara on the podcast and talk about something in the analytics industry you have an opinion about (or just want to suggest a topic for them to chit-chat about), email podcast@measurelab.co.uk or find them on LinkedIn and drop them a message.
Transcript
[00:00:17] Dara: Hello, and thanks for joining us in The Measure Pod. A podcast for analytics enthusiasts where each week we dive into an analytics topic, or we discuss a challenge that we’ve faced, or we debate an opinion, and we try and have a little bit of fun along the way. I’m Dara, I’m MD at Measurelab. I’m joined as always by Dan, a Lead Analytics Consultant also at Measurelab. Hey Dan, what’s new this week in the world of analytics?
[00:00:41] Dan: Hey Dara. One thing to talk about this week, Data Studio has released a Amazon Redshift connector that’s now available in beta. I’ve not been able to play with this just yet, it’s just been released. But what I think is quite exciting is that they’re actually creating, supported, fully supported connectors to non-Google products. So hopefully this is a sign of things to come and we’ll get a plethora of other supported connectors in the future. One thing to know with this is that, while it’s in beta at least, who knows what it will be when it’s out of beta, is you can only query your maximum of 150,000 rows using that connector. So it might be a blocker for you, it might make it unusable, but it might also be absolutely fine. Hopefully when it comes out beta we’ll have a bit more info around what it does and doesn’t do.
[00:01:22] Dara: Okay, thanks Dan. So this week we are joined by a guest Lace Rogers. She heads up the Advanced Analytics team here at Measurelab, so another Measurelabber. It’s really great to have you on the show Lace. What we’re doing and this is the first time we’re going to try this, is we’re going to ask guests how they actually got into the analytics industry. Cause it seems to be a bit of a theme that people stumble, fall, veer into analytics rather than necessarily setting out to go into that in the first place. So what was your journey like into the wonderful and weird world of data analytics?
[00:01:59] Lace: Well, I definitely stumbled into it as I studied Gemology which is jewellery. I moved down to Brighton and got the job at Lloyd’s bank, which luckily enough, they allow me to start playing with code and SQL and it just came pa ion from there. And then I took another risk of moving into mobile gaming when it was a very new industry. And there’s not much things around SQL or digital analytics that point, gradually it’s just developed from there. And then two years ago, I took the jump over to consultancy with Measurelab and haven’t really looked back.
[00:02:30] Dara: You moved to the dark side, you mean?
[00:02:32] Lace: Yeah, definitely dark side.
[00:02:35] Dara: Well, we’re very glad to have you both at Measurelab and on the podcast. And obviously the fact that you’re here, means that you’re bringing a topic to discuss. So what is it we’re going to be talking about today?
[00:02:46] Lace: Okay, so we’re going to be talking about a recent blog, which is why you need to connect your GA4 account to BigQuery today.
[00:02:54] Dara: Excellent. So I’m going to say we’ve teed this up nicely in some of our previous episodes, because a few times we’ve hinted at the extra benefits you can get. Dan loves talking about GA4, believe it or not, that might come as a shock to you or to our listeners. But he does like talking about GA4 and we have on several occasions mentioned that obviously you can get a lot more value out of that data if you do connect it to BigQuery. So, I think this is going to be an interesting topic to explore.
[00:03:21] Lace: So the first area is really the fact that you can store your data forever. The GA4 dataset has a daily file and that is stored infinitely every day that you have a connected. And I know from GA4 perspective, there is a limitation in terms of what GA4 will store. Whereas what’s stored in BigQuery does not get removed unless you proactively delete it.
[00:03:46] Dan: Yeah, GA4 has a data retention setting which is actually the exact same as Universal’s always had. But the only difference now is that the option of do not automatically expire has been removed. So there is a lifetime for the event level data within GA4. And it defaults to, I believe two months. The aggregate data, it will never be deleted. So daily sessions and conversions and revenue will never be removed from GA4, you’re always going to have that. What you won’t have access to is the raw event level data. So if you’re building audiences or segments or using the explore workspace, that’s where you’re going to come a bit of a miss where you won’t be able to query and reprocess that data. So I think this is a really fundamental part, and I think this is where something you said Lace is really important because it’s about getting it ready today because it doesn’t backdate. If you were to get GA4 set up today and then let it run for three months and then connect a BigQuery, you’re not going to get that historical data in BigQuery. So even if you have no intentions of querying that data right now, it’s almost imperative to get that connected right now so that when you come to it, you’ll have that data available to you.
[00:04:49] Dara: Sorry, just to chime in on that quickly, for anyone who’s upgraded to GA360, they’ll probably have in their heads that you get this historical 13 months backfill. So you might be forgiven for mistakenly thinking that you’re going to get that if you switch to GA4, but you don’t. And that’s the point you’re making isn’t it, you’ve got to start collecting from now.
[00:05:10] Lace: Even when you’re in development mode of your app or websites, using the data you can still get a wealth of information and it helps you track your development over time as well. A lot of people won’t actually implement their GA4 BigQuery feed until they’ve got their GA4 custom events in place. Whereas actually the power of the staging side of things, even if it is just in dev mode is actually, you can test to see if things are actually working via BigQuery as well. So there’s a huge amount of information that we used that allows us to actually make sure that the app or websites worked well and properly.
[00:05:47] Dan: So basically saying is that we don’t actually have to wait until you’ve got good clean, proper data, maybe from the start of a month or start of a calendar week or a financial year or whatever you’re working towards. It is actually just connect it from when you set it up with those enhanced measurement events. Because while the apps and websites are in development, it gives you a lot of information. Even if you’re debugging t hose apps and websites. Cause you can do it through the DebugView, you can do it through the console if it’s on a website and there’s the equivalent for apps as well, but actually another way of debugging that is going to be through the BigQuery feed.
[00:06:18] Lace: The other advantage about getting BigQuery implemented earlier is your analysts who are building reports of the back of BigQuery will be able to actually structure their code in anticipation of the launch of your website or app. Because the actual structure of the table will not change, even if you move into a new project.
[00:06:35] Dan: That’s a really good point.
[00:06:36] Lace: Makes life a little easier. So the next point I wanted to discuss is the fact that you can actually clean and fix data. Um, the advantages you can effectively retrospectively apply conversions to your data without having implemented them on GA4. And it allows you to track over a longer period of time. So there’s lots of cleaning opportunities there.
[00:07:00] Dara: And that in itself answers the question though we’ve been asked so many times over the years, which is when you fix something traditionally in Google Analytics, the first question you get asked is can that fix be applied retroactively. And the answer is always the worst answer to have to give when you say no, it’s only going to work from here on out. But what you’re talking about Lace is obviously if you have the capabilities to get that data into BigQuery and work with it in BigQuery, you have effectively full control over it. You can clean it up, you can rename things. And then like you rightly said, you can also apply your own logic for conversions and you can apply them all historically as well.
[00:07:39] Dan: Yeah, the classic in Universal is getting that bot or spam view filters in. And as soon as you’ve done it, generally speaking, you would have done it as a reaction to seeing it in the data. And you’ve always got this bump in the data that you just have to put annotations on, just so that you’re aware that something happened that you did fix it. I think this is really important that you can go back and apply a sweeping change across all the data set based on whatever you want. You can rename stuff. I mean, the classic UTMs are going to be a really important one there, the amount of times we’ve seen all sorts of variations of spellings and upper cases and lower cases in the UTMs.
[00:08:12] Lace: Yeah. And there’s is even elements such as actually retrospectively clean PII data as well. What it means is you can actually still use your data and be compliant if you have incorrectly been sending personal identifiable information as well. And as you mentioned Dan, editing names of sources, mediums really do support effective analysis. And you can then regroup and reclassify very easily based on them, or even the events if you make an error in a parameter name or an event name, you can also clean them retrospectively. So it’s really at your power of writing some SQL and overwriting tables.
[00:08:51] Dara: I guess I’m spotting the risk there that it means people will not have a good naming convention in the first place. It’s still, it’s still good to try and do it right at the source, isn’t it. But I guess the point here is you have that control after the fact to make changes if need be. And another interesting point that I picked up on there from what you said, Lace. So going back to the fact that you can retrospectively assign conversions, but you can do as many as you want. So you can also through BigQuery you can get around a lot of the limitations that you have in the UI with GA4, and this was the same with Universal as well. There’s a lot of limitations within the interface that can be quite frustrating when you’re trying to do some kind of comprehensive analysis. But again, you can get around a lot of that in BigQuery. You can assign as many conversions as you want. You can add as manny additional fields as you want, you pretty much have control to manipulate that data as much as you want to without being restricted by the limitations of the UI.
[00:09:48] Lace: If you think of it, you can pretty much do it with SQL, that’s really the crux of it. You have full control of your data.
[00:09:54] Dan: And something that came up recently on the podcast is actually custom channel groupings, right. So GA4, hasn’t released the feature of creating custom channel groupings, they’ve recently updated the defaults, but you still can’t create your own. So this will be a really good way to maybe even work around some of those limitations or maybe some of those features that have yet to have parity with Universal Analytics. It’s actually integrating features that we maybe were used to within Universal, but don’t have access to right now in GA4.
[00:10:23] Lace: Okay, so next reason is data joining. There is a huge opportunity with the GA4 data to actually join it to multiple other sources, including Google Ads. But additionally, it could even be your CRM data where you’re trying to show the user journey from start to finish.
[00:10:42] Dara: I’m going to sound like such a dinosaur here, but I used to really frustrate me when people would always try and shoe horn data back into GA, rather than actually having a way of getting the GA data out and then correctly, and kind of usefully merged with other data like CRM data, which sits much more naturally in something like BigQuery. In the olden days, people we would always try and shove data that didn’t really fit with the data model. It would skew a load of metrics as well, because it would be like forcing it in as sessions or something like that. It never quite felt like it worked well. It always felt like it would make much more sense to do it the other way around and get the GA data out into a data warehouse and then blend with those other data sources that you mentioned.
[00:11:26] Lace: Yeah. And I think the great thing about the fact that it stores data forever, you can retrospectively applied this external data and create your own reporting tables based off these external sources. So using the BigQuery interface and warehouse functionality, you can have ultimate control and also be able to them visualize that in tools like Data Studio and Looker.
[00:11:47] Dan: This, this is fascinating, I love thinking and talking about this. But I think there’s two threads here, right. There’s aggregated data, which most data is becoming, or is already. And is very much a reporting exercise. If I wanted to incorporate my marketing spend data into a report, I’d have to blend my performance data and my marketing spend data. And the join key might be date, it might even be campaign or ad group or copy or whatever that is. But what we’re doing is we’re taking aggregated data and we’re joining it with aggregated data, finding different again in my head is not SQL, it’s very Google Sheets or Excel based, it’s an amazing VLOOKUP, right. Based on a couple of different parameters. But something else you said that Lace is really fascinating, which is that getting into the data like CRM and other user-based or user level data that we can join in a single customer view as such. So there’s two sides. There’s a reporting aspect, which is I want to get cost by campaign by day. The other side is going to be, I want to join this data with some other identifiable user data that you can integrate into the user journey so that you can probably then go back in time and rerun attribution models or reports or things like that. But I find that difference between the two fascinating. Both are data joining, both 100% sit within something like BigQuery, for sure. But both very different approaches and very different outcomes.
[00:13:09] Lace: Yeah, so the user journey reporting when you go through a individual level, you can completely reattribute and review exactly where your income and your ROI is coming from. Additionally, the aggregation side of things is key to really understand how you’re performing, but also understand where things are going wrong and why they’re going wrong.
[00:13:31] Dan: I think it really depends on that type of analysis you do, as you say Lace. It’s that, um, you know, attribution modelling that multichannel attribution, single customer view, I can draw a line in time for a customer and see all of their interactions. And then there’s also the other side, which is going to be more econometric or media mix modelling, which is going to be aggregated data over time. Looking at really clever ways of identifying correlation and trends. And there’s going to be like that trend analysis and that user analysis and that those two more so than ever are having to play nicely together. Because the access to that user level data is diminishing, but we’re still going to get the aggregated data. So actually what we might have had maybe five years ago is everything was based on that user level data, multichannel attribution, and the kind of econometric side or the media mix modelling side would have been kind of secondary. It’s almost like the scales are shifting slightly so that we have to take them both on equal pegging. And I can’t see that changing anytime soon. We’re never going to go back to a world where we track everything from everyone without any restrictions. I think is only ever going to become more strict, so it’s actually something we’re adapting to now. And it’s all about the models that we’re using, right. It’s about what analysis, what models can we apply on this data based on what type of data it is.
[00:14:46] Lace: Yeah. And this is a great segue into the next point, which is data modelling. There is pretty much infinite list of models that you can apply to data. Some of it aggregated, some of it user journeys. And you can actually model the data on multiple levels. You can model in BigQuery, you can also export it out to your tool of choice. So it could be SAS, Python or R, or it could even be a tool like Looker or Power BI to help you predict your performance and see your trends. And the great thing is, is the ease of usage between the systems and how they talk to each other. Due to GCP’s API that they’ve set up to allow us very quick BigQuery access.
[00:15:27] Dan: This is probably the most important point, at least from my perspective, is why we should be looking at this today. Because without historical data, doing things like predictive modelling is almost impossible because you’re just guessing you may as well have a random number generator in a spreadsheet right. Going back to one of the earlier points, it was you need to look at setting up the GA4 to BigQuery connector as soon as possible, even as soon as you’ve got that Enhanced Measurement events tracking implemented. Then when you come to do this kind of stuff, even if it’s not on your radar right now, you’ll have that historical data to be able to do that modelling. A lot of people might think about connecting BigQuery when they have clean data, when they want to do data modelling, when they want to join data with other datasets. But actually what we’re saying is we get that, that is their natural progression, and today we should be thinking about this so that when you get there, you’re not going to be like, oh, I’ll turn this on and start from scratch and wait three months before I can start doing this again. So that’s a really good point, I think the modelling for me is the most important point on this whole thing. And one of the biggest reasons why I recommend to everyone to turn that on as soon as possible.
[00:16:28] Lace: Yeah, it’s imperative for significance in terms of volume of data . If we don’t have that, we’re not able to build the best models. The more accurate model is usually over a time period or over a big volume, and that will allow you to make decisions based on these models. Without having a data come through today, you will have to wait a significant period. And having this data today allows you to come quite data led organization rather than gut instinct. It allows you to dig into the data, to identify things that humans wouldn’t be able to identify, using clustering and tools such as that.
[00:17:03] Dara: And there’s no real, flipping this topic on its head completely, there is no real reason not to do it today is there. Because all of the more sophisticated analysis and models that you can run, so things you mentioned Lace, like using BigQuery machine learning or using R or Python or whatever with the data, you don’t pay for that if you don’t use it, essentially. If you set this up today, you pay for your storage, you’ll pay for any queries you run, but it’s not like you’re paying for a full feature set that you’re not going to use today. There’s not really any drawback to doing this is there?
[00:17:42] Lace: No, and the great thing about GCP is it has a scaling price which allows it to grow with your business. So initially you do get a free allowance which is 10 gigabytes per month storage, and you also get 1 terabyte of data querying. But in general, actually the cost is very low and you’re able to calculate and see in advance how much your data warehouse or your code process is going to cost by the GCP calculator as well so you can prep for cost increase. You will spend very little on your data storage until you start to utilize that data. And even then, if your queries are efficient, you tend to actually not generate a huge amount of cost.
[00:18:22] Dan: So playing devil’s advocate, that is a reason. Or at least the reason I’ve heard the most of why people aren’t doing this right now. And that’s because it is new. So someone coming from Universal Analytics, they’ve never had to think about paying for anything to do with the analytics. It has been a free product to them. Obviously we know that’s not necessarily true. It’s a free tier of an enterprise level solution. But what we’re saying now is even on the free tier of Google Analytics on GA4, what we’re suggesting and what a good best practice, or at least our advice would be, is to connect a BigQuery as soon as possible, which does inherently come with a cost. And it doesn’t matter how much or how little that cost is and how scalable that is. That’s always going to be the blocker, I think, to doing this because it’s an unknown. Especially people that don’t have GCP experience or don’t have a GCP accounts to begin with. So they don’t, everything’s new to them. It’s like, well, what is the GCP? What is BigQuery? What is this billing account? What am I putting my credit card against? How do I justify that in my budgeting for the next financial year? I can imagine this will change 2, 3, 4, 5 years down the line, it would be inconsequential. But I think right now there’s a transitional period where people are thinking, why, why would I need to do this? And why is this important? Obviously that’s the conversation we’re having now, but again, playing devil’s advocate, that is the reason why people probably wouldn’t because, not only is it a variable billing, it’s something they’ve never had to pay for before.
[00:19:46] Lace: Yeah, and I think the key thing is keeping track of your billing. GCP actually provides lots of functionality to allow you to be in total control of the billing including setting limits, quotas to allow you not to have any nasty surprises. They have a great interface as well to track the billing. But the main thing is having to look at the GCP calculator when you start to have a look at the cost. In general, you can control these costs quite a lot though, even at a query or personal level. Just tracking and reporting on billing. And that allows you to really identify, if you do suddenly see a boost in spend, you can very quickly resolve it.
[00:20:20] Dara: That’s the key point, isn’t it. Cause to put it into context, even the allowance you mentioned, so you get the 10 gig of storage and a terabyte of querying costs every month. For quite a number of websites, they wouldn’t breach that limit, would they.
[00:20:33] Lace: No. And in general, even when I’ve streamed 15 million rows per day, which is a huge amount, we were spending about $10 a day and that was extensive querying on different systems as well. So it is incredibly hard to spend a lot of money. And the biggest thing is just making sure that your analysts understand there is a charge, and look at the dry runs and the system information that’s provided to them when they’re writing their code as well.
[00:21:01] Dara: Yeah, and I think it is a misconception isn’t it, I think sometimes people think something that sounds advanced, sounds complicated, sounds vast, sounds enterprise level, they automatically think there’s lots of dollar signs. So that it is a bit of a misconception, isn’t it. And it is it’s really worth stressing this, that for the vast majority of users, this isn’t going to cost them a lot of money.
[00:21:23] Lace: Yeah, absolutely. And BigQuery has quite low price points, which is fantastic. And GCP are incredibly open with all the costs, there’s no hidden costs. They make you very aware with each of the systems.
[00:21:34] Dan: I love this conversation too, around how does Google provide something like Google Analytics, which, you know, there’s lots of numbers flying around the internet, but up to 60% of all the websites ever made have got Google Analytics on for free. How can they do that, how can they make money? And it’s not from Google Analytics. It’s from the peripheral tools like Google Ads and the integrations they have with them that enable you to track and spend more money there. But I think this is just the next generation, and saying that you can have your data collection done for free using something like Tag Manager, Google Analytics, and you can report that in something like Data Studio and even do AB testing and Google Optimize, even Firebase to some extent is free to use. But where you’re going to start paying, is for these privileges of having things like your data stored forever, or to be able to join your data, or to be able to apply your own models on it. I think this is the exciting future aspect, where it’s free but if you want to take it to that next step, then you start paying for it. And that’s variable based on how far you take it. I think it’s a really nice approach, because it scales with you. As you do more with that, as you get more value, as you activate more of your data, then you pay more for that. But hopefully, you know, the whole idea there is you get a return on that and you can keep justifying that investment.
[00:22:48] Lace: Yeah, it’s a great example, elastic pricing really, because it just scales as you grow. And also if you then found that you need scale back, it’s very easy to then scale about the price as well. So you are ultimately in control of your spend.
[00:23:02] Dan: You can always delete it, right. You can always just click delete, delete the data, not query at anymore and stop spending money. It’s not something that’s going to cost you from now on forever, even if you don’t want to. This is something that you can turn on, find out that it’s not for you two years down the line, and then just turn it off. There’s actually no risk in setting this up now.
[00:23:20] Lace: Absolutely. And the other great thing is that GCP has designed tools that allow you to also migrate your data to other platforms, should your company or business change structures as well. Ultimately you’re in control of where your data is stored, and how much you’re spending.
[00:23:36] Dara: My heart skipped a beat, went Dan said, you can always delete it. Was I the only one?
[00:23:42] Lace: Yeah, scary as any programmer here in data, been deleted. Archived, archived.
[00:23:49] Dan: just, don’t give everyone admin access. That’s the rule, right?
[00:23:52] Lace: Yeah. And GCP has so many levels of access and you have ultimate control who accesses, who programs, who can even generate tables. So you can stop people doing bad things. The other great thing, which is not mentioned on the blog, is the fact that if you do accidentally delete a table, you have three days to actually get that table back up and running. And if you accidentally overwrite a table of incorrect data, you’ve got five days. They’ve designed it so that you are in control as a project owner.
[00:24:23] Dara: That’s a bit mean, in GA you get 28 days to restore a deleted view. So you’ve gotta be quick to realize your mistake if you’re talking about deleting BigQuery tables.
[00:24:32] Lace: Yeah, most analysts will realize very quickly that they’ve done something. Usually when the ETL fails, it’s quite quick for people to notice. This is where you can even build in tools, which show you error detection or issues with your code. Because everything within even the GCP system provide you login to be able to see what’s going on. In fact, you can even see who programmed, what on your project.
[00:24:57] Dara: Uh-oh.
[00:24:57] Dan: I love to be able to point the finger.
[00:24:59] Lace: It’s a great tool the audit logs.
[00:25:03] Dara: Okay. I think that’s been a really good overview of the reasons and I I’m going to probably do quite a bad summary. I think that the standouts for me are the fact that you get around the limited data retention within GA4, so you get to store your data in BigQuery indefinitely. A huge amount more, if not complete control and flexibility over what you do with that data. So you can clean it, you can blend it with other data sources, you can rename things, you can change it to your heart’s content. And then there’s the obvious, extra next level advantages where you can do advanced modelling or advanced analysis using all the tools within the huge GCP ecosphere. And it’s not that expensive for most users. So if to, again to flip it round, what are the reasons not to do this today, well there aren’t really any at least no significant reasons. Our consensus here is that this is something if you’re using GA4, you should set up the connector with BigQuery and you should do it right now, as soon as you’ve heard this podcast. I think that’s the moral of this story. But enough about GA4 and BigQuery, this is the point in the show Lace where we switched gears and we talk about what we’ve been doing outside of work to wind down. So what have you been doing in the last, let’s say in the last week or so to wind down?
[00:26:21] Lace: Um, I’m not sure if it’s a wind down, but I’ve put 1500 fairy lights on a Christmas tree and throughout my house, which no longer needs lights.
[00:26:31] Dara: Sorry. I think I misheard, it sounded like you said 1500.
[00:26:34] Lace: Yeah, I got to be excited when I bought the lights and may have overestimated how many I needed for a tree.
[00:26:41] Dara: So your electricity bill and the talking of a BigQuery been cheap, it’s going to be a lot cheaper than your electricity bill.
[00:26:46] Lace: Oh, for sure. Definitely.
[00:26:48] Dara: What about you Dan, what have you been up to?
[00:26:52] Dan: So I was going to say the exact same thing, minus the 1500 fairy lights, but I did the Christmas decorations last weekend, got the tree in and decorate the house. Me and my wife loved to spend the time doing that every year. But actually as you’ve taken that Lace, what I’ll say is I recently got a new video game on Stadia and it’s a game that’s been out for quite some time that I’ve completed at least twice before uh, but Rayman Legends. And I’ve just been absolutely obsessed with playing Rayman again, and it never ever gets boring. So my, my wind down this week Dara is I played another video game, and it’s no Flimbo’s Quest though of course.
[00:27:28] Dara: No. I was thinking of Flimbo’s Quest.
[00:27:31] Dan: How about you Dara, what have you been doing mate?
[00:27:33] Dara: Well, I mentioned last time that I was struck down with the dreaded COVID, so I was in self isolation. Good news, congratulations to me, I’m now out of self isolation. And I’m going to, so this is, this hasn’t happened yet, but I’m going to Ireland this week. So I was due to go last week when I was in self isolation, so I postponed my trip. So I’m going to be going over there this weekend to see my family pre-Christmas. So I’m looking forward to that, and I will almost certainly have a few pints of Guinness. Okay, that’s a wrap for this week. As always, you can find out more about us measurelab.co.uk, or you can get in touch via email at podcast@measurelab.co.uk, or just look us up on LinkedIn. And please do get in touch if you have a topic or an opinion that you’d like to come on and either discuss or debate with us, we’re open to both. We’re actually going to take a break now for a couple of weeks. So this has been episode 20, we’re going to take a break for a couple of weeks and we’re going to be back in the new year with the next episode. So I’m going to wish everybody a very Merry Christmas in advance, and a happy new year. So join us then for some more analytics chit-chat, I’ve been Dara joined by Dan and by Lace. So it’s a bye from me.
[00:28:48] Dan: Bye from me.
[00:28:49] Lace: Bye from me.
[00:28:50] Dara: See you next time.
Daniel Perry-Reed
Subscribe to our newsletter:
Further reading
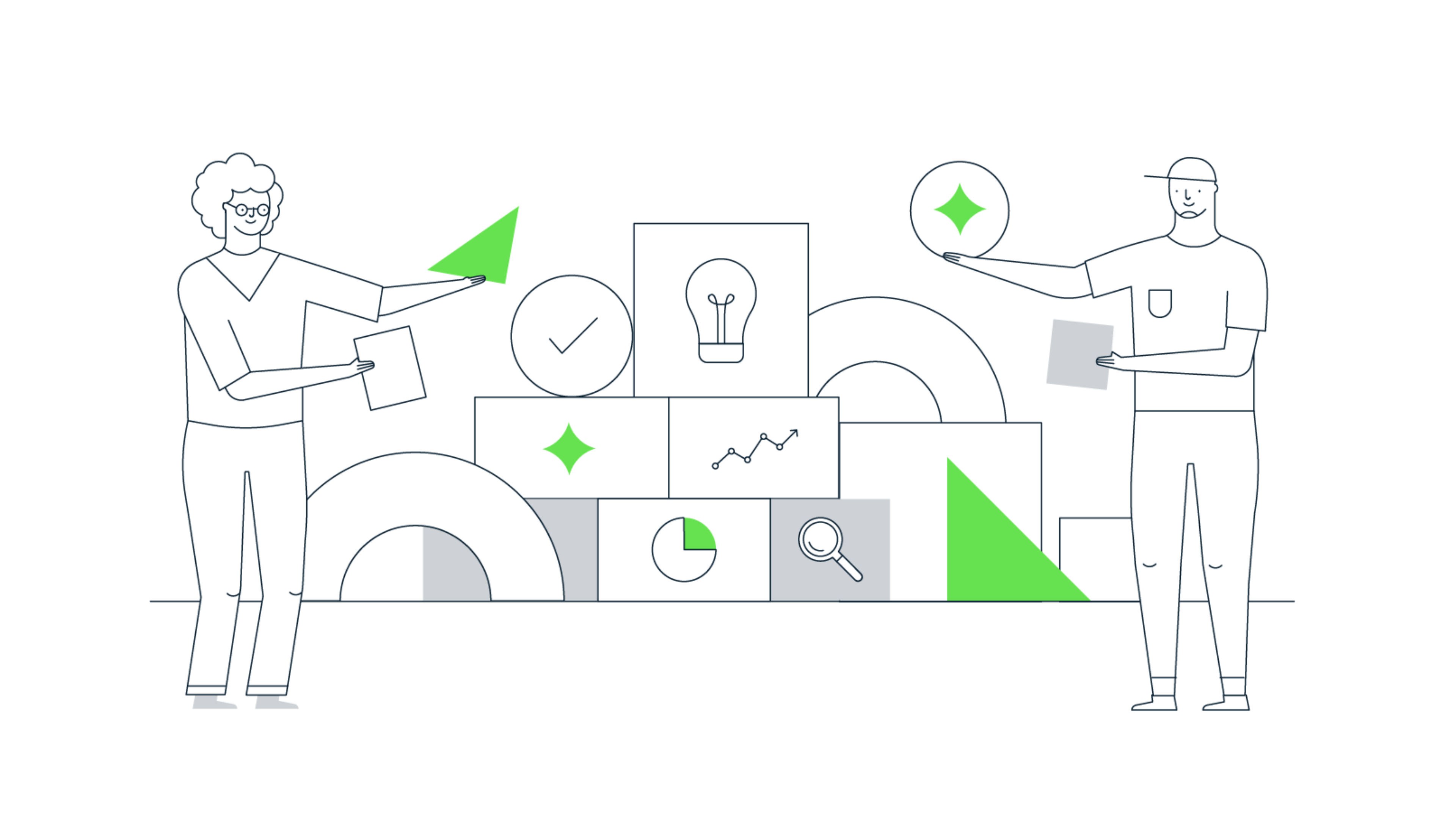
Easy ways to prepare your BigQuery warehouse for AI
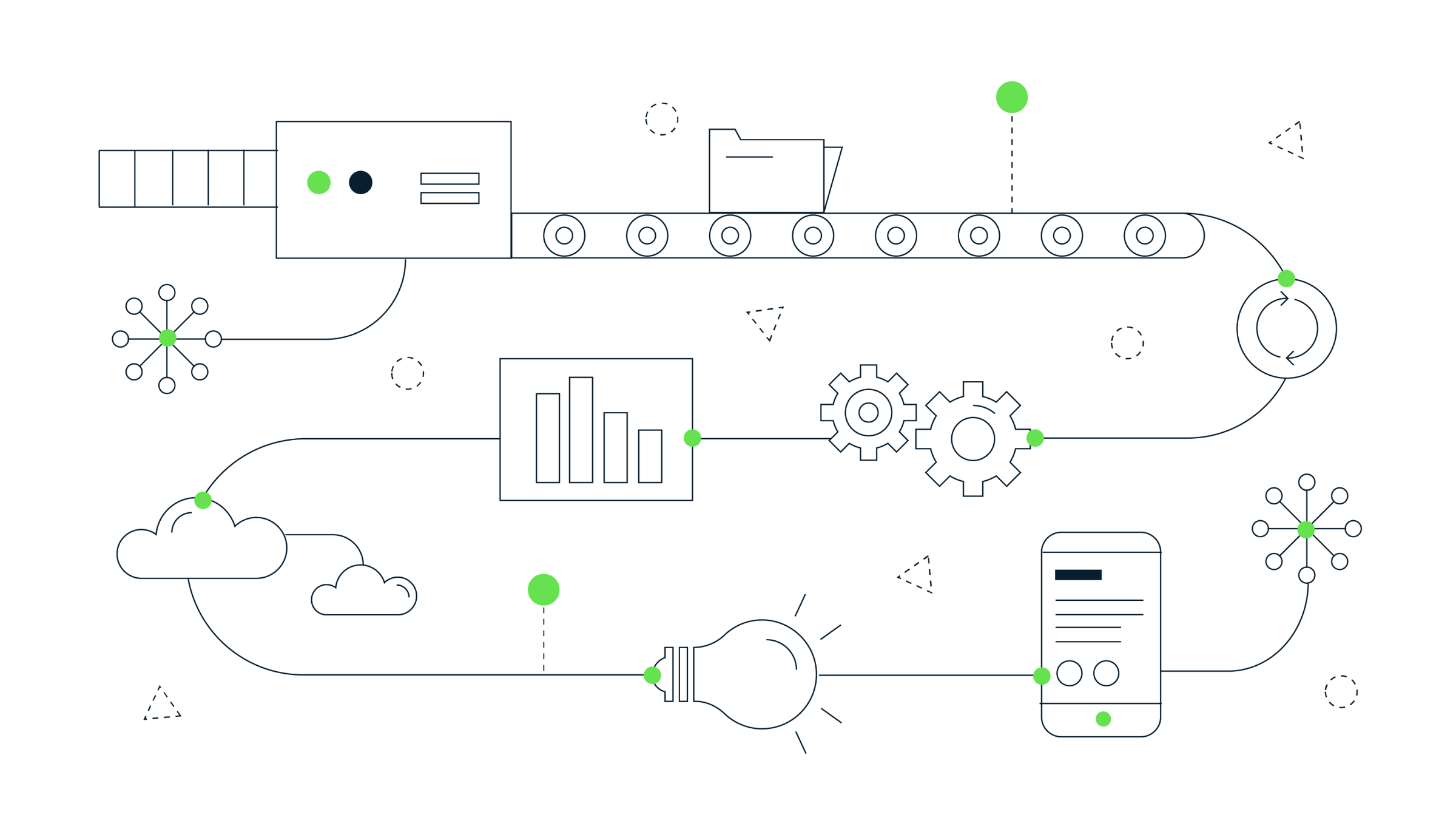
Data pipeline optimisation with Google Cloud and Dataform
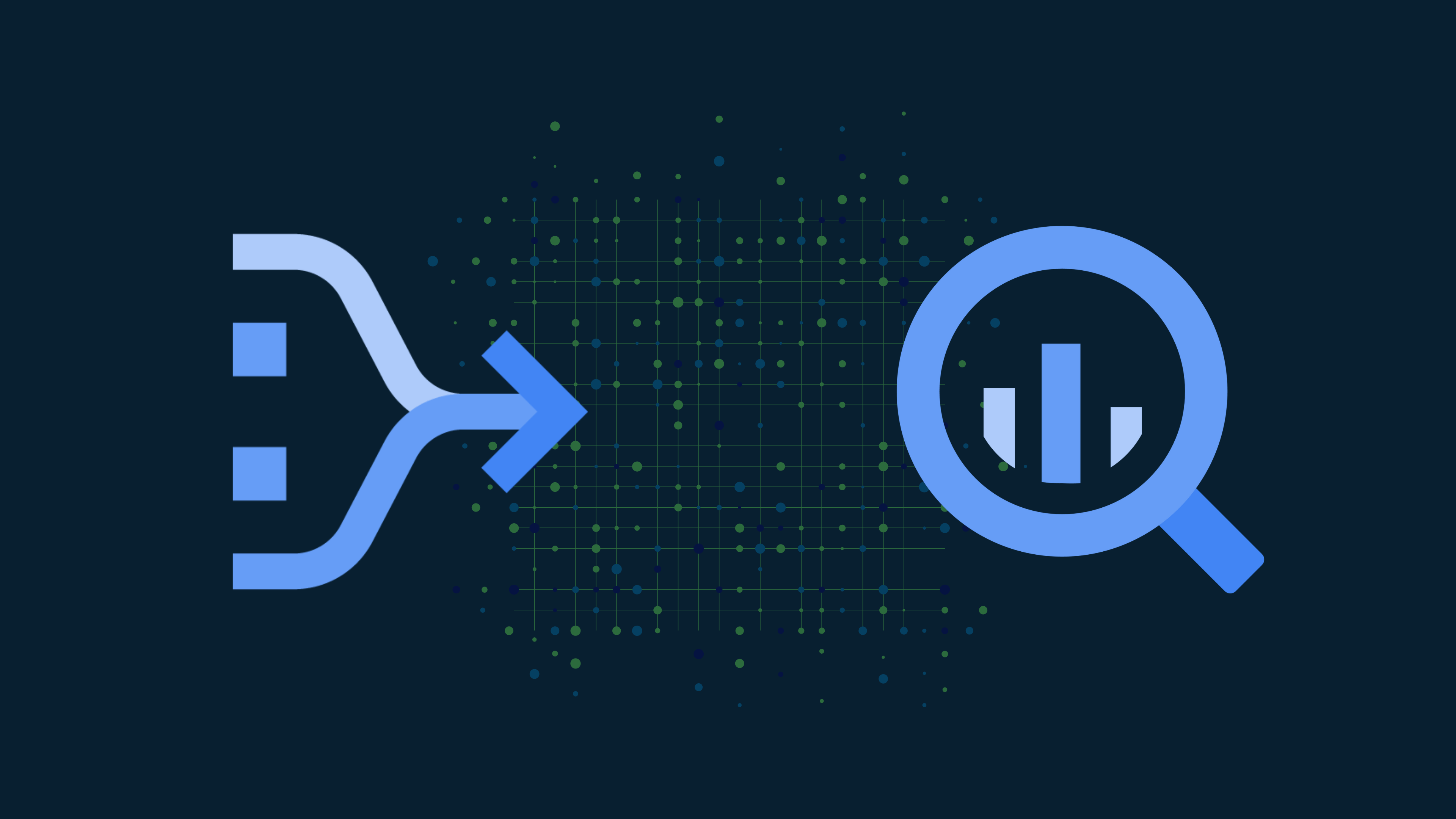